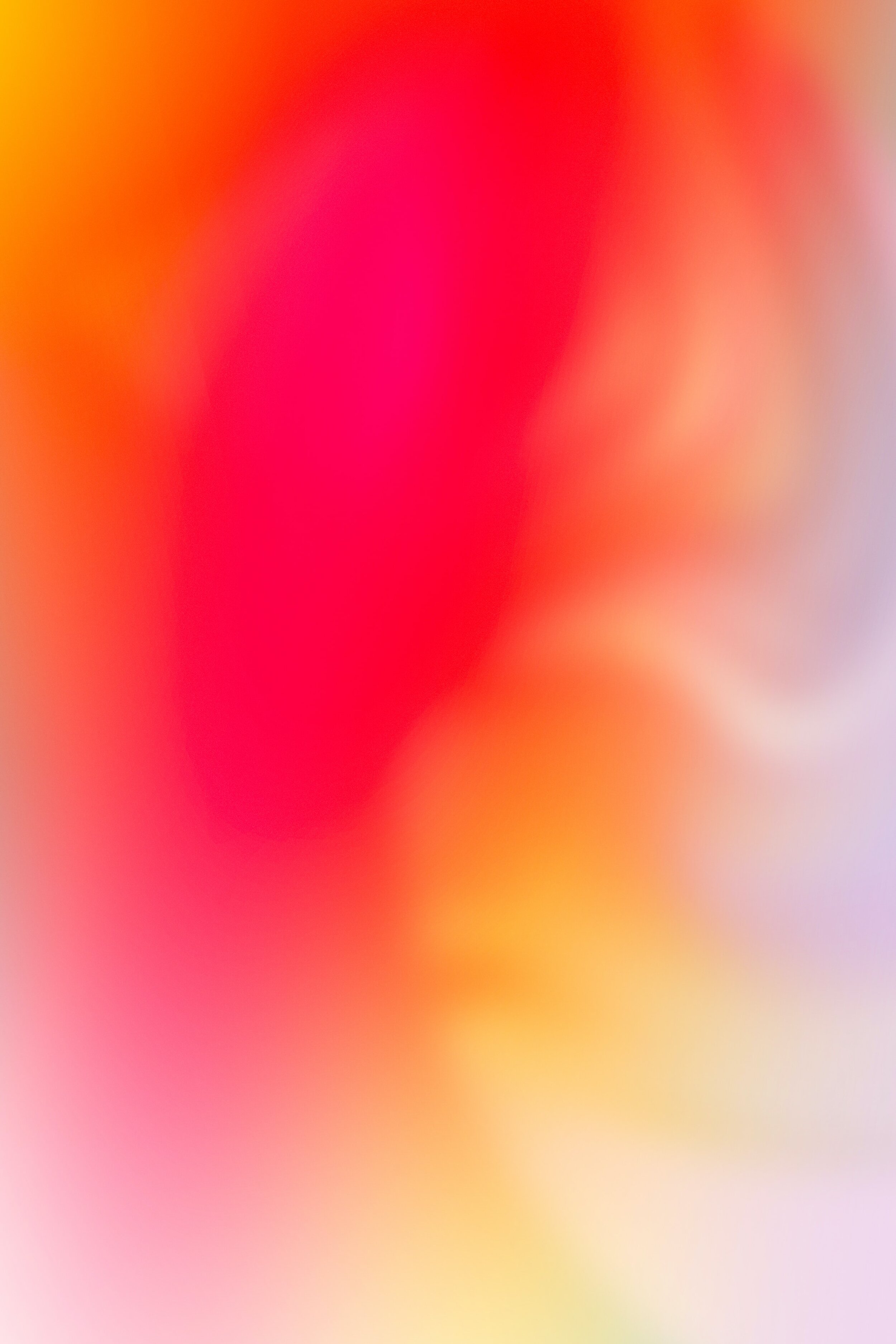
February 10 2023 • Episode 014
Lukas Vermeer - A Global Leader In Online Experimentation
“There is no such thing as the perfect experiment. The notion of a perfect experiment doesn’t exist. There is only a continuum between very well executed experiments, and completely winging it off the seat of your pants. The intent is not to make a few high-quality decisions and guess the rest, but to make all decisions a little bit better”.
Lukas Vermeer is widely acknowledged as a global leader in the field of online experimentation. He is an expert at establishing and scaling experimentation and is currently the Director of Experimentation at Vista.
Lukas has co-authored many influential academic papers on experimentation and is a highly sought-after conference speaker. He is an experimentation practitioner at heart, combining industry experience in A/B Testing and Data Science with a background in computing science and machine learning.
Previously, Lukas was Director of Experimentation at Booking.com, the world’s leading accommodation website. At Booking.com he was responsible for the tools, infrastructure, and training that helped product teams at Booking.com improve the customer experience through hundreds of thousands of experiments.
Lukas also works as a freelance consultant, helping businesses grow their experimentation culture.
Get the transcript
Episode 014 - Lukas Vermeer - A Global Leader in Online Experimentation
Gavin 00:03
Hello! And welcome to the Experimentation Masters Podcast. Today, I would like to welcome Lukas Vermeer to the show. Lukas is currently the director of Experimentation at Vista. He is widely acknowledged as a global leader in the field of Online Experimentation. Many of you will be familiar with Lukas's work as director of experimentation at booking.com, where improved customer experience through 100s of, 1000s of experiments. Lukas Vermeer has co-authored many influential academic papers on experimentation, also working as a freelance consultant to help businesses grow their experimentation culture. Welcome to the show, Lukas.
Lukas Vermeer 00:47
Hey, happy to be here.
Gavin 00:49
I feel that has massively understated your career and achievements. But you're pretty humble. So, let's just run with that.
Lukas Vermeer 00:59
I think you’re overstating it. I didn't write many academic papers just a bunch. As second or third or fourth authors. Don’t overstate it.
Gavin 01:10
Now, let's make a start with some introductory questions that one of the things, that I was interested in exploring further was going back a little bit to your childhood and understanding what it was like to grow up with academics as parents.
Lukas Vermeer 01:31
That’s a fun question, so yes, so both of my parents were Linguists, they were interested in language. My mother was focused on language acquisition by children, so, how do you teach kids, Dutch? I was born and raised in the Netherlands. So, how do you teach kids Dutch in primary school? And my father was focused mostly on second language acquisition. So, Immigrants wanting to learn Dutch as a second language, mostly when they were adults, which is an entirely different field, obviously. What was it like?
I don't actually think, I got a lot of the Academic background of what my parents were doing. Like, we didn't, it wasn't like, we talked about statistical models at the dinner table. I think what I did get from it was, that my parents had a lot of freedom in terms of working from home at the time already. So, both my parents were, I think… I mean, I was young, so I don't really recall. But I think, both of them were working four days and at least one of them from home. So, there was almost always a parent in the house. But at the same time, they were both working parents. So, it wasn't like, I had to stay at home mom or stay at home dad, I had two working parents that were home a lot.
So, I think that's what Academic freedom gives you, that you can work late. You can work late in the evening, but you can be home for your kids. But I'm not sure, I got a lot from it. Although, now, you mentioned it like, my first starts in Computer Science was actually through my dad because he was working at the University of Tilburg. And one of his colleagues was working on Language processing on computers. And I think, it was eight or nine years old, when he brought home a syllabus, that explained that how to program in Turbo Pascal.
And that was my first introduction to programming, it was a unit like an Academic syllabus that was intended for first year students. It was my first Intro. I think the first computer program, I ever wrote was actually constructing, I was eight right so humor is different. I think, it was constructing curse words, by taking random strings from a set of strings and then concatenating them. So, it would be like a first take an Adverb like dirty or ugly or nasty, and then concatenate a Noun, such as pig or pumpkin, and then press a button and it would generate a new curse word. And that was fascinating to me that you could use, you could make the computer create things that you didn't predict in the sense that there's almost like an emergent property coming out of that, out of the Software, which I found fun fast. And that I think later inspired me to study Computational Science and Artificial Intelligence and then experimentation flowed from that.
Gavin 04:39
That's one of the things that I was wondering that back in the day, those fields that you've now pursued, machine learning experimentation, data science were very much emergent at the time, so, I was wondering.
Lukas Vermeer 04:52
Logic again [Crosstalk 00:04:54] So, we've had the AI winter several times now. All right, so, machine learning was very popular in the 60s, 70s. But then it sort of died out when people realized it was actually very difficult to do, specifically language was very difficult to do, which is how I ended up in that field. And now we're seeing a resurgence on this. Now, machine learning is once again popular, because there's new technologies coming out that make new things possible that weren't possible before. And everyone's up in arms again, screaming how amazing AI is. And like, we've been here before, like, it's going to die out again, we're going to see the limits of this technology again, be disappointed again. And then it's going to be quiet for a few years, I suspect. And then there will be another re-emergence because AI has been having these waves where people say, Oh, I think the first like, a first winter came after some professor says, “Let's take us, let's take a summer to figure out how to do language generation with a computer. It should be easy, right? And then it took like 50 years for them to get that and be get to, where we are now.
Gavin 06:09
Interesting. So, thinking about some of those things that you're obsessing about right now. What are some of those things that are consuming you at the moment?
Lukas Vermeer 06:23
So, many things like my brain has so many different threads. So, my current role at Vista, I've a very simple task, really. And this is a company that runs experiments already but wants to run more. So, this is one of the Academic papers that I've contributed to, in the past, actually, which is the experimentation flywheel, which was spearheaded by Alexander Fabian. And in that paper, we sort of described this idea that if you want to scale experimentation, culture, really the only thing you need to do is, identify where the friction is, and then remove it. And that is pretty much it. And so that's what I'm trying to do. I'm trying to find a company where there is a will to experiment, but they don't really know how to scale out. And so my day-to-day, and the things I obsess up about is pretty much figuring out what is currently stopping Vista from running twice as many experiments as they are now like what is the bottleneck right now. And then sort of pulling that thread and seeing whether we can remove it or whether we can circumvent it, or you can do something to sort of, grease the wheel a little bit. So, that's what I'm obsessing about trying to figure out like, what is that thing?
Because there's always like, 1000 things I could be doing. All right, and I think I don't know if you've read “The Goal” by Goldratt, which is about Process Optimization. But, one of the key insights in that book is, that if you have a bottleneck in the Process, and you Optimize just before the bottleneck, you make things worse, not better. And that's because the work will just pile up, in front of the bottleneck. And this only makes the bottleneck more of a bottleneck. So, if you want to Optimize a Process, then you need to deliberately not invest in Optimizing before the bottleneck, but you need to invest that same time in figuring out what the bottleneck is and then removing it. Because that is the way, that you Optimize a Process. So, that's what I'm obsessing about now.
Gavin 08:23
I read a good one. Recently, it was the founding and scaling of Vans footwear, the streetwear label and the founder, he very much started in quality control Process Optimization, continuous improvement, which was an interesting sort of first 50% of the book. It did feel very much like, The Goal.
Lukas Vermeer 08:53
Yeah. I mean, have you read the Phoenix Project?
Gavin 08:57
No, I haven't read that one.
Lukas Vermeer 09:00
Now, it’s like yeah, the Phoenix first, right. So, a bunch of people re-wrote The Goal essentially, but for IT, including Jean Kim, who was very much into the DevOps movement. So, it's essentially the same idea as The Goal, except apply to I-T processes. And it's fascinating. I mean, I obviously with my experimentation lens, I see a lot of opportunities for experimentation in that story, right? There's never mentioned directly, but I see a lot of angles there. And to me, it's interesting that when you think about how you scale out experimentation in an organization that's partially sort of operational efficiency and removing bottlenecks, because a lot of this is about how do I as quickly as possible push ideas out the door, right and that is very much process optimization. But the other side of it is very much, how do you get people to even understand and appreciate that, this is something that they should be doing, which is a lot about change management.
So, this flywheel of experimentation, there's actually, it's not one thing, it's actually two components. One is the operational efficiency. And the other is sort of getting people on board and sort of bringing them along and sort of helping them understand, why they're doing what they're doing. And both that have to happen at the same time, right, they have to go hand in hand, because if the operational efficiency isn't there, then it's going to be a lot more difficult to convince people to move along. One of the books that really crystallized those ideas for me was, “Switch: How to Change Things When Change is Hard” by the brothers Heath. And then they talk about things like highlighting bright spots, right? So, rather than focusing on the places where it's not working, which is what operational efficiency is all about, right figure out, what the bottleneck is, when you want to change sort of organizational mindset, you actually need to focus on the things that are working and highlight them, and show them to people and say, “Okay, this team is running experiments, and they're having a lot of success doing it.” And here's how they did it. So, you sort of have to have this split brain almost where on the operational side, you're trying to identify bottlenecks. And then on the organizational side, you're trying to identify bright spots.
Gavin 11:33
Yes, I've had that book previously recommended by another podcast guest, Ruben de Boer
Lukas Vermeer 11:39
It's good. It's a good book
Gavin 11:40
Yes. How about thinking outside of work directly. What else are you obsessing about?
Lukas Vermeer 11:47
Oh, boy. Well, I have three young kids. So, my life outside of work is mostly kids. What am I obsessing about? How do I make those into good human beings? So, that's a momentous task that's worthwhile. It's difficult though, because I've never done it before. And there’s not a lot of good literature on this topic. To be honest, it's surprising like given, how long people have been raising kids, it's difficult to find good stuff on how to raise kids.
Gavin 12:17
There's no playbook. Is there?
Lukas Vermeer 12:18
There's no playbook. This is mostly about mindset, not your own attitude, I think. The other thing I mean, I've been obsessing about plants in my office for a long time. But now, you can see behind me, if you've got the video on. But we've been branching into aquariums because I realized, I had all of these different plants like plants on the floor, or plants in the ceiling, plants on the walls, as you know. You know where else plants live? They live under water. So, I decided, I was going to do an aquarium. So, I've been obsessing about sort of optimizing the quick, I have a bigger one downstairs. Now my wife was saying, she wants a salt-water aquarium. That's just another level. Like we've got a sweet-water now, which is fun with a bunch of shrimp.
So, yes, I try to find like, things to sort of tinker and toy with. But I'm not someone who will completely obsess about one thing for 10 years, like I get bored very easily. And so, I obsess about something for at most a year. And I was like yes, done. Next, move on. So, I was very much into home electrical wiring of my house last year, like I re-wired a bunch of rooms just because I wanted to know how to do it. Like I've never done it before. Then by the time, I knew how to do it, I'm like okay, now I know how to do it. Next.
Gavin 13:51
It’s more that once you feel that you've exhausted the learning opportunity from the immersion in that area, you move on to the next opportunity?
Lukas Vermeer 14:02
Have you seen my GitHub like that's just the… This was a graveyard of half finished projects. Be aware. The project ends as soon as I know how to finish it, like that, not when it's finished. But when I know, when I can see the end. That's when I give up, I do not give up but sort of move on and say Okay, so now I've exhausted everything, I can learn from this, I know how it ends now. Move on.
Gavin 14:25
So, with books do you read to learn rather than read to finish.
Lukas Vermeer 14:30
Oh, no. Oh, that's okay. So, this is weird. No, that's exactly the opposite. So, with books my OCD kicks in and I absolutely have to finish it, even if I absolutely hate it.
Gavin 14:41
Really?
Lukas Vermeer 14:42
Yes, I will plow through and read a book that I absolutely hate. So, that at the end of it with full conviction, I can rate it one star on Goodreads and say, I absolutely freaking hate it because if I don't finish it, I don't feel qualified to sort of give this a rating because I looked through, maybe it gets better. All right, so yes, now with books, I definitely have to finish it. So, books have a more rounded finish, it feels like a lot of these projects that I have on GitHub. They're like never ending projects, they can always… You can always iterate and improve and go on and do more. Whereas, with a book, like once you finished a book, it's over. Right? There is no iteration to have this book. Some books, yes.
But if you're. That's why I never started a series. Because like, I did make the mistake, once of starting “The Name of the Wind.” I believe that book is called, where it's a three part series, where the third book at the time when I was reading, it wasn't written yet. So, I read the first book, loved it. Read the second book, loved it. And then, wanted to read the third book and it didn't exist yet. That was the most frustrating experience ever. I want to read this now.
Gavin 16:00
Instant gratification. Let's switch focus a bit. And talk a moment about booking.com. One of the things that I'm sure the audience will be really interested to learn about and understand is, what it feels like to work day-in, day-out in a market-leading, world-leading experimentation program. So, on the ground, when you're operating at peak, what sorts of things you seeing, and what does it feel like?
Lukas Vermeer 16:32
Oh Boy, this efficient, now it's went. I mean, like when you're in the middle of that, I don't think I realized all that much. And it looks like that from the outside. Because we wrote about it, right? We wrote as if we have fully appreciated and understood what we were doing. But really, we're just living the day-to-day, I’m like, it's just a job, man. It's just an office you go to and people you work with. And then I think in hindsight, those were some of the most brilliant people I ever worked with, I mean, it still is an amazing team, like I’m nostalgic about those times. But I don't think it was a very deliberate conscious thing where like, day-in, day-out, we were thinking like, yes, we're operating at peak. No, we're just doing our thing. Like there's always ship broken. There's always stuff to fix. One of the things, I found interesting actually is that people from the outside would look at booking and say, “Oh, you're operating at peak efficiency, you're doing so well in experimentation program.” Whereas, the conversations I was having with my own team about what we were doing, because we saw all the risks.
The thing is, if you're running 10 experiments on the side, in your marketing department, and you're making mistakes, that's not so bad, because it's really not that much of a threat to the business. But at booking when you're at that scale, and pretty much every single product decision is supported by experimentation. If you're not running those experiments to sort of the utmost quality standards, then that is a real threat to the business. I would argue it's less of a threat than sort of not running experiments, I’m guessing. But it does put a lot of responsibility on the people responsible for the infrastructure and the people supporting the decision making process, because they understand now that all of the decisions in the business are going to be in some way supported by what they're doing. That's a lot of pressure to put on a Central team, not because they're making the decisions, but because they know if we make a mistake in the data collection process, 2000 People next week are going to make the wrong decision. Right, that's a lot of pressure. So, I think we didn't really think about it in terms of operating at peak, we really felt sort of the pressure to perform, and to sort of give these 1000s of people running experiments, the best possible data and the best possible support. It’s a rush, I can tell you.
Gavin 19:27
Do you feel that with that level of maturity, then the focus can shift somewhat to really being sharp with decision evaluation and decision making?
Lukas Vermeer 19:45
This is one of the things that I came to realize and I find this fascinating, when I talk to other people in the field, is that there's no such thing as a perfect experiment. It does not exist, right? There's only, it's a continuum between very well executed detailed experiments, and sort of winging it off the seat of your pants without an experiment. And between that there's a large Scala of different options. And I've always thought about this as a rising tide floats all boats. So, the intent is not deliberately not to make very few super high quality decisions and then wing the rest. Because that is what, going to happen, right. The number of decisions that the company makes is almost like a constant. It is a fixed external thing, that this company is going to make decisions, whether you like it or not. And I think, as a decision support team, you have to think about how do we improve the quality of decisions, overall, all of them, not just a few that we are actively involved in, but all of them. And I think you do that by looking at the broad Scala decisions that are being made, and saying, “How do I make all of them just a tiny bit better not take a few and make them perfect.” Again, that's impossible, but make all of them just a tiny bit better.
I think, this is one of the key mental models that I took away from booking also, is that you look at sort of all of the experiments that people are running, you try to identify what are the threats to validity, that are being made, or that are happening here. And then you try to address those at scale. So, not by intervening and sort of going to an single experimenter and saying, “, you forgot to do a power analysis, can you please do a power analysis?” Right, but thinking about why did this person not do a power analysis, what would enable them to do a power analysis next time without only my direct involvement? So, take the thinking about this, as that the decisions will be made. Whether you like it or not? How do we improve them at scale for the next one? I think that's the key.
Gavin 22:02
So, thinking about some of those other mental models you formed at booking, what would some of those top mental models be?
Lukas Vermeer 22:12
I don't know where he got this question. But does anyone deliberately form mental models? And when they do, are they like aware of them, because I'm not like, I learned a lot of stuff over the years. And I would say, 80 to 90% of it isn't conscious, like these are just things that seem normal to me, or that I consider to be common sense. But when you explain that to someone, they go, “Oh, that's interesting.” And I didn't even realize that was something that you could consider, right. This happens to me all the time, when I do consulting, when we're talking about something and I explain, how I view something. And then people go, like, people respond as if it's some deep insight or epiphany, where that's not what it was at all, like these are just habits that I picked up along the way. It's not a conscious, deliberate thing. I guess, there's what we mean by lived experience, but having mental models.
And so one of the mental models is what I just described, that the decisions will be made. And the job isn't to make a few perfect ones. But the job is to make most of them better. I think that is a key one. The other one that I picked up, thanks to a man named, Chad, who was one of the developers on the team. Brilliant guy. There were a lot of good human beings on that team. Like, they're not just good developers, good as a good data scientist, but just good humans. He was one of them. And the model is that you can't really force people to think or understand. And what we want with experimentation is, we want people to understand what they're doing and think about what they're doing. So, that they can learn about the customer experience. That's what we want to achieve. And we cannot really force them to, like, you can't say, you must write a Hypothesis or you can, but what they're going to do is basically master hands on the keyboard and sort of input random strings into a field because you made them, right. So, this idea, for example, that you can make a power calculation mandatory. And I think is a fallacy like I don't think, you can make those things mandatory, you can make filling out the field mandatory. That doesn't make thinking mandatory, right, we'll just put random gibberish in. And the model, Chad came up with is actually is an eight-step model, where before you even contemplate making something enforced, there are seven other things that you need to do. And I can't run with them off the top of my head, but they're basically things like it has to be possible first, right? If currently, it's not possible for people to enter a power calculation result in the tool, then how are you going to expect them? How are you forcing them to write? First, you have to make it possible then you have to make it easy, then you have to make it desirable. Then you have to make it default, then you had to make it encouraged so basically, you can sort of escalate the amount of pressure or the amount of nudging that you're using to encourage people to comply or to follow these procedures.
And so, I think a nice example is that a lot of these experimentation platforms that are on the market, they will ask Users to pick their primary metric. And then when you do, that is the first metric that they show on the report. So, when you run the experiment, the first thing that they show you is that primary metric. Now, that is a way to make it very easy for people to indicate what is their primary decision metric, right? Not all platforms do this, some platforms just give you 500 metrics, and then allow you to fish after the fact. Now a statistician will tell you, that's a bad idea, you shouldn't be able to pick like your metric post hoc, you should be picking it upfront.
But rather than saying it is mandatory to input this information, right, these platforms are giving you value in return, you've picked the primary metric. And that's the thing that they show you first, which for you as a user is one, it's easy from a U-X perspective, but also gives you immediate motivation and incentive to actually comply with this part of the process, that idea you can extend out, right? So, if you think about the booking platform, or you think about A-B smartly that I'm working with at the moment, they don't just ask for the primary metric, they also ask for the expected direction. So, is it? Should this number go up or down? And by how much? Do you expect that it should at least go up or down? Now, those last two are essentially the minimum detectable effect that the statisticians have been trying for years to elicit MDS from people, right? By asking them, can you please do a power analysis or by saying it is mandatory to do power analysis?
But I think that the answer is, that should actually make it easy for people, not say, it's mandatory to do it, but to say, what is the easiest way that I can elicit this information from a user, what is the most value and immediate value, I can give back to them by displaying this information as part of the report, for example? So, the booking tool, when you enter this information, the report will actually change to adjust, so, that you don't have to remember what the direction was that you were expecting, it will actually highlight this, and then you don't have to remember what size effect you were expecting, it will show you. You don't have to do the power analysis yourself, it will do it for you. Because it has all the information now that it needs to do these things. So, the user is incentivized to follow protocol correctly, because the tool is giving them value back, I think this mental model of experimentation as a strict protocol, can be accomplished, not by enforcing the protocol, but by giving users value back when they stick to the protocol. And by encouraging those defaults. I think that is a key mental model. And that relates to what, I was saying, earlier, where you want to support as many decisions as possible, you want to sort of rising tide floats all boats, you want to elevate all of them. And the easiest way, I think to do that is not to act like the police and enforce it at scale, because that doesn't work. But the easiest way is to give immediate value back so that when people make similar decisions, they come to you and say, actually, for this decision, I also want that value that you're giving me. So, it's suddenly it becomes push and pull, right? Suddenly people come to you and say, well, actually, this value that you're giving us, we would also like to see it here.
Gavin 28:34
Right, you touched on compliance. So, rather than being compliant and a carrot and stick approach, it's really helping people to become more effective in the role, which ultimately they want to do, they want to be more successful.
Lukas Vermeer 28:48
Yes, I guess, you asked about mental models, I think another mental model I picked up is that building or sort of building for internal Users or building an experimentation platform, isn't all that different from regular product management or product development, right? We think about these websites that we are building, where users come to the website, and we want them to convert, we want them to buy our stuff. And to encourage them to buy our stuff. We explain to them why our stuff is the best. And then we make it really easy for them to flow through a funnel and ultimately they give us their money. Right? That is basically what product management is trying to do, sort of solve User problems on the way to giving you money. And experimentation internally in the company isn't very different, right? We want people to make better decisions. We have a lot of Users in our systems. We want them to make those decisions using our tools.
We have to think about how do we encourage them to come to our platform? How do we make the platform as easy as possible so that they go through the flow and ultimately make decisions using the platform. This isn't a whole lot different from regular product management. You could even define like a funnel for experimentation and say, well, these are all the decisions that are out there which is equivalent to sort of the market, the entire market. And saying, how much market share we have like for how many decisions when people come to us. And then all of that market share is. If the market share is small, then maybe we should do some marketing internally, right and get more people to come to our platform. And of the people that do come to our platform, how many ultimately do set up an experiment? Right? So, how many convert down the funnel? So, you can model it in exactly the same way.
That means, you can use all the same books and techniques. So, one of the books I've been using, don't tell anyone that just. This isn't being recorded, right? The influence, Cialdini is geared towards sales, right? It's geared towards marketing. But actually, when you read, Switch about change management, and you read Cialdini, like how do these ideas are the same, that reciprocity doesn't just work, when you're selling things to customers, it also works when you're selling things to internal stakeholders, right? You can apply exactly the same things, highlighting the bright spots in Switch, is essentially just social proof. Right? You find places where things are working. And then you go, look at this team over here, they're doing great, right? The VISTA hiring me is essentially authority, right? It's basically they hire the guy. And so, we now have the guy who does experiments, right? Now we're going to do experiments. And that's just proof by authority.
So, these techniques that we think about in the context of experimentation, as being the content of the experiment. So, the things that we're trying out on our website, in order to convince users to buy our product, those exact same techniques, you can apply to experimentation in general, in your own company. So, well, actually, I want to nudge people to make better decisions, what tools do I have at my disposal to nudge them in that direction?
Gavin 31:58
And I guess, overall, it gets back to that. That little loop. I think it was highlighted in Alexander's article about the flywheel investment, value investment. And yes, it feeds the whole flywheel on the experimentation program at large. Let's shift the discussion to Vista, now. So, thinking about those early days at Vista, what was your initial assessment of experimentation at Vista?
Lukas Vermeer 32:32
So, this is a good question, because I came in thinking that there was going to be almost nothing. And I was actually pleasantly surprised in the sense that there's actually a lot of testing already going on. One of the things I found fascinating is that, they've actually been testing for a very long time, as well. So, they started running experiments pretty much at the same time as booking did. The thing they didn't do was Centralize. So, there's a lot of pockets of the organization where experiments are being run at a pretty decent scale. Not 1000s. But I would say, the ones that were tracking it like 50, but I think there's more pockets that we haven't touched it, where there's more experiments being run. So, I would say, they're on the order of hundreds. And that was interesting to me in the sense that, where I came from. So, booking has a pretty much, since the beginning a centralized place where all experiments were stored, were retrievable, discussions were happening, people would look. So, there was a very. Even though decisions were decentralized. And I mean, we talked about this in democratizing Online experimentation, right? We talked about decentralization of decision making. But ironically, that is made possible by decentralization of infrastructure and documentation, right. People trust that this other team over there is doing the right thing, because they can inspect all of the decision, all of the data that's supporting that decision. So, the centralization of tooling makes the decentralization of decision making so much easier. And one of the things, I find interesting about Vista is that they do not have decentralization, or did not. When I joined, one of the first things we started building is figuring out like, how do we take these different tribes and help them speak the same language, help them put documentation in the same place so that they can learn from each other. Because I think, that is the critical mass that you need.
If you really want to scale, then people need to learn from each other. And they can only do that if the entire thing is transparent. And transparent isn't. You could always ask me about my experiments, right? That is not transparency. And transparency is not, “Oh, I put all of my stuff over here in an accessible folder.” That's also not transparent. Transparency actually requires standards, because you need to have the same way of writing down things so, that I can understand what it is that you did. Because if you use different words to describe the same metrics, then even if I can read all of your stuff, I still won't understand it. And I still won't have transparency. So, you do need some amount of centralization and standardization, limited amount of how knowledge is documented, in order for the sort of the learning to happen at scale. And one of the books that was really influential there, which inspired my thinking, but I would not recommend anyone read it. It is “Communities of practice,” which is a very Academic piece of work. But it describes several groups of people who learn from each other, a practical skill. So, the classic example is tailors, street tailors or butchers.
There’s a lot of practical details to that skill, right? It's not like you read a book about tailoring and then suddenly, you know how to be a tailor on the street. Like you learn this, from observing other people. So, this book talks a lot about how do these people learn. And so one of the things I find interesting is that, you learn to do these things almost backwards, in the sense that, you don't start with like a fancy suit, you start with underwear, because it's easier, right? And you don't start with the cutting of the fabric. You start with putting buttons on existing, almost finished garments, right. So, it's really inside-out, back to front. So, a master tailor will cut all the cloth for you, sew together and then say, “Please put the buttons on.” And once you know how to do that, then they cut all the fabric, give it to you and say, “Please sew it together,” right. And then, as you mature, as you get better, at some point, you will be cutting your own fabric, and then you work from inside-out or you start instead of making underwear, you start making shorts and sort of expand that.
There is a lot of parallels between these communities of practice that are being described in that book. And what I saw happening at booking, in the sense that when booking grew really fast, we brought people-in, who didn't really have practical experience running experiments. And then within weeks, they were running their own A-B tests. And like I mentioned before, like we were efficient as we doesn’t know, it's wet. Like I didn't think about how that was even possible, right? We had a one day training where we taught people the sort of the basic skills. And we always said, “This is a language course, this is where you learn the words to talk to your peers.” But I didn't fully appreciate how important it was that we then gave those people the language and then put them in a community of other people who knew how to run tests. I think that was the key that we took a developer off the street, taught them the words like significance, confidence, a power, right, teach them the basics, and then put them in a team with five other developers who all have been running experiments for months or years. And that is where they really learn how to run experiment. They didn't learn it from me, they learn from their peers.
I think the experimentation landscape at Vista to bring it back to the question is because it's not centralized, it's so fragmented. This is really hard. Because we bring in someone, we teach them the basics. Where do we put them? We put them in a tribe that is closed. And I'm trying to break open those silos, trying to sort of get those people to talk to each other. Because I believe that, I say, believe, right, I have no data to back this up. But I believe that if we get these people to talk to each other and they can teach each other and that is a much more scalable approach to improving the overall decision level, rather than having a centralized authority being the only one. So, I think that the central team should be teaching those few people at the peak. And this is also the model that the Lave uses in The communities practice.
There is a very selective group of people who are the absolute experts on a particular topic, then there is a first ring of people around that. That are very dedicated topics, spend a lot of time and they tried to really understand it. And they get most of their knowledge from those experts, they get, they really talk to the expert team. And then there's a super wide periphery of people who are just tangentially involved, like who are watching from the side and who are learning by observing others doing. So, a lot of the learning isn't actually happening between the experts in the first year, a lot of learning is happening between first year and the wide periphery. So, you have to create an environment where the wide periphery can actually see what is going on. And I think, this is one of the things that we're trying to engineer, is to figure out how do we create connection between the experts.
So, my team, expert team and first year. So, first, we have to create first year, how to create connection between experts and first year, how to create connection between first year and periphery? How do we make that process transparent so that other people can see what is going on and learn from it? And we wrote about this on the blog. Also, we wrote about the ambassador program. The ambassador program is essentially that first creating that first bootstrapping that first year, right? Ideally, this occurs naturally, organically comes into being but we sort of had to bootstrap it.
Gavin 40:47
That's a good segue into my next question around how you're organizing for scaled experimentation. You mentioned one of those pillars is in ambassador program, what are some of the other pillars?
Lukas Vermeer 41:04
It’s essentially a hybrid model, right? There's a lot of the main, a lot of stuff out there already about the different models that you can try. And I think, we are very much going for a center of excellence model where there's a central group of people, one of the tweaks we're making to that is, that we're saying, the Center of Excellence isn't just experts on experimentation, it also has to be a product team. So, we have a product manager for developers. Because I mentioned before, you want to remove friction from the process, you want to sort of create operational efficiency. And to do that you have to build stuff that people can use. So, just analysts isn't sufficient, right? So, the Center of Excellence has to be, I think, a combination of analytical expertise. And then a product development team, then the ambassador program is that first year I mentioned, that's the first line support. That's a group that is deliberately heterogeneous in the sense that we wanted to create connections between the different silos in the organization in different parts of the business. So, the ambassador program is deliberately taking from different parts of the organization.
They're creating human connections between them, so that they know to find each other and they know where expertise lives. So, it becomes sort of a highway for information between the different tribes that already there. It’s also deliberately heterogeneous in terms of background. So, it's not just analysts, it's also U-X, and product managers, and hopefully, developers soon, because we want that sort of group to have as diverse a Skill Portfolio as possible. And because we wanted to make sure that, this wasn't a side job, this was a sort of a clear and supported mandate. Every Ambassador also has a Sponsor in their own part of the organization. So, they don't report to me, they report to someone, somewhere else in the organization. And I go out to the sponsor and say,
“Hey, I want you to dedicate at least one or two days of this person's time to being an ambassador.”
So, on the one hand, that's a signal for me that they are bought into the program that it isn't sort of perfunctory saying, “Oh, yes, we will have an ambassador, but they don't have to have time for it.” I want ambassador from parts of the organization where there is buy-in and this is a way for me to sort of validate that there is buy-in. On the other hand, it gives the ambassador a clear mandate to invest time in this, like, it's not just like you are expected to do this. This is literally your job now. So, you're being paid to do this. So, it gives them incentive to actually commit to the program. And it gives them a piece of authority, right, so to do their own part of the organization, because I am the ambassador and this is my sponsor and here's how I'm spending my time, here's how you can see I'm part of the program. So, we very deliberately made all of these tiny decisions in order to sort of create a cohesive group that had authority.
And then, like I mentioned, you want the periphery to learn, right? So, we want the rest of the organization to learn from these ambassadors. And so, very much from the beginning, we said, an ambassador is the long term vision for this program, is for it not to be required. Like, if we are successful, this thing will go away. Because ideally, the ambassador program becomes an organic thing where every part of the organization has their own local resident. Know it all. That is, the person that you go to when you have questions about experimentation, and that shouldn't be sort of an Official program that is something that Organically grows. So, the long term vision for the master program is afraid to disappear. I mean, it takes time and effort to support it, right, ideally, we would spend that on other things. That really set the tone, I think also for the ambassadors, for them to realize, oh wait, actually, I'm learning all these skills now.
And I have a title now that is not forever. And that is not the end goal. The end goal is for me to teach everyone around me, so that I can go back to do other things. Right. And so it really set the tone also for the ambassadors to understand that their role isn't so much to sort of follow the curriculum and then sort of play this new part. But that really the role for them is also to figure out, how do I teach everyone around me to do the same things. You're about a year and so we'll see, how successful you are right? Because all of this is just an experiment.
Gavin 45:49
So, thinking around 18 months on now, into the Vista journey, what are some of those parts of the flywheel that you're working on to remove friction to get to spin faster?
Lukas Vermeer 46:02
Oh, there's a lot like that. I mean, this is the fun part about thinking about experimentation in this model, right? Once you start thinking about in terms of identifying points of friction and resolving them. And thinking about it, that's product development, you get to do things like prioritize, by effort reward, like the same way, we would do for a product by saying, like, how much value do we expect to get? And how much effort do we have to put in, you can do the same thing for friction, right? You look at points of friction and say, solving this thing, or making it easier for people to do this thing will take us like, six months and the number of experiments we can run additional would be like 10 a month, that is not worth it. That's not going to be something that we invest in. So, what bottlenecks of friction was. One of the things, I found surprising is that the vendor platforms that you buy off the shelf, they don't really seem to be built for a scale of experimentation. They're more like, they seem to be built for like, if you have one team that runs experiments. And then there's a dashboard that you look at that gives you the results, they don't really do the Process part in helping you document what was the Hypothesis? What did the screenshots look like? What decision did we make? Who made that decision? Why did they make that decision? Like those are all things that are maybe not part of the reporting aspect of the metrics. So, they're sort of put to the side. But I think from a from a scalable experimentation point of view, they are super important, because I need to be able to go into someone else's experiment and understand why they made a particular decision, or even what decision they made. Right?
So, the bottlenecks of friction points that we're seeing are around, at how do we document these things in a central place that they're accessible. Another that I found interesting was, how do you do Q-A? So, the ability to quickly turn things On and Off to see whether the experiment actually does what you expect it to? And how do you check whether metrics are flowing through correctly? So, how do you know that the thing is actually tracking what you wanted to track? These are all things where there was a lot of friction in the process. And people were doing a lot of manual work. And sort of having a few developers say, “Oh, you know what people are manually editing, look the vendor documentation in this case, literally said, open up the cookie in the Developer Toolbar and change that, copy the text in the cookie to something else. And that copy pasting it from one tab to the other tab to sort of change the cookie value. And we're looking at going like that the amount of errors that people can make and the amount of tabs and mind capacity that they need to do this.
So, one of the developers just created a simple Web App, where you click a button that changes your cookie, like that is the kind of friction removal that I'm talking about. That is like a developer spends maybe two days building that. And then like 50 people who are setting up tests can suddenly Q-A with a click of a button like that is the kind of friction that we want to take out of the process, if we want to get really efficient at this.
Gavin 49:07
Good point. Now thinking about, you know, experimentation. Notionally, experimentation is a business utility that can be used broadly across any business. So, how are you expanding experimentation beyond A-B testing at Vista?
Lukas Vermeer 49:25
Oh, that's fun. And this was one of the things that actually drew me to Vista is, that booking is a two sided marketplace, but it doesn't really control the supply. They like, the hotels decide what goes on the website. This actually does the production as well. So, they have factories around the world where the stuff that they sell, gets made. So, there's opportunities for optimization and experimentation as well. And so, places where we're expanding is what we published about them, time split testing for pricing. Testing, we didn't want to run experiments, where based on your cookie, we show you a different price that didn't seem like the right thing to do. So, instead, we flip the price by day, so every other day, the price might change. And then we use that as a way, it's essentially still an A-B test, except that we're randomizing on days rather than on cookies or users.
And then there's experiments that we're running in the call center, where a lot of customer support is done through call center agents, willing to figure out like, how do we make them happy? How do we make them efficient? How do we make sure that they help customers in a way that makes those customers come back? So, that's an interesting area that we're expanding in. And I mean, we haven't done this yet. But I would love to expand it onto the factory floor, I think so much of our business is sort of relying on efficient processes in the factory. And I would love to run more experiments there. And that's one of those areas where I know they are already running experiments. You’re just not documenting them in a central way. So, I would actually be interested to see what can the people who are running experiments in the call center learn from the people who are running experiments on the factory and vice versa? So, yes, that's, I'm looking forward to that.
Gavin 51:12
Yes, it's an interesting thought that like, the spectrum is very broad. There's a lot of opportunity right through from front-end, through customer acquisition, right through into logistics and supply chain. So, sounds like there's enormous possibility.
Lukas Vermeer 51:30
Yes, we did the same thing with booking though, right? So, booking has the call center Software, it is integrated in the same experimentation platform, so they can run routing experiments. So, you think about things like, if a Japanese customer calls customer service, but there is currently no one available, who is Japanese. But there is someone available, who is Italian, but happens to speak Japanese? What would a Japanese customer prefer speaking to an Italian who speak Japanese or waiting for a few minutes until a Japanese person is available? Now you could obviously ask them right as part of the flow. But you can also run an experiment to see which one of these leads to more abandonment and lead to more returning customers and more loyalty. So, that's the sort of thing that you can do in a call center that, I think from a User experience point of view, these things are super impactful, right?
When we talk about experimentation, we often talk about button colors, I don't actually think button colors are all that important. Apart from things like contrast. Contrast is super important. But I think when you think about the customer service, like that's a moment, that the user really needs help. And that can really impact the customer experience. So, ideally, I think you find those places where you can actually have the most impact on the customer experience. That's where you want to experiment.
Gavin 52:58
Yes, I mean, that could significantly increase retention and lifetime value. It's highly impactful. Okay, let's close up with four fast questions now. So, number one, what's one topic that we haven't discussed, that you'd like to discuss?
Lukas Vermeer 53:22
That's a really difficult question. I don't know. We talk mostly about organizational dynamics, organizational change. We didn’t, we hardly talked about statistics, which is good, because it's really a sideshow, honestly. So much emphasis on our statistics in the field were. Yes, I'm happy, we didn’t talk about that today.
Gavin 53:46
We had sort of plan to, we're going to dive into this SRM but…
Lukas Vermeer 53:52
Oh, right. Yes.
Gavin 53:54
But time is at a premium.
Lukas Vermeer 53:56
Well, you should definitely check for SRM just be clear, like let me get on my high horse for like 30 seconds. It is astounding to me that there are still experimentation platforms out there that do not check for SRM. It is super trivial. It's super easy to do, you can catch a whole boat-load of issues this way. Even the best experimentation companies and experimentation platforms in the world still suffer from this every once in a while. So, it's super important that you check for a sample ratio mismatch.
Gavin 54:26
One thing that I wanted to ask you quickly about that, do you feel that, you know, some people may be trivializing it that in your taxonomy of root causes, there's, you know, approximately 30 causes there that have been identified and very much the focus is largely around incorrect randomization that should people be looking more broadly and deeply at causes of SRM.
Lukas Vermeer 54:54
I think on the web, at least, that incorrect randomization isn't actually or likely cause because if there is problem with randomisation, we would see it in almost every experiment. And that's actually the easier ones to fix. The most likely cause that I've seen is actually missing data as a result of telemetry being affected by the treatment. So, if you change something about a Webpage, that can change how likely it is that the Browser can report back, that something was changed. And actually when we started writing that paper, I thought that was the only cause. I thought there was only one cause for SRM. And that was missing data. And it's only when we started writing the paper that we realized that there were other potential causes. Now, I don't think people are trivializing it, by the way and certainly I hope not. No, I mean the odds that if you find an SRM, the odds are that it's actually benevolent and that there's no problem or really slim, like, usually, your stuffs just broken.
Gavin 55:59
Number two, what's frustrating you the most with experimentation at the moment?
Lukas Vermeer 56:05
Yea, frustrating the most with experimentation. Off the top of my head, I can think of two. One is this recurring theme of bandits are better than experiment, than A-B testing. And people just completely confusing the two, like they are different. They're solving for different use cases, they're doing different things that one does not replace the other. Please, stop.
And the other is, there's a lot of emphasis on sort of new novel statistical techniques, for example, that allow for continuous testing, so, continuously valid P-values, or that do some form of variance reduction, sort of reduce the variance on a metric. And I'm not saying, that those techniques aren't correct. Like, I think both of them are interesting techniques. I just think that they're being over-sold or over-valued. I think a lot of companies still struggle to get the basics, right. And same with the multivariate testing, right? These companies are trying to run A, B, C, D, E, F G experiments and analyzing like a multifactorial experiment, before they've ever run a proper A-B. And running a proper A-B is hard. Like it's not easy. So, I think people should make sure that they can walk and run before they try to fly. Like I understand from a marketing perspective, it is interesting to be able to say, we have the newest fanciest statistics, like see from an application point of view, that is the least interesting thing. I think, platforms should help Users run experiments correctly rather than emphasize the newest shiniest statistics.
Gavin 58:08
Is there anything else that you're learning about? That we should know about?
Lukas Vermeer 58:14
That I'm learning about?
Gavin 58:15
Yes.
Lukas Vermeer 58:16
Oh boy. And I had a great conversation with Positive John about this, that I'm not actually that deliberate about what I learn and he was talking about like, how about serendipity and luck? And how do you get to where you are and sort of. And I realized that I actually haven't been very deliberate about the things that I learned. I've been chasing things that I find interesting, or that sort of tickle my curiosity. And I've been thinking about not in terms of learning, but more in terms of like, what opportunities do I create for myself. So, if I learn to do this thing, what new things become possible? It's actually a life lesson, I learned very early on in my career, which was very much the opposite where a sitting at a customer… I was a consultant, I was sitting at a customer together with a very senior consultant. And he was doing most of the stakeholder management, that sort of documentation writing, I was writing all of the code. And I assumed that he was a management consultant that he couldn't write a single line of code.
Until I think about six months into the project, something broke in production. And like, within a minute, this guy loaded up the terminal, logged into my development environment, fixed my bug in production and logged off again. And I just stared at him like wait, you know, how to code? And he turned around me like. Dead serious, Lukas, don’t tell anyone. I was like, what do you mean? Don't tell anyone. It’s like the moment that, this client realizes that I can code, I will also be asked to code and that will make us as a team, less efficient, because then we will have no one to do the stakeholder management, we will just be code monkeys, actually executing the tasks that we are given. So, it is very important, Lukas, that these people don't know that I fixed your bug. So, I got the credit for fixing the bug in production. And it made me realize that the things that you learn, they create opportunities, but they can also close opportunities, at least when you tell people about what you know, right?
And so thinking about what you learn, not in the sense of like, what are you learning or what new skills you're picking up? But what opportunities are you trying to create for yourself, I think has been helpful for me. So, what am I learning that we should know about? I'm actually learning how to do finance. And the reason for that is, at some point, I want to run my own business. And if I want to run my own business, I should be able to know how to do taxes and stuff like that. So, that's what I'm tinkering with.
Gavin 1:01:01
Yes, that's pretty important. Right. Okay, last one, number four. Top three resources, non experimentation related, that you don’t tell to listeners?
Lukas Vermeer 1:01:16
I don't like that constraint? Top three resources.
Gavin 1:01:22
Constraints are one of the best ways to ideate right?
Lukas Vermeer 1:01:24
Yes, fair. Actually, I cheated a little bit, open up my Goodreads. And I started looking at sort of what are the books that I've read, that had a big impact on me? Well, I've already mentioned Switch. I think that was good. I think predictably irrational for me was one of the first books in sort of the behavioral economics that changed my view on how humans operate. By now, everyone's heard of “Thinking Fast and Slow,” which came later with predictably irrational was actually built on a lot of the same work. If you've read, Thinking Fast and Slow, you should actually also read the undoing project, which is the biography of Kahneman and Tversky. Amazing book. I lost count. And then the Radical Candor and the Culture Code, I think in terms of sort of organization dynamics and what it's like to be a boss and how teams operate.
“The Five Dysfunctions of a Team,” it's also good by the way. So, I mean, top three issues is, it really depends on what you want. Like, it really depends on what you want to do. Like if you want to be like a manager, or you want to be a people manager, then you read different books then if you want to be a runner, right? If you want to get better at running, I highly recommend endurance at 80/20. Like those are books that changed how I view running, but I wouldn't go out here and say like, “Hey, I recommend everyone to read those books.” Because I don't know whether you want to run. This idea of like top three recommendations. I don’t know. Send me an E-mail. Tell me what you want to learn. I will reply with a bunch of books to read.
Gavin 1:03:05
Good open invitation, there to the audience. Lukas, let's leave it there. Fantastic chat. And thank you so much for joining us today, on the Podcast.
Lukas Vermeer 1:03:17
Thanks for having me, Gavin. That was a blast.
Highlights
The Experimentation Flywheel - if you want to scale experimentation culture, you need to identify where the friction points are in your flywheel, and remove them. What is stopping you from performing twice as many experiments? Where are the bottlenecks in your flywheel?
Scaling experimentation in an organisation has two components - Operational Efficiency and Change Management. Pushing ideas out the door as fast as possible is process optimisation. How you help people to understand and appreciate experimentation is change management. Both of these components need to happen at the same time. If operational efficiency is low, it’s going to be difficult to bring people on the journey
If you want to change organisational mindset, focus on the things that are working and highlight them. Show people which teams are performing experiments and the success from doing it
The “Experimentation Ship” is always broken. There’s always stuff to fix. “As an experimentation support group, a lot of the conversations were very negative … in the sense that we were talking about all the problems that we were seeing, and all the ways that we could still improve. There was a strong drive within the group to make Booking.com even better at experimentation”
Quality Standards - if you’re performing 10 experiments per annum in the Marketing Department, and making mistakes, there’s a low business threat. However, when you’re at the scale of Booking.com, and with so many business decisions supported by experimentation, quality standards need to be of the highest level, or else the threat to the business is real
Pressure to Perform - at Booking.com there was a lot of responsibility on the teams supporting the decision-making process. “If there were any mistakes in the data collection process, next week 2,000 people will be making incorrect decisions”
There’s no such thing as the perfect experiment. The notion of the perfect experiment doesn’t exist. There’s only a continuum between very well executed experiments, and winging it off the seat of your pants
Decision-making is a constant in any business. The company will always be making decisions, whether you like it or not. As a decision support team, how do you improve the quality of all decisions, not just those that you’re directly involved in? “How do you make all decisions a tiny bit better, rather than few big decisions perfect?”
It’s difficult to force people to think or understand - what we want with experimentation is for people to understand what they're doing and why they're doing it … so they can learn more about the customer experience. Making tasks mandatory doesn’t make thinking mandatory!
Building an experimentation platform is like regular Product Management - Who is your target customer? How big is your market size? What customer problems are you solving? What is your CVP? How do you communicate and attract customers? How do you convert customers? How do you engage with / retain customers?
If you really want to scale experimentation, you need to think about establishing Communities of Practice - people need to be able to learn from each other through peer-to-peer communities. Ring 1 - Experimentation Experts, Ring 2 - Experimentation Ambassadors, Ring 3 - Peers (business units/teams/squads)
Experimentation education is more about teaching people a common language so that they can communicate and learn from one another in a transparent manner - this is a more scalable approach to improving decision quality across a business
The democratisation of experimentation comes from the centralisation of platforms and documentation
In this episode we discuss:
What it was like growing up with academic parents
What is stopping Vista from running twice as many experiments
Why scaling experimentation is part Operational Efficiency and Change Management
What it feels like to work in a world-leading experimentation program at Booking.com
Why it’s better to make more decisions a little bit better
Why building an Experimentation Platform is like Product Development
How you can use Communities of Practice to help people learn from one another
How Vista have organised for experimentation
Why it’s important to take experimentation beyond A/B testing
Episode Resources
Book - Authentic: A Memoir By The Founder Of Vans
Book - Switch: How To Change Things When Change Is Hard
Book - Influence: The Psychology Of Persuasion
Paper - Introduction To Communities Of Practice
Book - Communities Of Practice: Learning, Meaning & Identity
Book - Predictably Irrational: The Hidden Forces That Shape Our Decisions
Book - The Undoing Project: A Friendship That Changed Our Minds
Book - Radical Candor: Be A Kick-Ass Boss Without Losing Your Humanity
Book - The Culture Code: Secrets Of Highly Successful Groups
Success starts now.
Beat The Odds newsletter is jam packed with 100% practical lessons, strategies, and tips from world-leading experts in Experimentation, Innovation and Product Design.
So, join the hundreds of people who Beat The Odds every month with our help.