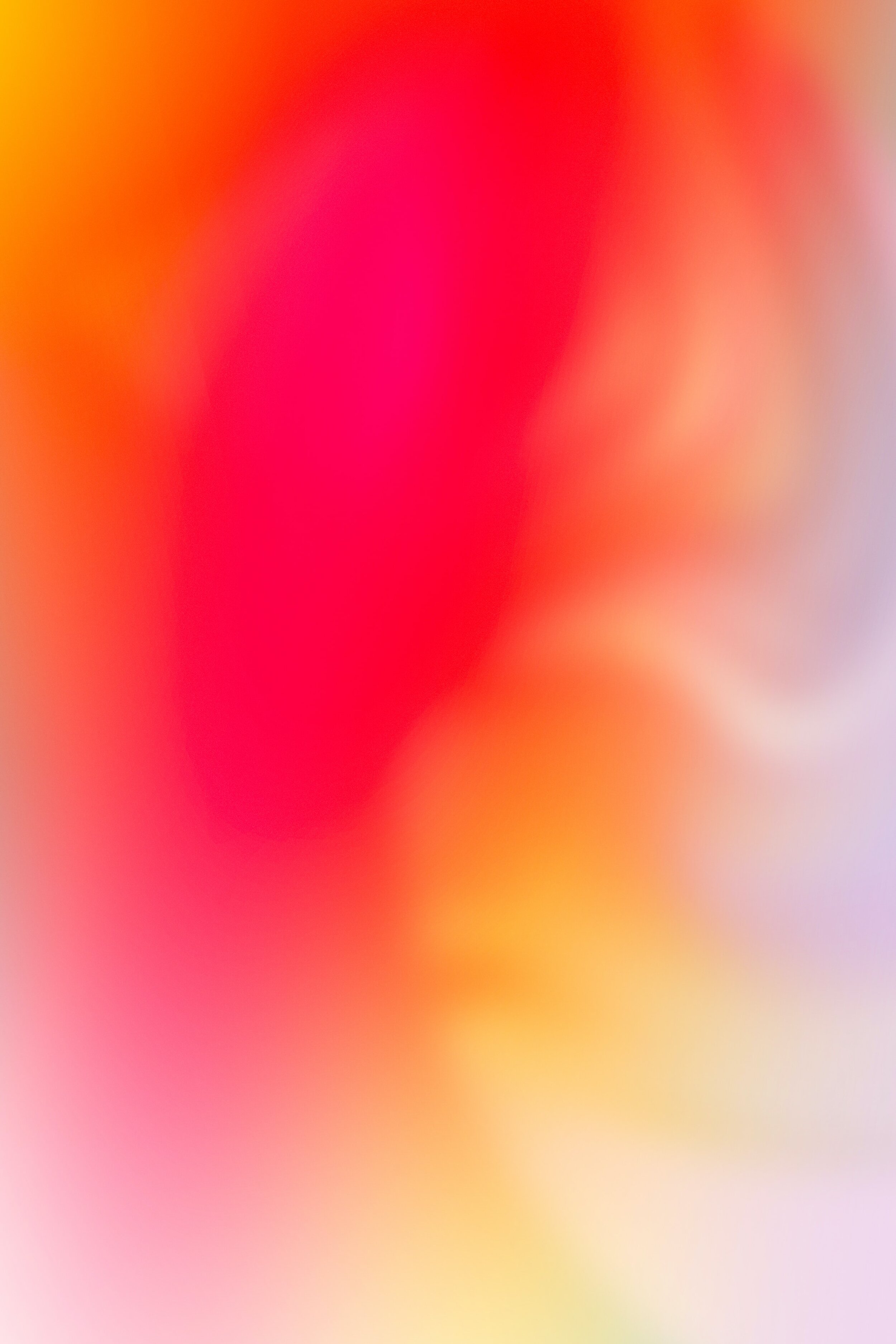
September 16 2022 • Episode 013
Vishal Kapoor - Marketplace Experimentation: From Zero To One (Part 2)
“Testing big bets, we always want to start very small. The bigger the size of the bet, the more risk. If you take a big swing and miss hard, there is a big business impact. It costs a lot to take a moonshot. You want to be even more surgical, breaking the big bet down into small experiments.”
Vishal Kapoor is Director of Product at Shipt. Shipt, owned by Target Corporation, is a marketplace that facilitates delivery of groceries, home products and electronics. With more than 300,000 Shipt shoppers delivering same-day customer orders across the United States, Shipt was recently valued at $15B, with revenues of more than $1B per annum.
He is a highly experienced senior product leader, with deep expertise spanning Marketplaces, Transportation, Advertising, Search, Messaging, Gaming and Retail Industries. Vishal has built, launched, and scaled next-generation consumer products that have generated billions in revenue.
Prior to Shipt, he was Lead Product Manager, Marketplace Pricing and Intelligence at Lyft and led growth of Words With Friends at Zynga. Vishal has also held roles at Amazon, Microsoft, and Yahoo focussed on Advertising and Platforms.
Get the transcript
Episode 013 - Vishal Kapoor - Marketplace Experimentation: Zero to One (Part Two)
Vishal Kapoor 58:39
Right. Awesome. All right. Let's talk about whether we can do something better. So far, we have not talked about our biggest state, our biggest market, which is New York. Let's bring that into the mix, and see if we can potentially create something or create an analysis technique where we can make the analysis a little more robust. So in California, we said, you know, there was some external impact, some celebrity released a book, so California metrics were elevated. Can we actually do something where we can kind of bulletproof ourselves against that effect, that external effect on the control, especially the control not the variable, but the control? Can we actually bulletproof the control a little bit? Make it more immune to external effects that may happen when we are doing the test? And the comparison, can we make it more immune? That this technique is actually called creating a synthetic control, and what this does is really, it uses different, and this is typically used in geographical context, but that doesn't necessarily have to be. It could be used in a temporal context. You could use different slices in time, but generally this is done in geographical context. It's just easier. What this shows is that, what it will do is can be used more markets with California to create a control, which is more robust against external interference against external biases.
So here, for example, what we would do is, we know this right without making any change the unpaid sales in our variant in Washington, the chosen state, they were 40k. In California, let's say that was, now we're making a synthetic control, so in California, your sales were 60k. In New York, your sales were 100k. Let's say can we create a control so that the effective sales are actually the same. So for the variant, all of your data is in the variants, so all the sales are in the variant, all the 40,000 books, the weight is 100%. But in California, you basically take 37.5% of the total sales, so 60 over 160. And in New York, you take 63% of that. So this is a very made up example, but really what we're doing here, is we are now not comparing only with California, we are diminishing the weight of California, in control. That's what we're trying to do. And we are trying to bring New York as another example. In the real world, what will happen is Gavin, we will basically act so you can see in terms of weighted sales, if you use percentages, left side is 40k, right side is also now 40k. Assuming sales is our metric that we are measuring on, you create a synthetic control on that metric by using many geos. This example just shows us 2, but in the real world, it's usually a combination of 15, 20 25, up to 20, by 30. Like any number of geographies, any number of markets, the idea is to create a combination with this kind of a weighted combination of different markets. So if something external happens in the market, it's actually unlikely to throw the average.
So now when you're measuring Washington against that nothing went wrong in control, nothing external went wrong in control. And now you can be more sure that Washington is probably, the lift you're seeing in Washington is a true change versus a synthetic control, because it's difficult to move synthetic control. It's difficult to have an external effect in all the geographies which comprise the synthetic control, if that makes sense. So that's generally the technique that is used, so we do that. Now what we're saying is okay, instead of looking at only California, we are going to look at 14.8 1000 sales in California... Sorry, instead of looking at entire California, we're going to look at about 15,000 sales in California, and about 25,000 sales in New York combined that, only take that when the sales are happening. We will take percentage of what was the revenue for that percentage of the sales, 14.8 1000 of the sales, what was the revenue? We will take a percentage of out of all the 100,000 sales in New York, what was the percentage, what was the sales for 25.2? Consider that, and that is our comparison number. Now, if for California, that went up, that wouldn't have such a large effect as versus before it just had a very large effect, it wouldn't have such a large effect in this example. So that's fine. It is more robust than doing a pure geo versus geo test, right? Comparing one state to the other. It's better. And the good news is it doesn't quite need similar trends. It doesn't quite need it, meaning you can construct a control over a period of time that can mimic well, it mimics similar trends. It doesn't need them to construct a synthetic control. It just mimics the same central systems. That's the goal here. But the problem, again, is that it cannot discount for effects. So now it is making your control more robust, which is good. But that doesn't discount, it cannot make your variant more robust, unfortunately.
So in Washington now, what if a competitor closed all their stores in Washington, causing an inflation in Washington sales, that's still something that we have not accounted for through this technique. In practice, maybe the way you can work around this, if you don't have a more sophisticated way of experimentation, the way in which you can work around it says, use multiple states, use Washington, use Nevada, use something else. When you have multiple states or multiple cities, and then you know that directionally at least if you have a very high lift in sales. If you have like a 5, 10% lift in sales, which sounds pretty high. And that happens in at least two out of three states, you directionally know. If it happens in all the states, then I think you can be more confident, you just get more data, you can be more confident that it's not a fluke. It's not by noise that it only happened in Washington because something happened in Washington, which elevated Washington's results. So you can kind of discount for that. Using synthetic control is a technique using multiple variants is a technique where you can actually make somewhat reliable. This is called quasi experimentation. By the way, in the real world, they don't call this an experimentation technique. It's a Quasi experiment, not fully scientific. But still you are a little bit better off than everything we have seen so far.
So people use that in the real world, in the absence of creating some more advanced tests, which we will see in a minute. This is still something that is a healthy balance to make some large decisions. If you want to run your business this way, this is not as bad as the ones that we saw before. And I think one of the examples here just to close this out is this was used to analyze the effect of usage of cigarettes, cigarette smoking, because of sales tax in California, and in California, so there was a proposition released, there was a law released called Proposition 99. And there was a sales tax introduced because of that, what was a drop in the smoking rates in California. And the way they did that was they looked at smoking rates in a lot of other states in US and created a synthetic control. And only in California, they applied the tax, and then they basically compared what was the change in California versus these other states. This control, which was synthetically created it kind of makes it more robust, versus just relying on one state versus the others. All right, I think with that we will come to one of the testing techniques, that is probably the most popular, and, you know, the most widely used in the industry. Gavin, have you heard of an AB test before, or an ABN test just once or twice? Okay so, truly an AB test is the one technique that is used far and wide in most of the technology companies. So, if my audience wants to walk away with two insights in this, pin out of this presentation, I would ask them to focus on this, and then ask them to focus on the next one. The next one is far more complicated to execute, but that is truly the one that is the latest and the greatest technique that we use in marketplaces. So we'll come to that. But this is fairly statistically, this is fairly data driven, there's high statistical confidence. This is a technique that is used for a lot of business decision making. So this is something that I would love for my audience to you know, read more about, educate themselves about what is an aviator, what is it AB test. So let's take that example forward, right. So, suppose in this example, now, you take two books, you take a book A, and you take a book B, with similar weekly sales, both the books have the same releases.
So remember, we said that in the public, the publisher sells many books across three different states. You pick two books with similar releases, they sell about the same books per week in the variant, you drop the price of one book, which is book A to $9. So remember, your hypothesis is to test that the drop actually increases sales, right? It doesn't mean you have to experiment on your whole state, it doesn't mean you have to experiment on your whole stores, it can actually mean you can experiment on a single book title, which is really good. And the reason I say that this is this, again shines light on the previous point I was making, you would rather experiment on one or two books, versus actually doing it across an entire state. If this works, then this is a good, it's the least costly for us to actually make it happen. This is the reason why this is the most popular as well. So you drop the price of one book, to $9, and next week, what you see is that the sales of that book A versus B, are now up by 10%. Now, this is fairly, this feels like there are two books in the same store in the same state, everything has been the same. All you're saying, literally what you've done is you've dropped the price of book A to 9, with nothing else changing and the price of that book is now 10% up by.
Now, I think we are getting somewhere. Now it feels like there is little room for error. Why like could this be noise? Could there be something external? Should we assume that if it worked for A that now this will work for all the books like that. Now we feel like we're getting something, so let's talk about that. So this, this is something else that I want to talk about. And this is a little bit of math. I'm sorry about that, but I'll try to explain in very terms what this means. So this is a concept called statistical significance or you can think of it as, how much confidence statistics gives you that when you observe a change, when you observe some effect, we talked about cause and effect. When you observe an effect, was that effect just random? Did that just happen without the cause? Or was it really truly because of the cause? That is your true causality. So that concept is called statistical significance. So the question, really, what we're trying to ask here is, we saw 10% lift in A, so that just noise? Is that just variance? Would that have happened without changing the price of the book at all? How do we know? How do we guarantee? And how do we get confidence in that?
Let's look at a similar question. Suppose you flip a coin, simple coin 100 times, and it gives you 45 tails and 55 heads, which is a 10%. Difference between the two, the heads are 10% higher than the tails? It's a similar example. Is this actually hedge bias? Or is this noise? Does this coin that you flipped 100 times, is this bias somehow that it lands on heads more than tails? Or is it just noise? Or is this just regular variants that any coin you tossed would actually is likely to say 55 heads 45 tails, or anywhere in the middle? How do you know when there is a 10% difference between the two results? That is random? Or is that noise? Or is that actually because the coin itself has some flaw in it? That's the real question that we're trying to answer. So here what this test does is, if a certain number of flips will give you a certain number of heads, a statistic statistical significance test, will give you an estimate of how confident you are that this is head spine, it's a number. It gives you the chance, the likelihood that this thing is head biased, this coin is head biased.
So there is a calculator, I linked it here. And this calculator, it says that if you have minimum 56 heads, and maximum 44 Tails, which is a difference of 12%, then you can say with 90% confidence that this is head biased. But if you need a higher level of confidence, then you need more heads and less tails for it to be biased. In that case, if it gives you at least 57 heads, and at most 43 tails. Now imagine that the heads are increasing, 55 and 45 isn't getting us anywhere. It's not 90% confident. I it is with some confidence, the confidence will decrease. But as you get to 56 and 44, now you're 90% confident that this coin is heads biased. Now you want to be even more sure about your business decision, you want to be 95% confident, so then you say that it has to have at least 57 heads and at most 43 tails, and that gives you a 95% confidence or a 14% difference or 14% lift between heads and tails, gives you that confidence. And generally in industry, the industry standard is to make business decisions on 95% confidence. When you have at least 95% confidence, you assume that this is statistically significant, it's sound, and then you move forward. That's not always true. If you go to, for example, surgical tests, like when you're testing in a surgery equipment, or when you're testing in something that you need a very high level of confidence you need 99.9999% of.... The failure rate of that needs to be very low, because the cost of failure is extremely high. But in online marketplaces, in businesses, in tech companies 95% confidence is supposed to be the industry norm, so that's what you prefer.
So in this case, using that heads flip, the heads analogy, if only 100 books were sold, and we saw a 10% lift, a lift of 10 can be variance, it's not good enough. You need at least a lift of 12, with a confidence of 90% to say that, when you drop the price for A, you drop the price by $1, you have to at least see a difference of sales of 12 to say with 90% confidence that the drop in price is what has led to sales. And you need a difference of at least 14 to say that a drop in price from which to save a 95% confidence that a drop in price has now actually led with 95% confidence to increase in sales. This is kind of where, we are getting into it a little bit, but I hope that this is a relatable example.
Gavin Bryant 1:14:39
Can I ask a quick question? Some of the academic papers on marketplace experimentation have proposed that toy modeling or creating scenario models of marketplaces could potentially be more effective than randomized controlled experiments. What are your thoughts on that proposition, from a practical perspective?
Vishal Kapoor 1:15:07
Yeah. You can't you can't you can't simulate everything you can't. That's my answer. You just can't like if we are testing a new pricing model, take this example, if you're testing a pricing model… you can run, you can look at your historical data. So I think that is… that's a great point. I think that is the reason why I started with a very simplistic scenario where all books are sold at $10. If there was some price variation done in the past for this bookseller then you could potentially look at pricing data and you could run some sort of an elasticity curve, you could plot you know at what price sales are safe… you know how much does that impact sales, you could actually do that. And you could do some analysis. But guess what that curve has to be robust across time it has the sale that curve has to stand you know in during your Christmas holidays that curve has to stand during your book month that curve has to stand during.
So unless you have that level of depth where you have done this you know nine ways to Sunday you know and you're a very, very analytical company where you monitor sales and you're able to do that usually, usually, the reason why companies test and do not rely on historical analysis is exactly that. What is what is going to happen now because the world is constantly changing why you test now and you draw an insight now and you launch it, you do it fast, like that's one of the you know one of the tenets of failing… one of the underlying, underlying, you know, the idea behind fail fast you know as people say is experiment fast, learn fast, and launch fast. And if it doesn't work, pull back fast, right? But the way you do it as I said, if you make a price change, you can look at historical data all you want but then that does not guaranteed you know take airlines as an example, prices change for airlines and COVID.
They were all over the place. How would you determine you can you can have elasticity course from the past? How do you determine at what price point the airlines are going to get sold? The world just completely changed. So that is the reason why an emphasis on actually trying at a small scale like this and then making a business decision at that instant in time. And by the way, that decision may or may not work in future, right. So you know, that's why these businesses are complex because you have to come back and think through, think hard through whether this is something that still holds or whether we need to do you know go into something else that may or may not hold. But that's how you build it like you know card by card by card, brick by brick, you actually build that house. But the thing is that you try… you try you run a fast experiment, you get data very quickly, you make a decision and then you actually move forward. So you can test everything, you just can't simulate it.
Gavin Bryant 1:17:42
Yeah, that was where my thinking landed as well, that it's very difficult to forecast and predict the future and test retest, retest it's a better model.
Vishal Kapoor 1:17:53
I mean, there is value in forecasting but everybody understands that forecasting is directional forecasting is based on historicals. It is not a true sense of you know it's not a true sense of, of what will happen in the real world. I will give you a very, very simple I don't want to get you know I don't want to get too negative here. But during COVID A lot of tech companies hired because they forecasted that, you know, their business will grow it their demand will grow, etc. And now, as you may see as right now and we're speaking there are a lot of layoffs. Those all the hirings were based on forecast demand forecasts, sales forecasts, and things like that. They expected the businesses to grow. But the reality is very different. And now people are unfortunately, you know, seeing mass layoffs in the tech industry.
So forecasts will take you so far, tests are the ones that doesn't mean there is no value to forecasting, there is value in in doing business assignment, there is value in doing portfolio management, there is value in doing there is always value in saying what do you expect to happen? You know and then actually trying to see whether you run an experiment whether or not the way we do it at Shipt, let me say it like this. Before we even run an experiment, we have some hypothesis. When we create a hypothesis, we have something called an expected outcome, which is if we change if we drop price, take this as an example. We drop the price of book A. What is the change in sales that we do that that we would expect? Now in this scenario, you know, our bookseller is a very simple, it's a very simple business, they are never tested, any differentiated pricing with everything was sold at dollar 10. But guess what, after this one time, let's say that they do this test, they launch it next time they have this data. So next time, they would actually do differently, right, they could fall back on these results at this time at this point in time, and actually use the results to draw some insights in the future. This could lead to a forecast in the future to creating forecast.
So we whenever we do hypothesis testing, any experiment launch we have something called an expected outcome, which is a version of a forecast when we run an experiment, a small experiment, what is the change in metric that we expect either an increase or a decrease? Depending on what we're testing? What do we forecast that metric to change. And then when we finish it, we actually see where we landed. Either we undershot or overshot. So it's some sort of a forecasting. Okay, as I said, right? The one thing I want to take, that I would love for the audience to take away from this talk is, this is the main technique that is used to make launch decisions in tech companies.
The advantage here, as we saw through the previous analysis, if you saw a lift of 14%, the advantage is you… that is statistically sound that is statistically significant. And that is backed by a very, very high confidence of 95%, that the book sales are… every 100 sales, if you see, you know, 14% lift for every 100 sales or 14% Lift overall, you would be you would be, you know, guaranteed that this is actually causing. And by the way, as the sample size increases, if 100 goes to 1000, 1000 goes to 10,000… the number that number that 14% actually gets smaller and smaller. That’s generally the way that works so you can use that test calculator, I'll just flip back for a second, you can use that it is a really just shows you out of the number of trials that you had, how many successes that you had in your variant and in your control, like a conversion rate. And if your conversion rate goes higher in your variant because of the change that you made is that true… At what confidence level, is that a true lift? Or is that just noise, you know, so that's what it is, and the bigger and bigger and bigger your sample sizes.
So take Amazon as an example, right? Amazon sells millions of books. For example, if they did this change, they would probably know, they wouldn't even have to wait a week, they would probably know within 10 minutes, right? Whether or not because they have so much so much sales coming through book books are an example. But you know, take any general merchandise, so much volume coming through within 10 minutes, you would have enough samples, where you would have seen, you know, not even 10 minutes, like ad systems, advertising platforms, they show so many ads, within minutes, they know whether or not an ad is actually seeing enough user interest, whether users are clicking on it, or users are not clicking on it. Whether this is a good ad, or this is a bad ad, they don't have to try too much. They will show that ad like within minutes, they will show that show that ad across the Internet to millions of users. And within minutes, they know whether or not somebody is clicking on it and whether this is statistically significant. And all of this, by the way, is automated in these ad systems because they are constantly they baseline this baseline other ads, good ads banner ads, every time a new strategy or a new ad comes into the market, they actually are trying to compare that with the baseline, right? So that's definitely our start so very, very fast.
When you when you get bigger and bigger and bigger, you don't need that interval to be 14%, it can get much, much, much smaller, then you can make decisions. You know, this is 0.05%. And this was what I was reading in the OKR [phonetic 1:23:00] book yesterday, the measure what matters, where they the Google engineers were literally obsessed about raising the rate of you know, views or you know, billion views per day, by 0.05%. They already had such scale, that if they raised it by 0.05% there, the scale was so high that they knew that that was happening because of a feature that they had launched, even that small of a lift does not happen by noise, it happens because of the cause the effect is because of the cause that you actually did in the marketplace. Just wanted to bring that point…
Yes so here, you can be very well, you can be very confident that the difference in metric, if you use the right techniques, the difference in metric is a true change. It's not a false positive. It’s not a false negative. It’s a true change. And because you're testing in the same scenario, both A and B are two books in the same store in the same state in the same region, etc. external changes will actually impact both the books in the same way, if another book C was released, taking the previous example some of the previous examples. If there was bad weather, one of the examples that we took, it could be bad, it would be bad for both A and B, if sales went down, they would go down for both A and B. Now you can do a relative comparison, right between A and B. And you know, if sales went down for both A and B, but A is greater than B, then you know that you can do a relative comparison between the two. If guess what if you know in California, if a celebrity releases a new book C that would deflate the sales potentially deflate the sales of both A and B equally, it doesn't mean that you no one would get there is no reason to assume that one would get impacted disproportionately versus the other.
So the relative comparison of launching a change on one versus the other... You're very confident making that decision as long as you know the as long as you are able to quantify the impact. The only the only place where this this does not work… This is coming back all the way to what we were talking about at the top of the talk. It doesn't work when the variant can impact control due to network interference, and this is the primary problem in, in marketplaces, where, where, when you're testing in a complex marketplace, where there are so many variables that are happening inside an economy, there can be network interference like this. That's the only thing. Any questions here, Gavin? would love to see if any, any, you know, any examples that come to mind that you have where you have used this term? You know… any comments from your side?
Gavin Bryant 1:25:29
One thing that came to mind for me was, as Director of Product when you're thinking about testing big bets? How does your approach change with relation to the types of experiments you're performing? And statistical significance, when often you can be more looking for signaling and directionality early on?
Vishal Kapoor 1:25:55
Yes. I would say that, regardless of how big the bet is, we always we always start very, very small. Always. That's just, if it is a big bigger bet, there is a bigger risk because if you if you take a bigger swing, and you know, miss hard, that is even a bigger business impact… You know, a business, a bigger opportunity cost for the business because it takes longer, it costs us more to actually take a bigger moonshot, you even you want to be even more careful. And you want to even figure out how, how surgical can you be? How can you take the big bet? And how can you really piecemeal it into very, very small scale experiments. And by the way, you don't have to run all the experiments, like in this example, you run one in California, you run one in Washington, you run one in New York, and then you know, and then you kind of like, you know, you try different things in different markets, which kind of looks similar and then you kind of scale that up.
So you run things simultaneously without potentially them interfering depends on what the marketplace is, right? In an online marketplace you know, it may not matter whether your seller is in Washington or in New York, right? Like, it doesn't matter. But in a in a local… hyperlocal marketplace, like shipped, which is you know Uber and Lyft. What you do in Washington… is potentially different from you can test Washington very differently from New York or Paris or London, for example. Like they're completely disjoint marketplaces, essentially. But yeah, but the but the tenet remains, the bigger the bet, the more confident you want to be that you are running more and more and more experiments, more and more hypotheses are validated. And, you know, and you are doing this bottom up, generally speaking, Gavin… generally speaking, right, product leaders, business leaders, generally want to take baby steps, they generally want to crawl, walk and then run, even for a big bet, they always want to take baby steps, because the risk of launching a big bet can be so much for a brand. And it can, taking a full swing on a big bet can potentially be… not only be reversible, but it can be very, very detrimental to your business. If you actually learn something without having full confidence, it may not be, it may not be a situation where you are able to pull out from unfortunately.
So that is the reason why you want to be even more careful, you actually want to give yourself more time to test. Like let it sink in like watch. Watch your long term user? What's the user behavior over a longer term, don't just give it a week, give it a month like wait… be patient, right? Because the bigger the bet, the more patients you need with that. So that's what I would say, in all the companies that I worked for, right? Even at even at Zynga… you know, my previous company, we launched a new version of a game, which was a very big bet for the company, I worked on a game called words with friends, which is where you play Scrabble, you're really playing Scrabble on mobile phones with your friends. And it's a very, it's a very nice sort of family connector, especially during the holidays because playing Scrabble is you know classical thing that families will come together and play during the holidays during Christmas.
We decided to completely, completely create a brand new app, which was a big bet for the company. You know, this app makes millions of dollars in revenue. We created a brand new app from scratch. But as I was telling you, right? The way you do it is we started with the existing app. And we started making a lot of small changes in the app, testing them. And then we decided they were big enough. And we were seeing enough lifts that we actually wanted to move them into the new one. And the new one, by the way, there was a parallel effort. And I and I led that effort at the company where we were starting a very rudimentary app, we started a very rudimentary app started taking that up scaling that up slowly. And you know, some of the other best practices from the existing app, some of the existing mechanisms, leaderboards, you know, competitions, things like that, which we knew worked in that app we actually migrated as is. But we did experiment with a lot in the in the in data at scale. We did take that experimentation mentality. And slowly this this bet slowly became bigger and bigger and bigger and bigger, with all the learnings, micro learnings that we had in that. And then we created this big bet and then we lost the new app. And as soon as we launch new app after a few, you know, after some time, we basically migrated all the users over. And then we even pulled out the older app. But by the time we had enough confidence, and by the way, big bet, this bet went on for a year and a half.
So it took us a long time to actually make that. But that's how you have to be patient. And it gave us revenue, like it gave us incremental revenue. It's not like we didn't see anything for a year and a half. Because you know, the features were building and users were starting to go to the new features that they liked, and we were promoting it, and so on and so forth. So it started building up. At some point, we decided this was bigger than what we had and better than what we had. And we cut everybody over. But that's usually how you build…
Gavin Bryant 1:30:38
Good explanation. Thank you.
Vishal Kapoor 1:30:41
Awesome! I think the last year sort of we're coming to the tail end of the presentation, the biggest issue that I would say, so AB [phonetic 1:30:52] testing takes you very far. Right, the previous example that we talked about takes you very far. But the biggest issue with marketplace testing in a small economy in an inside it inside a network is something called statistical network interference. And this, we talked about this a few times now, it happens when the variant will unexpectedly start to interfere with control, right? You don't expect it to happen but it unexpectedly starts to interfere with the metrics. So in this in our example, high sales for that for book A can potentially… we were talking of an offline scenario, but let's just take that, you know, let's say that book A is, you know, is let's say that the bookseller also has an online website, just for the sake of this, this conversation. Higher sales of book A can increase its rankings and popularity, or even in an offline scenario, right? A sells a lot more… It's you know, it goes into a lot of, you know, people's homes, it probably makes it into book clubs, and so on and so forth.
So the higher sales of A can increase its popularity, which can make people come and buy book A more and more and more. There are many books, which kind of organically you know, Harry Potter, right, like, you know, became organically popular, many people will come and buy Harry Potter more and more and more because it's more popular. But because of that popularity, it can start to cannibalize B, which is remember what we were talking about the seesaw effect, you don't actually intend that to happen, but because A is becoming more popular, it is now and you know, a buyer will only buy a certain number of books in a month, for example, or a week. So they if they are coming, everybody is now trying to now starting to buy Book A, it actually starts to decrease and cannibalize sales of B, which basically creates a cycle. Now B is not being popular, not becoming popular, because it starts going off people's bookshelves, A starts going on people's bookshelves. So it creates this kind of vicious cycle, where the metrics for… the sales metrics for B are actually going down, because there is an increase in sales metrics for A. And this is what I meant by, you know, it can actually skew that seesaw effect where it's not one to one, it's not one to one, unfortunately, that can happen.
In practice, in practice, now, this is where it, this is where it becomes this is where the art meets the science. It's actually it's impossible to avoid this in practice. And I'll give you one example, which I learned that I was scratching my head on it, this was a this was a while ago, and I was like that's true. I mean, it can go you can take that down to a very, very deep level. So let's talk about that. So what if the sales of A are potentially you know, causing longer lines in the store, which is actually reducing the sales force that can happen now A is selling more and more, and people get you know, people are the people want to buy B are like, I don't care about this, I don't want to stand in line. I don't care about this, like I don't want to… so therefore the sales are decreasing. How do you avoid this, you can't avoid this, right if A becomes popular. Now imagine if you drop the like, let me let me make a hypothetical just to bring the point home, if you drop the price of A to $1 or if you gave if you started giving book A for free, like if you drop into $0, everybody would come in a checkout line to buy book A like buy book A right, because they want to purchase book A. But then B is completely cannibalized and nobody is able to buy B because everybody's just looking to buy A, nobody's able to buy B at this point. So that kind of creates a cycle, which is now A’s sales are looking through the roof, while the sales volume is looking through the roof, the revenue is zero, but the volume is looking through the roof, and B is actually falling on the floor. And it is because an effect that A caused on B. It's not because of the change that you that's what... that is what interference is. And actually, when you take it to an online scenario, this is what you know... This is what you know, was an “AHA” moment for me early on when I was experimenting when I was getting into experimentation. Imagine if this was software on the same server… physical server. Right. And now there is you know, people are they're buying book A and a lot of people are buying book A, which is analogous to the previous bullet point. They are all buying book A which is basically creating a lot of load on the CPU on that... And therefore people who are trying to buy book B… Book B is not that popular, book A is the popular book, right? Because the prices have dropped, okay, so everybody wants to buy book A, okay, B is at the same price people want to buy B are just impatient. They're like I don't want to wait for like a minute for me to check out book B. I'm just going to give up and you know for doing that in a place where you know, that kind of experimentation where you compartmentalize everything… fully compartmentalizing experiments where something is running on one side of the server, versus the other is running in another set of servers, they are exactly the same. At some point, you cannot avoid two different versions of the same thing.
Vishal Kapoor
Okay, so with that, let's talk about the last technique, which is actually called Switch Back or Time Split Test. Now, for the sake of the audience, if you go and look at the blogs of the newer marketplace, companies like Uber, Lyft, DoorDash, Instacart, these are the popular companies in North America. This is the technique that is sort of the latest favorite child, if you will, out of all the experimentation techniques that we discussed. This is something that the companies are relying a lot on. What this does is this is a technique that can help you get around marketplace interference, essentially, that is the reason why. The technique is very simple. It is a version of an AB test, right? Where instead of you were actually splitting and compartmentalizing between a control and a variant, right? In our example, B was control, where there was no price change, book B. And then the variant was A where you dropped the price to book A, but you dropped the price by one dollars. What this is doing, and as we said, right, the sales of book A could actually come and cannibalize the sales of book B, which would look like the sales of A are actually inflated versus book B, which would actually cause a problem in your business decision making essentially. This actually helps you get around it. And how does that do that? So the foundational idea is very simple. It's a fundamental way of AB test. Instead of doing it across compartments, you're actually doing it, instead of doing it across geographies, you're actually doing it across time. That's really what it is.
So, in this example, instead of only dropping A's priced to $9 for one week, why don't we drop both A and B, and switch it back and forth. So both A and B go to $9, and then they go to $10, during the week and $9 and $10, and one week in one week out? It's a time split test, right? So it's going back and forth, switching back and forth between these options. That's really what a switchback is. One way how to switch is, one typical way in which you would do this is what if the price is set to $10 during weekdays and $9, on weekends, right, you could potentially do that. But a true switchback test is you actually want to randomize so that there is no time selection bias. Meaning, if the price naturally on weekends was high, the sales on weekends were higher, then you don't want to just keep switching price down to only on the weekend, because that would not give you a true result. Because that would just amplify the result that you already have in the marketplace, and again, it would cause a problem. So if you did that, if you only switched during weekdays to $10 and $9 on weekends, one of the issues with this would be that customers who are coming both on weekdays and weekends will actually see price swings, right? Because it's very reliable during weekdays, you're seeing $10 during weekend, now the same price goes to $9, $10, $9.
So instead of that, what you do is you create time blocks, and you randomly switch between them. So your weekdays is one time block, your weekend is one time block. And your $9 is one variant and your $10 is one variant. And you basically are just like weekdays nine, weekends nine, weekends 10, weekdays 10 or something like that. You just kind of like switching back and forth between different time slots. So in this case, what you could do instead of doing weekdays and weekends, what you could do is let's say if the bookstores are open every day, between 11 and 7pm even if you just want to switch back within the same day to avoid cyclicality, between weekdays and weekends, if they are open between 11 to 7pm, about eight hours every day, you divide them into equal blocks of four hours before and after 3pm, let's just say that, right? And then you switch random, you say that in the morning it's nine, in the evening it's 10, the next day in the morning it's 10, in the evening it's nine, the next day something else. It's purely random, you just purely are randomly selecting where what value is applied, but the beauty of this is that the entire market is actually going into the new test, everything becomes a variant. So, both A and B become a variant or they become controlled or they become variant or they become controlled. So now there is less likelihood of A actually impacting B because both of them are now $9 or both of them are...
So this is the reason why because it gives you this time based, instead of a geo based split when you do a time based split test. This is the reason why this is becoming very popular because it can actually help you get around market interference. And as a case study I've linked here, which actually has, it's a very nice blog from Lyft, which is a ride sharing company in the US, you know, one of my former employers, which actually talks about how they use switchback to work around interference in pricing experiments. This is a technique that that company used, for example. So, again, TLDR, you know, hard to construct, hard to analyze, because you have to now keep track of what blocks were $9, and you have to make sure that you are randomizing the $9 in enough blocks, if you only set $9, on weekends, but not on weekdays, then you're not likely to get a true unbiased result. Because the sales on the weekends could be different from the sales on weekdays, and so on. So that's truly what it is, it's a little difficult to construct, but it is fairly robust, because it is a version of AB test, which you are actually doing on a time speed basis.
So I think that is all that I have on testing. I will add one final note on what is the difference between a test, an experiment and an optimization, and optimizer, we'll talk about that. And then finally, we've talked about some key insights and key takeaways from the presentation. And then what can you walk away from the presentation? So, the main difference between what is an experiment and what is optimization is that statistical testing or experimentation, is generally used to choose a winner between many options, right? You're testing between A and B, you're testing a price of $9 or $10, that was a decision we were trying to make, whether or not even between A and B, you're just testing whether the price should be $9 or $10, and you're trying to choose a winning combination to raise your sales. So that's what you were doing. But actually optimization is to actually discover the true value amongst many options. So 9, $10, it could be $8, it could be $5, you could get the highest sale at any of those values between 0to 10, or it could be $11. You could actually raise, your sales could go lower, but your revenue, but not low enough, a $1, higher would not actually, you know, would lower it enough, where you would still see higher revenue. So the people's willingness to pay is actually higher, something that you alluded to before Gavin. So discovering that, and that is really the concept behind surge pricing, right. Surge pricing, it goes up and truly tries to discover people's willingness to pay, and then it kind of comes down, and so on and so forth, but dynamic pricing generally. So that is really in the realm of optimization, that's less of a test. And it's more of you know what numbers there are, and you're trying to maximize a certain metric by tuning certain numbers. So that is more of an optimization problem.
So the examples that I actually gave here, you know, so optimization, what is the best price to increase the revenue, $1, $2, or something else, the test that I actually gave you at the end, the bullet says that it's actually not the best example, for AB testing, it was just a representative example. It's truly once you make that decision, or you're able to make some decision, you can use it for some quick decision. Once you make that decision, you should really use it, you should really run it against an optimizer, which will be able to kind of tune those values, discover those values for constantly trying to figure out where is the revenue maximized? At what price point is the revenue maximizing? And constantly trying to test it. So that's not to choose a winner amongst any options, that winner might keep changing with time. And an optimizer will actually help you change that whenever, as time goes on. If that makes sense. That is the main difference. AB testing is good, very good generally for things like making launch decisions, right, which is, you know, should I launch a new feature? Or should I not launch a new feature? This book sale is, it's tuning a number. It's a unique example, but I use it for illustration purposes. But really AB testing is should I... the example I gave you before we launched a new version of a game. And a lot of those decisions, were actually micro AB tests, small scale AB tests. And eventually we decided whether or not we want to club them and launch a big game or not, that's where the differences are. There are many publicly available optimizers out there in the real world. Open Source optimizers, Google has an optimizer. Many optimization libraries that a lot of these companies will use for doing this kind of revenue optimization, forecasting, sales optimization, things like that. There are a lot of systems that scale back to this.
And finally, I wanted to end the talk with some final thoughts and key takeaways. The first thought that I will say over and over again is beware of interference and metrics inflation in marketplace experimentation, right? The biggest pitfall that somebody who's not well-versed known with marketplace experimentation, the biggest pitfall is you learn by falling, unfortunately, and it's costly to learn, it usually cost the company a lot of valuable time and money. And it confuses, especially new PMS who don't have a lot of experience with experimentation. It confuses them a lot. When they see why did the sales of my control drop when I did not make any change in control? It wasn't because of something external. It was because there was interference. Other markets did not see this, but my market saw it. It's analytically difficult to pass and you just, at that point, wasted a bunch of money and a bunch of time you haven't reached the decision faster. So that's one problem.
Second, I would say that although marketplace experimentation is complex, switching back is complex. Keep it simple, right. Stick with something like any AB test, whenever possible, stick with a decision test. Stick with something like an AB test, when you can potentially do a clean slate. It's not always possible, but try to make a clean split, and decide between two options as best as you can, right? So even if you're testing different price points, if you're testing, let's say different subscription packages, or values of the packages, make different packages, make different combinations, and then actually test different combinations and see, what is conversion, what is user's affinity to convert into one package versus the other? Instead of trying to formulate that as an optimization problem, don't try to run before you figure out how to crawl and walk, that comes later.
So, figure out how to do AB testing well, first and foremost, and then basically go from them. Learn some basic statistical techniques. That's misspelled, I wanted to call stats techniques, which is things like what is the minimum sample size? What is the P-value? P-value is the confidence that we were talking about. With what confidence do you say that this decision is not noise and this is a true lifting matrix? That's P-value. That also gets into statistical power and significance. What is the confidence interval? What is Alpha? What is Beta? Alpha, Beta is your probability of making a... it's called type one error or type two error, which is your false positive or false negative. How do you not make false decisions when the data is telling you something otherwise? There is a good resource here. It's a one small one page blog that somebody wrote and it walks through an example. So if audience wants to refer to it, I highly recommend you guys to see how, in a different example, how that author has actually used sample sizes, p values, significance calculator and things like that.
And here's three practical tips. How long to run a test for right? Usually, you want to run it for what I call as a Goldilocks period. You don't want to run it too short. You don't want to run it too long. I mean, I gave an Amazon example, I gave some ads companies’ example, which are at such a large scale that you would get an answer in minutes. That is true for some reason, at very high scale, and have been live for 20 years, right. Like ad systems were built a long time ago, Amazon was built a long time. But generally speaking, if you are running new bets, new experiments that is a tuning experiment, if you're running new bets, new features. I will say that run it for at least one business cycle. And business cycle is sort of what works for you, right? Sometimes a business cycle might be a week, sometimes it might be two weeks, it might be a month. What is your cyclicality of sales? What is your cyclicality of the way you make revenue? Or the way your users come back to you or whatever that may be, depending on what you're doing. So run it for at least one cycle. But run it for complete cycles. Run it for two, run it for three, don't stop at two and a half, don't stop at one and a half. Because then you're going to get like the business cycle is where you observe cyclicality. Across the cycle, you would observe patterns of cyclicality. Don't stop in the middle of a cycle. That's what I'm saying. Because then you will get half results or fully formed from weekend versus weekdays data if your cycle is a week. And otherwise, you're basically only looking at first half of the week, not the second half and all of that, that can get confusing. So don't do that.
I will also say that test it long enough, and avoid... This is something that I mentor my PMS a lot. Try to avoid bit test launch decisions based on positive results, based on novelty bias. A lot of times you launch features, you drop the price, in this example, and a lot of book buyers would come and want to buy but guess what that is probably going to fade over time, right? It's just because of... So give it a minute, look for you know, don't just jump the gun because you saw metrics go up for one or two days. That's unless you are a company like Amazon where you know what your long term metrics are you and you have benchmarked them over a long period of time. Don't make decisions on that short of a time period especially when you're doing feature launches. So give it at least... Generally speaking, what we do at the companies, we run things for at least two weeks, it gives us two full cycles, our cycle is weakly cyclical, it gives us two cycles, so that if one week goes off, we know that we have a way to sort of break ties. If it directionally is positive in both the weeks, we know that it looks positive, if one of them goes up, the other one doesn't. And at least we know that we are not taking an incomplete business decision, we might decide to run it for a third week, and that's okay, in order to break ties, but only running it for one week, when there might be something external that happened because things can happen. Something external that can no matter what you do, as I said, right, it's impossible to compartmentalize completely against the real world, things will happen. So run it for enough length of time, but not so long that now you are starting to cross over into a holiday or a long weekend, when the cycle is not replicated, right? Like it's not the same as what happened before. Don't do that. So it's a Goldilocks period, not too short, not too long.
A good rule of thumb, generally for feature launches, for product launches for app updates, for anything visual, or even anything that changes any user experience, generally two weeks. So run it for two weeks, and then go and take a business decision at the end of two weeks. Again, avoid the word novelty bias, because generally, that's what happens when you launch new features. A lot of early adopters are really passionate about your product, they will come and want to use those features, want to use those products early on. But if you give it a minute, if you give it a week, if you give it a couple of weeks, you will actually see that initial novelty tap down a little bit. And that's where you want to measure your steady state, not when there is early activity, that's not where you want to benchmark your success.
Gavin Bryant
No peeking on early results.
Vishal Kapoor
That is a statistical term. It's called peeking. So thanks for bringing it to the attention of the audience. No peeking on early results, because the act of being... It's like the Schrodinger's cat, it's a little bit of quantum theory there, which is the act of looking at something actually changes the results or biases your opinion and what that result is. So don't do that, run it. And usually your data science team, or even the calculator that I showed you, that also has another calculator, which is a time period calculator. So assuming a certain conversion rate. Let's say, you estimate that if this is what I was alluding to before Gavin. If you drop the book price from $10 to $9, you assume that the sales will rise by so much, assuming that there is a certain conversion rate, how long should you wait, so that you collect enough samples? How long would you have to wait to collect samples to make sure that you're 95% confident. That should be your testing cycle, really, because before that, if you pull the plug, you made a decision on 100 samples only, that's too early, you probably need to wait for like 1000 samples or 10,000 samples. And then beyond that, statistically if your confidence is high, even when the samples increase your confidence is high, and your sales are high, you know that this is not nice. This is because of the cause that there is causality, there is an effect because of the cost. So I would definitely say, don't peek, and don't make business decisions and launch decisions based on that. I will say from experience that I was mentored very early on, from some of my leaders saying that, yeah, the first thing that anybody gets excited when they launch is, oh, it looks great, I want to launch this, I want to launch this to all to all the users. That's not how you do it, you have to be patient, right. So that's one takeaway, definitely that people should take as part of this presentation.
I would say, last two things, to generalize to any geography or any nation or all of your markets, you should ramp your geos one by one. If you're doing a geo based store, even if you are testing, even if you do a AB test in a certain store, you do a small scale test, or a switchback test, you run it in a certain market, you actually scale it to the state, and then you scale it to the next state, and then you scale it to the next state, and then you build it up slowly, as long as you have confidence that the results are significant, don't just like try it here, and then just do a big bang launch. Of course, if you're seeing results of the order of 20, 30% improvement, which is just something that you have never seen. It's possible sometimes that happens. It's something that you've never seen and you never expected at all because something clicked with your users with the new experience that you launched, then you want to recoup that opportunity quickly, then you want to launch faster. But even then just for stability and the fact that your system could actually handle the scale as you bring more and more users into that feature. Be patient. Launch it. Ramp it up over a period of time, ramp it geo by geo and go a little slow.
And again, I think the Goldilocks period kind of ties into the last bullet point. Make sure that you run in a period of time that will generalize. Generally speaking, don't try to test it on Christmas, don't try to test it on New Year's Eve, like those are generally outlier periods for everybody in the world. So don't run tests around that and expect that to scale to in January or February. That's just not how the world works. So, insulate yourself against these types of things. Don't have novelty bias, don't run it short, don't run it too long. So that it starts crossing over into weird time periods, and ramp slowly. Be patient, take a very sound business decision. Finally, I will end by saying, there is a book that I've linked here, I get no credit for linking the book, but this is by two Nobel Prize Laureates, both of them are economists. And that is a book that talks about really testing in the real world, from an econometric point of view. It talks about techniques like difference in differences, etc. It talks about some statistical tests, testing as well, AB testing as well. But it gives you a layer of very gentle introduction to different case studies, different examples of how these kinds of techniques have been used. That's what I would suggest.
Gavin Bryant
For sure, thank you so much for your time today. Really appreciate it. There's so many insights for our audience to unpack. It's been great chatting to you today.
Vishal Kapoor
Thank you, Gavin, I appreciate you having me on the podcast. This was a fantastic conversation. Thank you for your thoughtful questions. And I will just tell the audience, if you still have any questions, or any concepts that you want to run by me, feel free to connect with me on LinkedIn. Gavin, are there comments on the website where they can leave comments or if they wanted to get in touch with me, are there any pointers there?
Gavin Bryant
No comments on the website, so best to contact you via LinkedIn.
Vishal Kapoor
Contact me via LinkedIn. That sounds great. Thanks again, Gavin for having me. And, looking forward to more conversations in the future. Thank you so much.
Gavin Bryant
Thanks, Vishal. Great chatting.
Highlights
ANALYSIS TECHNIQUE #2 - Synthetic Control - (PRO’s) more robust than comparing different geographies (CON’s) cannot account for external marketplace effects for the variant
TESTING TECHNIQUE #3 - Marketplace A/B Testing - (PRO’s) captures true change through attribution of cause and effect (CON’s) issues with bias and validity of results if the variant interferes with the control
ANALYSIS TECHNIQUE #3 - Statistical Significance - (PRO’s) enables product leaders to determine if experimentation results are reliable, or just variance and noise (CON’s) time taken to perform post-analysis to determine statistical significance and specialist resources required
It’s very difficult to use models or scenario modelling to forecast the outcomes of an intervention (product change, marketing campaign or algorithm change) on real-life marketplace outcomes - predictions and modelling can only take you so far
Regardless of how big the bet is, we always need to start small. The bigger the bet, the bigger the risk. If you take a big swing and miss hard, it is an even bigger business impact. Moonshots can cost a lot. You need to be even more surgical in your approach by conducting small scale experiments
The bigger the bet, the more confident you need to be through running lots of experiments to validate your hypotheses. Launching a big bet has impact on a brand. Big bets can be potentially be irreversible, being detrimental to a business if you make a bad swing
The biggest issue with marketplace experimentation in a small economy is statistical interference - the variant unexpectedly interferes with the control. This can set off a vicious cycle of events. In practice, this is virtually impossible to avoid
For example, if you perform an experiment for an online bookseller that elevates the rankings and popularity of Book A, it starts to decrease and cannibalise the sales of Book B. This creates a cycle - the popularity and metrics for Book A continue to increase while the sales metrics for Book B are negatively impacted
TESTING TECHNIQUE #4 - Switchback Tests - (PRO’s) captures true change, while avoiding interference (CON’s) tests are complex to setup and analyse
Conduct tests for a “Goldilocks Period” - not too short, and not too long. Test duration should be for at least one full business cycle. Avoid cutting test duration short
Think about the duality of Tests versus Optimisation. Tests help you to discover which is the best option. Optimisations help you to constantly find the best value
BE AWARE OF STATISTICAL INTERFERENCE AND METRICS DISTORTION
In this episode we discuss:
Analysis Techniques: performing synthetic controls
Testing Techniques: A/B Testing for marketplaces
Analysis Techniques: statistical significance
Efficacy for marketplace modelling and scenario modelling
Why forecasting marketplace performance is challenging
How to test your big bets
How marketplace experimentation can set off a vicious cycle
Testing Techniques: Switchback (Time-Split) tests
Key takeaways and tips
Episode Resources
Slides - Marketplace Experimentation: Zero to One
Book - John Doerr - Measure What Matters
Book - Causal Inference: The Mixtape
Lyft - Experimentation in a Ridesharing Marketplace
Difference-in-Difference in The Time of Cholera
Difference in Difference - Card & Krueger - New Jersey Minimum Wage
Success starts now.
Beat The Odds newsletter is jam packed with 100% practical lessons, strategies, and tips from world-leading experts in Experimentation, Innovation and Product Design.
So, join the hundreds of people who Beat The Odds every month with our help.