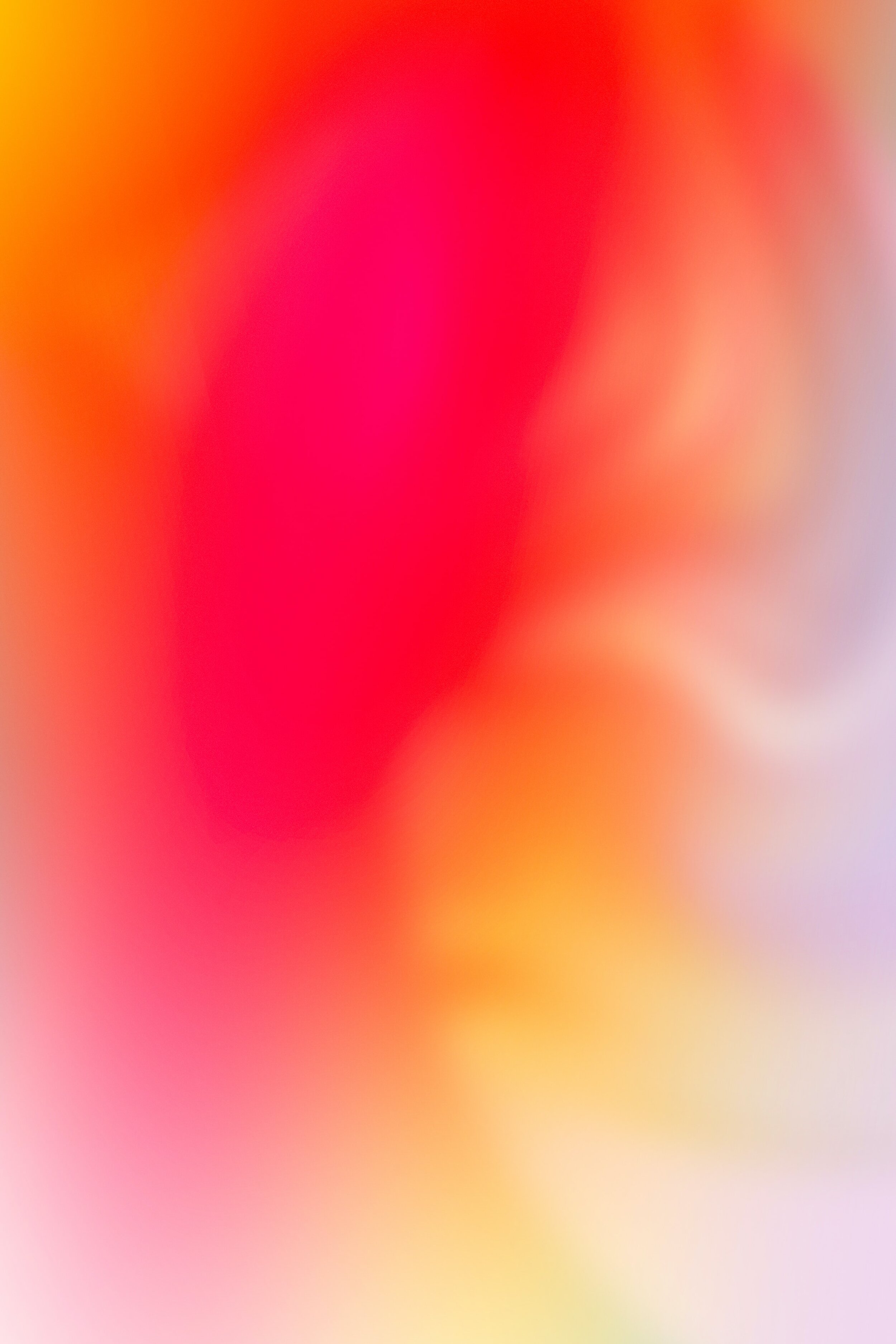
August 26 2022 • Episode 012
Vishal Kapoor - Marketplace Experimentation: From Zero To One (Part 1)
“The biggest problem with experimentation in multi-sided networks is interference. Your experiments can influence supply and demand in the marketplace. Experiment treatments affect not only the targeted users, but the entire marketplace. Test results can be biased based on true marketplace outcomes.
Vishal Kapoor is Director of Product at Shipt. Shipt, owned by Target Corporation, is a marketplace that facilitates delivery of groceries, home products and electronics. With more than 300,000 Shipt shoppers delivering same-day customer orders across the United States, Shipt was recently valued at $15B, with revenues of more than $1B per annum.
He is a highly experienced senior product leader, with deep expertise spanning Marketplaces, Transportation, Advertising, Search, Messaging, Gaming and Retail Industries. Vishal has built, launched, and scaled next-generation consumer products that have generated billions in revenue.
Prior to Shipt, he was Lead Product Manager, Marketplace Pricing and Intelligence at Lyft and led growth of Words With Friends at Zynga. Vishal has also held roles at Amazon, Microsoft, and Yahoo focussed on Advertising and Platforms.
Get the transcript
Episode 012 - Vishal Kapoor - Marketplace Experimentation: Zero to One (Part One)
Gavin Bryant 00:03
Hello and welcome to the "Experimentation Masters" Podcast. Today I would like to welcome Vishal Kapoor to the show. Vishal is director of product at Shipt. A high growth delivery marketplace that facilitates same day grocery deliveries. More than 300,000 Shipt shoppers deliver customer orders across the United States, where Shipt valued at $15 billion, with revenues of more than 1 billion per annum. Prior to Shipt, Vishal was lead product manager, marketplace pricing and intelligence at Lyft, and also led growth of Words with Friends at Zynga. Vishal has also held roles at Amazon, Microsoft, and Yahoo. In this episode, we're going to discuss marketplace experimentation, from zero to one. Welcome to the show, Vishal.
Vishal Kapoor 01:02
Thanks, Gavin. I'm very excited to be here.
Gavin Bryant 01:05
Now, a little bit of preamble for our audience. In this episode of the podcast, I'm going to try something a little bit different. This episode will feel more like a hybrid podcast/webinar. So think of it as an experiment on the Experimentation Masters Podcast, if you like. As always, I welcome your feedback on any of the episodes. We felt that this format would maximize value for the audience better as we'll be stepping through a practical experimentation scenario today. Vishal has kindly prepared some slides to accompany our discussion. To access the slides, head over to the "First principles'" website at firstprinciples.ventures, then podcast and Vishal Kapoor episode. I'll also be posting this episode on YouTube, you can access the episode on the First Principles Experimentation channel. Vishal, over to you.
Vishal Kapoor 02:06
Thank you so much. Again, thanks for having me. Let me start sharing my screen, and then we can take it from there. Can you see my screen, Gavin?
Gavin Bryant 02:20
Okay. I've got it.
Vishal Kapoor 02:21
Sounds great. So once again, thanks for having me. Before we get into the podcast and the content itself, I wanted to just give a quick introduction of why are we talking about marketplace experimentation from zero to one. This is my second attempt that at such a topic. There is another topic that I have spoken to in... That I've given public discourses on, which is called surge pricing zero to one. So at my company, I am responsible for products that actually price our offers, price the orders that are coming in for our shoppers. Shipt is, by way of introduction, Shipt is a three-sided marketplace, grocery delivery marketplace where customers are placing the orders. The orders that are being shopped from a retailer or a grocery store, and finally the shoppers are the ones who are actually fulfilling the orders. So one part of a big part of that marketplace of clearing the orders or having the orders fulfilled is how do we price the orders? So one series of talk that I've given before is... one topic is on surge pricing zero to one, how you build a surge pricing, a dynamic pricing system in a real time marketplace. This is the second in the series. It's called marketplace experimentation from zero to one. And I hope to continue giving such kind of talks in the future as well. So thanks for having me again.
Alright, so quickly just to introduce myself, Gavin, thanks again for recapping. I am currently a director of product at Shipt. I am an engineer turned product manager with my pedigree in different technology companies, large and small. I have spoken at different public forums, such as Microsoft, Carnegie Mellon, few conferences, and my LinkedIn is up there for anybody who wants to connect with me and ask me any questions. Follow an open door, open LinkedIn policy, please connect with me, feel free to ask me any questions. Alright, so loosely, this is what we are going to run through today. It's a lot but what my goal behind this talk is to cover, as Gavin mentioned, walk through a practical example of what a company might be trying to do and explore different techniques, different ways of looking at data running an experiment. What kind of different avenues do we have available? And these are some of the techniques that are used that some of the best tech companies in the world today. So what are some of these techniques available? We will start talking about why even talk about marketplace experimentation? What's the point? We will talk about, what are the goals of a business or a company doing experimentation? What are the business goals behind having an experimentation mindset? We're going to talk about some practical challenges and constraints. Specifically, when you talk about experimenting for marketplaces versus other scenarios, then we will go into a test scenario. We'll talk about different testing and analysis techniques. We'll go through it as we go through the deck. Finally, we will talk about what is the difference between what we call as an experiment or a test versus an optimization system? And what are some of the techniques that we use for optimization versus testing? And then finally, I will leave you guys with some final thoughts and key takeaways, of what should you take away from this entire deck? What should you walk away with if you are looking to experiment in marketplaces?
Alright, so let's start with why give a talk about experimentation in the first place. So testing and experimentation today is the primary technique for growing, either growing a business, or deepening the business, meaning, really improving your top line of how users come in, how they stick, how many users how they experience the product? As well as making them stick longer and longer and longer, improving your engagement. This is the way in which you may have heard of roles in product, for example growth product manager, or revenue product manager, or these kind of, or ads product manager. The primary skill set that a lot of these PMS use, including the PMS including me, I learned this as I moved over from engineering to being a PM. The primary thing that I learned, the most important skill set that I learned was to run experiments. How to experiment? How to think critically and analytically about different ideas, and to grow the business? It is a critical skill to master for technologists. But unfortunately, it remains somewhat arcane, and it remains limited only to people who have skills in math or computer science or statistics. My goal here is to demystify marketplace experimentation and make it more accessible to broader audiences, to more non-technical audiences. I don't have a lot of math in the deck. It's more about walking through a very practical, lucid example, and walking through what are the pitfalls of doing this versus that? How should you think about experimentation, from somebody who's not a mathematician, or a computer scientist, or a statistician, for example? So really, for business leaders, for business managers. That's where the top is oriented.
Gavin Bryant 08:36
Vishal, I've got a couple of quick questions at this point. First one, philosophically, I've previously heard you suggest the PM’s need to be more like a hunter than a farmer. Could you explain to the audience about that, please?
Vishal Kapoor 08:53
Yeah, multiple people have taken that quote, and have asked me about it. I think one part where that comes from is, for me, hunting is a skill where you are able to find diamonds in a coal mine, right. You're able to go and find jewels in the rough, you're able to go and see. There are a lot of different things you need to do as a product manager, to actually make a product shift. There's so many things that you need to do. You have to be able to not only just build the product, not only just communicate what you're trying to build, but make sure that it works fine, make sure that you are communicating outside with your audience, you're managing the change in the marketplace. You work with your communications teams, you work with your finance teams, you work with any legal challenges. There are so many things that a product manager needs to do to actually get something done. But when I mean that don't be a farmer and be a hunter. For me, it really starts from what is the idea that you're going after? Which is try to find and try to come up with a good justification of why should you... and come up with a data driven justification really? Or why should you try to do A versus B? Because there is always an opportunity cost if you did not do B, and if you chose to do A, there is always a time opportunity cost, right? Because you don't get that time back. Time is one fixed commodity for everybody in their life. So why should you think about this versus that? And I think that's a good question, Gavin. Because this experimentation is really the way to quickly validate.
Sometimes you don't have data, sometimes you don't have a strong hypothesis, sometimes you don't have intuition on whether you should be A versus B. And the real goal of experimentation, at least in big tech companies, is to really just do that and find that very quickly, in a couple of weeks. Generally, that's what you're trying to do. And, as you will see, a lot of tech companies, what they'll do is they do very incremental product updates, very incremental launches. I was reading a book yesterday about OKRs, and about how OKRs were implemented at Google. There's a famous book called "Measure What Matters", by John Doerr. And they talked about how they made YouTube as... they had a Northstar at YouTube, which is a billion views per day, which at the time seemed a big moonshot, when YouTube was coming up. And they talk about how they surgically did experiment after experiment, after experiment after experiment. Little things like does the like button work? Like, what content should we recommend after one video? Should we recommend anything, all of these things? Little things are experiments, small scale experiments, you try very quickly, really fast. You make a confident justification, whether A works versus B, and then you decide to launch. It's a decision making framework, essentially. You decide to launch A versus launch B. So in that context, the question that you asked, that is what I think is a critical skill for hunting. One thing is you need to be analytical besides the problem. But second is, if you don't know how to do it, you need to be able to experiment to find out how can you quickly get some data run a small scale test? Get some data to be able to say whether A is better than B.
Gavin Bryant 12:21
So the good PMS, the skilled PMS, they're very good at identifying opportunities, innovations, customer problems to solve, and then performing experiments to support their product intuition.
Vishal Kapoor 12:36
That's exactly right. Either that, either you know the problem. Usually, it starts with intuition, because you work on a product and you are intimately familiar with what the product does. You start with a feeling but many times we come upon ideas even internally at the company, where it's not clear whether it will go one way or the other. So then let's test it, right. Let's launch a small scale test, let's find out very surgical. What is the key idea we are trying to test? Just focus on that one idea, run it for two weeks, run for a couple of weeks, get some data. And we will look at some of the techniques that we use actually in this deck, and that's how we make decisions.
Gavin Bryant 13:13
Do you think that with product intuition that if we're not performing experiments constantly to update our mental models, and our intuition, that gaps then become evident in our product intuition? Have you experienced that?
Vishal Kapoor 13:33
Unfortunately, yes. I would I would answer that in two ways, right? One way is that... I would answer that in two ways. Sometimes, especially for very fledgling companies, very small companies where you're really trying to find product market fit, you have a product, you've just launched it, I would even call that whole experience of finding product market fit as one big experiment, if you will. Either it works or it doesn't, right. And it fails or it doesn't. But sometimes there are cases where you just cannot experiment, like for example, if you came up with the idea, and this is something called a split test, which is also technically called a test, but it's not technically an experiment where, let's say, if you were completely revamping your app, with a new version of the app, now, you wouldn't truly know you could test some things that go into that app into the old app, but you wouldn't truly know how people are perceiving the new app until the rubber hits the road, right? So there are some things where you have to really just try it, and launch it and see where at scale, basically what happens, but most of the times those cases are very rare. Most of the times what you do is you are able to make incremental changes to come to these decisions incrementally and then combine all the decisions into one launch and then launch that.
So I would say yes, I will. We see that unfortunately, in companies, there are companies, I've been in companies. And I think one of your previous talks talked about this as well. Not every company has the culture of experimentation. But I can tell you from experience, that the companies that have a culture of experimentation are leaps and bounds ahead of the companies that do not because there is no subjectivity, everybody is aligned. It's not just a culture of experimentation. It's a culture of being rooted in data, and making confident decisions, confident business decisions, based on data, not making false positive decisions, when you see something that is not reliable, not making false negative decisions. If something says no, but it's not true, not making a business decision on that and staying your course, these things only come when you develop some level of experimentation, in my opinion.
Gavin Bryant 15:48
Thanks, Vishal.
Vishal Kapoor 15:49
Awesome, so these are great. I'll keep going, cover some of these, Gavin, as we mentioned, as we go. And you know, that's a great [Unintelligible 15:59] into this. Why experiment? Why does a business care about experimentation, right? I mean, anything is costly, it's a skill. And building a skill is costly, and it takes time and all of that. So why even bother? We will actually start with... Let's get down to some definitions here, to kind of start bringing this data-oriented... What does it mean to actually experiment? So really what you're trying to do as a business, right. You're trying to, when you launch something, you want to measure causal inference. What that means is I did this, a feature was launched, therefore this happened, right? We launched this, therefore our users increased, or we reduced price, therefore our sales went up, or something else, like we did this, therefore that happened. That decision making that the cause is what led to the effect is actually not a very easy decision, its not very easy to actually draw. If you go down, and we'll look at that as we go, it's not a very straight line to draw in many scenarios, so let's start there. Let's talk about what does the business really want?
So first of all, let's define causal inference. Causal Inference means simply some action A was taken, and there was an effect B because of that action. So A causes B. Now, let's take a simple example from the real world. If someone plays the piano, I will hear music. Let's say, I'm in the same room, and somebody's playing the piano, I hear the music, that's straight cause and effect. One of the pitfalls that happens, as a business, is that sometimes people mistake correlation with causation, which means that something has happened. But that doesn't necessarily mean... That means B happened, an effect happened, but maybe that was because of A. That doesn't mean it was definitively because of A, and that is something that businesses want to avoid. Our users increase, but did they increase because of a change we made, or was that something else externally that happened? Maybe a competitor pulled out of the marketplace, maybe that's why the users had nobody else to go, so therefore the increase. These kinds of things, like how do you actually unbiased yourself is something that is a big pitfall that happens in business decision making. So let's take a simple, let's continue our example. If I am hearing music, somebody may or may not be playing the piano, maybe. It doesn't always mean... like our cause is somebody's playing the piano, our effect is I'm hearing music. I may be hearing music, because I'm hearing it off of YouTube, for example of my computer that doesn't mean somebody's playing the piano. So that's what I mean by correlation is not causation.
The other problems that also happen in decision making is you want to avoid false positives, which means that the effect happened because of it. So here, for example, if I'm hearing music, someone must be playing the piano. That's also not true, right? I may be, again as I said, I may be hearing music because of other reasons. It doesn't mean somebody has to play the piano, I could be doing it because of something else. That's a false positive, which is I am seeing the effect therefore that cause must be true. That is a false positive. And finally, a false negative. I'm hearing music, but nobody's playing the piano, which is B but not A, which truly means that... A false negative really is that I'm not hearing music, but that means that nobody's playing the piano. I think I need to revise that, I think I have a typo there. I'm not hearing music, but no one is playing the piano, but that's also not true. I may not be in the same room where somebody's playing the piano, therefore I'm not hearing music, I may be outside, I may be in a different room. That's a false negative. Always assuming that I'm not seeing an effect is in the absence of a cause. That's basically a false negative, and we'll see some of these as we go. So there is a small typo it should say I'm not hearing music, but no one is playing a piano. That's a false negative.
Gavin Bryant 20:05
So in your experience with product teams, do you think that when product teams mistake correlation for causation, they hear music, but there's no one playing the piano? Is that one of the biggest mistakes?
Vishal Kapoor 20:23
That is a fairly common mistake. And I will use another term for that. There's a term called confirmation bias, which is, you see what you want to see, regardless of what the data is telling you. And that usually is an indicator that you are trying to find correlation and data where there is no strict causality. That confirmation bias is an outcome of the fact that you can see A leads to B, but you don't know if A has strictly caused B. You don't know that. That happens fairly often.
Gavin Bryant 20:56
All music is associated with being a piano.
Vishal Kapoor 21:00
Exactly right. That's not always true. That's just not always true. So what are we trying to do as business leaders, right, and when we run a business, the goals of experimentation is to avoid correlation. We need strong causation, strong causality between the cause and an effect with very high confidence, and very low likelihood of making a business error, which is you don't want to have false positives, you don't want to have false negatives, right. That's essentially the goal. We want to have strong causality, we want to have high confidence that the causality is strong. We launched something, therefore an effect happened. We reduce prices, therefore, I will say sales went up, and it's not always easy. Again, as coming back to what you were saying, our sales went up is not always, because we actually dropped prices, it could be because our competitors went out of business, therefore our sales went up, there could be so many other things. And that is the key behind building a business, statistically or statistically sound principles on experimentation principles. How do we know when we did an action, that there was an effect because of the action we took, removing all the other biases that happen outside? That's really the question. Wonderful. Okay.
So now let's talk about some of the practical challenges and constraints, in building a business. Let's again, take it one level down, I promise, I will not bring math into this, but there will be some definitions. And I think it's only appropriate that when you talk about experimentation, any literature that you read, will use some terminology of running an experiment, it's only appropriate that we some variation of what that terminology means. So this is just very, very high level. So let's talk about three things. First of all, let's talk about what is a variant. Very simply speaking, a variant is a set of points for which there is a change. So like in continuing our previous example, let's say there is Jill, who is within the earshot. Here's the piano when Jack is playing the piano, right? So Jill, for example, in this case, could be a user who is part of a variant who happens to be in the room. And you know, the piano is played, so she happens to be the one who hears the piano. Now control is the set of data points for which nothing has changed, no change, you know, status quo. That's what we call as control. And variant is, when we do launch a new version of something we typically run on the audience like Jill, for example, that's our variant audience where we are testing that feature. Our control, for example, is Emily, who is not within earshot. She doesn't hear the piano, no change. That's your status quo, right? So Jill may be in the room, Emily may be in the room, either of them could be in the room. And that's what a randomized control test is.
A randomized control test is a test where the variant data points are really randomly chosen. So there is no bias between choosing Jill or Emily, for example, either of them could end up in the room. A randomized control test is where you get to control who gets to be in the room. Or maybe in another way of saying it is where is the piano, is the piano in the room where Jill is or is the piano in the room where Emily is. Let's say we control the piano or Jack controls where the piano is located. That is the test and the audience is your Jill and Emily. So a randomized control is sometimes it could be with Jill, sometimes it could be could be with Emily. And then the cause is Jack is playing the piano, and the effect is whether Jill hears it or Emily hears it. S randomized control is that there is no selection bias. Generally, you're trying to select out of a given set of data point, your sample. You're trying to select the data points purely random. That's the goal. You're trying to select them in a very unbiased way. And look at what happens on this set versus that. Generally speaking, that's what you're trying to do. So in this case, as I said, either Jill or Emily could’ve been within the earshot objecting to piano. So that's what a randomized control is, both of them are equally likely.
So with that, let's see some of the challenges. Now, marketplaces are multi-sided networks, right? Usually there is at least two sides to marketplace. You can take any marketplace, talk about Uber, there's a driver, there is a rider. Same with Lyft. Talk about Instacart is a North American grocery provider, Shipt is a North American grocery delivery provider. There are three sides to it, right? There's your customers, as I was mentioning, before your customers placing the order the retailer where the order is shopped from, and then the shopper actually going and fulfilling the order. So there are three sides to it. Think about some other networks. Think about Google AdWords, there are advertisers who are really the demand side, because they are placing ads, and the supply side in this case is are the eyeballs are the consumers, because they want to have the consumers do some action, like fulfill a demand that they are actually looking either watch a video, click a video, do something, take an action.
So there are two sides there as well. So multi-sided networks, like marketplaces, the biggest problem with testing in multi-sided networks is that they have something called interference. We'll see an example of this as we go. But really what that means at the high level is when you change demand for a variant, right, we talked about variants and control, when you change demand for a variant that can actually affect supply in that variant, which can actually affect supply and control, which can actually affect demand and control, it can actually have a see-saw effect, and that is really what a what a network interferences. We see this with an example as we go. Because of the see-saw effect, what happens is that the metrics between the two sides, when you're doing an experiment, they basically get inflated or deflated, one goes up more than it should, the other goes down more than it should. Typically it is one to one, but it doesn't necessarily have to be. It depends on if your distribution is skewed, how you select your audiences, etc, etc. Typically, it is one to one like, if your sales in one of them are doubling, in one or in the variant are doubling due to a price reduction, maybe in the control, you will see that everybody is going there, you're losing supply because of that, your books are running out something like that, and because of that your sales in the control are now plummeting. So there is some sort of network interfere, we'll see that example as we go.
So that is what I mean by if sales was your metric that you are measuring out of an experiment, you would see sales go up in the variant, but guess what, indirectly you are unexpectedly affecting your control where sales are going down, so it's looking artificially inflated more than it normally would be. If you had the same effect everywhere, it will not be that it will not be a double increase, it would be like somewhere in the middle. But it's difficult to kind of analyze that, it's difficult to discount for network interference and all of that, like in complex marketplace, it's just very difficult. And we'll see that. That's basically what I said there, especially when you're running many concurrent experiments with many different business lines across many... One user might be part of sometimes 5, 10, 20, 50 experiments. At this, they don't know, they might be part of many different experiments running at the same time, it could be very difficult to analyze the effect of network interference, because in your experiments, the variant actually impacted control, which again, went back and impacted variant. And finally, I think the biggest thing is, with any experimentation or just marketplace, do not confuse correlation with causation. The question you asked Gavin, do not confuse correlation with causation. Be very confident that you don't have false positives or false negatives, and you need to have a strong confidence and there are ways of statistically measuring confidence. You need to have a strong confidence that A actually cause B, and B did not happen. It happened because A happened that reverse causality is something here.
Gavin Bryant 29:08
So Vishal, do you think it's very fair to say then, that marketplace experimentation can be very hard due to the highly fluid and interconnected nature of the marketplace? Effectively, A product marketplace is like its own little economy, where it's very easy to either positively or negatively impact supply and demand through the experiments. So it's really something that experimenters need to be highly mindful of and cautious of.
Vishal Kapoor 29:44
Yeah, I think you summarize it in a very good way. It is like a mini economy in and of itself. Except in a real world economy, there are so many variables that, and you don't have control over all the variables all the time. Here, because you're running a business, it's a closed economy, you have control. And usually when you design an experiment, usually you're trying to affect one, two or three variables only at once. You're not trying to affect entire business, because your experiment is contained to a certain segment of the population. But you are right, even in that, even when you are very surgical, and the experiment is contained, one side can impact the other. It's pretty complex. In fact, I would say that a lot of new age experimentation techniques now are coming out of companies like Lyft, Uber, Instacart. All these new real world marketplaces, you can go to their websites, and you see data scientists publish blogs upon blogs upon blogs, about the new ways of running experiments. These techniques have existed, by the way for a long time, but they were never used in these complex, because the complexity of the marketplaces are so high, you have to actually bring a lot of different techniques into place to actually be able to make a sound confident business decision. That's absolutely true.
All right. Now we'll get into the meat of it. The art and science behind experimentation. And let's start by painting a picture of... let's start with a simple test scenario, or toy scenario, and let's go from there. So for the rest of the presentation, I want the audience to imagine that there is a bookseller, we have a bookseller. And the bookseller sells different books from different publishers, in roughly 100 physical locations in three states in the US. So in New York, in California, and in Washington. So they have 100 stores each in New York and California, Washington. 100 is just a number, but just to keep things equal. In New York, California, and Washington, they have 100 stores, they have 300 stores across these three states. And they are selling books, it's a physical location. I specifically chose an offline experiment versus an online, I was hoping that this would become more relatable. You should be able to take the learnings from an offline experiment, and actually translate them into an online world. But I just wanted to make a point, even in an offline world, how these kinds of techniques, some of the biases network interference that we talked about, how that can show up even in an offline mode? I wanted to motivate the example, because I feel like it will be more relatable with a simple bookseller as an example. So start here, and then we can see how it goes from here.
So now for this bookseller, just assume some very simple business model, a very simple framework, every book that they sell, they sell many books, every book is sold for $10, same price for all books. Also, let's assume that in a week, throughout the week, they sell about 200,000 books. And just for the sake of explaining the different techniques a little bit, let's assume that their biggest market is New York, where they sell about 100,000 books out of the 200,000 weekly. And then the other two markets, it's 60, 40 split. So 60,000 in California, 40,000 in Washington. Very simple model. And generally, because they're selling 10 books, right, sorry 10 bucks per book, and it's 200,000 books, the weekly revenues $2 million. And a very simple goal. Now this business, this bookseller, they have a business goal, where they want to simply increase the revenue, right? They want to go from 2 million upwards. That's it. Now, this is another word that comes from the parlance of experimentation, which is hypothesis testing. Hypothesis is generally you have an idea, you have a theory, and you basically want to test that theory through an experiment and validate whether or not that hypothesis is true or false. Really, generally, that's what you're trying to do. And their hypothesis, their guess is that if they reduced the book prices from $10 to something lower, that would increase sales, the number of books that are sold, you know, will go up from 40k, 60k, 100k, from 200k upwards, and that will actually increase the revenue. So the rise in sales should offset the drop in price. That's really the second layer behind the hypothesis. The rise in sales should actually offset the drop in price, and it should effectively raise the $2 million to a higher number, over $2 million per day. So far, so good. All right. Very simple.
I'm trying to be... this can get very complex. So let's, yeah, hold your horses now. Here we go. So let's talk about some testing and analysis techniques now. All right. So the very first thing that any business, any company, the way we start, is the first thing that they will do in the variant, which is again just to remind everybody is the set of data points where a change was made, and control is the set have data points where no change was made. So in the variant, their variant is they do a time based test. And this is also called a pre and post, pre the change and post a change. So what they say is, let's drop the price of the book to $9 on eighth of May, right. So they pick a date, they say that the book prices will be dropped to $9 on 8th of May. We will look at data before and data after pre and post the price drop, what happens? So the results are versus the control period in the previous week, which is 51 to 57, and we follow the MMDD system yet. So that's month and date, for people who are not familiar with that system. So that's May 1 to May 7. The sales in Washington are higher in the next week, but between five eight to five. Sorry, the sales rise in Washington in the following week. So versus the control, versus the previous week, the sales rise in Washington in the next week, 58 to 514, but they actually fall in California. Now remember, the hypothesis was if you drop prices, the sales should go up and the revenue should go up. In this case, one of them shows that the sales have gone up, Washington sales have gone up, which is the smallest metro, which is only 40k, but California is the middle metro with 60k, the middle state with 60k, and there the sales are actually falling. Why would that happen? Right? Let's talk about that. What can happen? A lot of things can happen.
One of the possibilities is that there was some external interference, meaning some bad weather happened in California, there was a wildfire, there was a storm, there was lifelines, something like that happened, because of which maybe there was some civil unrest, for example, something like that happened in the post period, because of which the sales actually dropped in California. That was not the intention, this is something external happened, completely external to the experiment. And we will look at this, this is just to paint an example of what are the pitfalls in business decision making, ultimately, that's why we are going with this right. So this is a pitfall, if you only looked at Washington, and if you only tested in one case, and you only analyze Washington results, you would say this works, and you would just launch that nationwide, which would probably be not a sound business decision, because you haven't actually looked at California and New York in this example, you know, that can happen. So that's clearly not bulletproof, right? So we need something better, let's just say that. But here's the interesting thing, right? For a business, really, this is the blueprint of how to run a business, right? When you look at the stock market filings, Wall Street filings in the US, your quarterly reports, your annual reports, they will usually report growth metrics, revenue metrics, user metrics, ad revenue, this and that, quarter over quarter sales, year over year.
So again, coming back to what you're saying, if you think of the entire business as an experiment, in some sense that it's at that level, at a very high level, when you're dealing with billions of dollars, it is fair to say that the business is doing better over time, because it is a collection of so many small decisions. At that point, it is sound to say that, yes, the business is doing good, therefore investors want to invest in it, because they feel that their business is doing better, they have sound strategies, etc. At that level of granularity, at the highest level of granularity, it is probably fair to to measure a business and a business experiment to measure that using this kind of technique, using this test. But if you go down as we saw, if you go down, if you go to Washington, California example that we saw, that's usually not a good launch decision for launching a small feature for just dropping the price from $10 to 9, that's usually not a good decision, you just haven't looked at all the factors, it doesn't discount for all the external factors.
So I mean, the good news here is that it's very easy to analyze and understand that's the probe, right? Everybody can say, my sales last week versus my sales this week, that's very easy to understand for business, for anybody in the company. The problem is it is very difficult to attribute cause and effect, as we saw here, you don't know whether the sales rose or drop. Now looking at California, how can you guarantee that sales in Washington did not rise because there was something else that happened in Washington, you can't guarantee that right? Because something external could have happened. So it's not very sound, unfortunately. And as I was saying, it's not a very scientific way of doing things. So don't make... it's a good way of making business decisions for at a higher level at a very aggregate level, it's not a very scientific way of deciding whether this is the next feature to launch or not. So don't make small optimization or launch or incremental optimization decisions based on it. And that's generally in practice, use that on an aggregate on a directional basis. So that's our test one, right? I'm sure Gavin, you may have seen various places, as I said, very relatable, right? You see that in stock market filings or all businesses are doing overtime, etc. That's generally how people think about whether a business as an experiment is successful.
Gavin Bryant 40:07
Yes, I was just reflecting, and pre and post is the most heavily favored form of analysis in business, and the most readily used. However, there's never any thought of the upside and downside of using this approach and the nuance involved. So, to your point, it's not scientific and difficult to attribute cause and effect.
Vishal Kapoor 40:39
Not scientific. That's exactly right. All right. Let's try something else. Let's actually do this based on geographies, right? I mean, time doesn't work. Clearly, we saw that. So now let's do this. Let's only drop prices in Washington to $9, and everything else is the same, right? California and New York, no changes there. So now we should be able to see a lift in sales in Washington versus California, right? All other things being equal, we should be able to see a lift in total revenue, you can always normalize it. California was selling just to anchor everybody, California was selling 60,000 books per week, Washington was selling 40,000. And they were making a certain revenue, you can normalize by the number of sales. But you should say that, if your hypothesis was true, and you drop the price only in Washington by $9, your price per book, or your total sales, and your total revenue should actually go up, right, your total sales should go up. So that's what should happen. Now, what we see is, in this example, next week, from between May 8 to May 14, put in the following week. Now, sales rise in both Washington and California. This is not intended, California was never a variant. It was control, essentially, but sales rose there. Now, why would that happen? That's again, like it's this very scientific. How do we make decisions here?
Gavin Bryant 42:04
I've just got a quick question on Geo based testing. And in reference to marketplace experimentation, that what we've discussed is that randomization of experiments locally to supply and demand, it can introduce biases in experimentation results, and potentially overinflate experimentation findings. From your experience at Shipt and Lyft did you find that for a product change, to test a marketing campaign or an algorithm change, that you were then elevating experiments up to the level of city or state, and then comparing city or state to try and overcome that localized user bias?
Vishal Kapoor 42:57
That's a good question. We will cover an actual example of what is a sound technique for running it so forth. So first of all, I would say that running an experiment at a city or a state level is very costly. First of all, this is a very simplistic example. But imagine if this was literally, 200,000 was the only revenue that this bookseller was making, doing it at the state of Washington level is just extremely costly, because you're doing it across all the stores. So we said Washington was 40k, out of 200k, so that's 20% of your entire volume, you're actually rocking it by $1. That can be a very costly experiment to run. So you don't first of all, you don't try to run off just because you don't observe strong causality, in a certain in a small city or in a small state. That doesn't mean you go one level up and you start aggregating, it's costly to do it. You do go to some other techniques, you do that fall back to other techniques. And we will also see an example specifically from Lyft. To my second point, Gavin, where there is a case study of how Lyft actually tested pricing prime time, which is surge pricing, and how the network interference actually showed up and what technique did they actually use to get around it? So we'll talk about that. Geo is not that technique is what I'm alluding to. This still looks like it's kind of flawed, because as in this example, the sales rose in both cases. Now, how do you know what was the cause? And what was the effect in this case? So we will come back to that. Let's put a pin on that for now.
All right, so what could happen here? Well, in California, something like a major book released by some celebrity author in Los Angeles, it's Hollywood, somebody might have released a big book, some celebrity and that would have inflated sales in California, completely external to the experiment again, unfortunately. As you said, this is pretty common to test in one metro, on one market and compare it with a control market. Typically, it is a technique that is used in marketplaces. So the point that you are making is valid, that it is used, and it is easy to understand and analyze as well, right. You can normalize, as we said, not normalized the total revenue by Washington sales and California sales. It's very easy to understand and analyze. But unfortunately, in a lot of marketplaces, it does not scale from one to one to all markets, especially in geographical marketplaces. Because in a lot of these marketplaces, the effects are very hyper local. So what actually works in California, Washington during various factors, state laws, minimum wages, how you price things, this and that. Usually it's not every book across every state is priced at $10, so we have a hypothetical scenario. But even then, what worked in Washington and what worked in California should not and cannot be generally used as a proxy for all states and the entire country.
I will give you an example, where we had run an experiment in the past, without disclosing too much, we had run an experiment in one of our smaller markets, where we actually thought of launching it in nationwide scaling nationwide, we saw very good results in one of our smaller markets. That small market was actually, this was before my time, but I what I heard about the experiment, that's more market was a very healthy market, it had a very high number of shoppers, it had a very good supply demand balance ratio, things were getting claimed really fast and orders were getting claimed because there were enough shoppers etc. But the average supply or demand ratio, the average claim rate across the nation was not exactly according to it, although it was a small market, we went for size, we didn't want to, as I said, we didn't want to cause a big impact. So we went for size, and we went for the saline market. Unfortunately, the dynamics in the entire marketplace nationwide, were not the same as that market. And so when we did that, in that case, what we did was we did launch incrementally. So we started going from one market to the other, and we somewhere caught it in the middle, where we figured out that this is not something that is scaling, we didn't catch it. But you know, case in point is, if you are a smaller player, like you have only three states in this example, you don't have that many things to experiment with in the first place.
So how do you actually even do it? This is not the right way we caught it. But there is always a risk that in these kinds of marketplaces that generally marketplaces which are rooted in the real world, not on online marketplaces, or different online marketplaces, then you talk about things, because they scale globally. So then you're talking about whether an experiment in US will scale in China, or whether it will scale in the UK, it's the same problems apply more or less, but it's a little more homogenized, in offline marketplaces or real world marketplaces, it's there is more heterogeneity, there is more hyper local effects. So in that case, usually what happens is that what translates in a few markets, which are very similar to each other, may not translate to some other markets, which don't look like that. That happens a lot, unfortunately. But a lot of companies for lack of a statistical orientation and experimentation orientation, or that mindset, will resort to let's test in one in one market, because they understand markets, let's test in one market, let's go to the other, let's go to the third one. Unfortunately, again, drawing that causality and being able to say that with confidence that if this happened in Washington, it will also happen in California, if this happened in these two, it will happen nationwide.
The biggest tech companies, what they will do is they have such strong, there is a strong emphasis on having high confidence in that causality. And once you know with high confidence that Washington works, then you can just literally launch across the entire US without even trying to test it in another market, you can just go for it because you have very strong causality. That's how these companies with which have an experimentation mindset, that's how these companies are able to scale that fast. It takes time to build that culture, but once you have the confidence in the data, you don't have to think twice. You don't have to look over your shoulder. You did it once, you did it on a small a small set of data that you weren't biased in picking Washington or California or there was no hyper local effects just to take that as an example. You were confident that when you selected the metros or when you selected the audience, the variant to experiment on you need new this variant is representative of my entire population. So I can just skip, but this is not that use case. That's typically not true, because hyper local effects are very different.
Gavin Bryant 50:00
Yeah, I find that geography quite intriguing that I've run a lot of experiments here in Australia across the country. And in my experience, I've found that the eastern seaboard particularly behaves very similarly across Queensland, New South Wales, Victoria, the west of Australia operates like its own micro economy. And the remaining states and territories also behave independently and very different to the other states and territories. So making an assumption through an experiment on the Eastern Seaboard, and assuming that consumer behavior will apply a broad brush across the whole country is fundamentally flawed.
Vishal Kapoor 50:41
That doesn't work. Thank you for bringing that point, which is exactly my last word. This slide in this example, you would literally have to change. Test this in every single state independently. In which case you're not running a scalable experiment, you're just testing whether something works. And then it's scattershot pricing, right? You have some pricing here, you have some other pricing there. It's just all scattershot. It's not experimental. Thanks for being there. All right, so we can do better. So let's see, even with this, we can do something better. Let's see, if let's say that we did run that test, and it costed us some money. Can we actually salvage some of the data that we bought there? Let's start there. There is a analysis technique called difference in differences, or DID, the acronym is DID. What we're trying to really ask here is, can we get a true change in Washington, using California as a proxy? Okay, because sales rose in both Washington and California. Can we get to change in Washington using California as a proxy? There is an assumption that we will make...
The example I gave previously in the previous slide was some celebrity released a book in California, which is an outlier, which I understand, but if you assume there is a strong assumption, which is assumed that California and Washington have similar sales trends. Assume that nothing external had happened, in California, just for a moment, let's assume that without price changes, sales in California and Washington would have changed the same way, if you did not change, if you did not experiment. Let's say there is an organic increase in sales for whatever is in Washington, let's say it's the National Book month, somehow the post launch period falls into the National Book month, some event something like that happens, or maybe a celebrity author doesn't just release his book in California, they release it nationwide. So all markets get it, and the rate and sales are elevated across all of the markets in this example, so you would have to assume that they are similar trends. And in that case, if you did assume that, then a different model, what the essence of the model is that you can use California metrics to discount for changes in Washington, which means that the table at the bottom really just says, it leads to the insight on the left hand side, which is if you looked at the difference in sales in California, 40k, 60k, that's where we started. So between, in the pre-launch, the sales were 40k and 60k. In the post launch, the sales became 70k and 80k. So there is a rise in both sides, right? 60 goes to 80, 40 goes to 70. But what is the true difference in Washington, compared to what was in California? So the different really says that you take a difference in California, you do discount Washington for that, and then what is remaining is really the true change of your experiment. That is the reason why that was a true change of your variant. That's simply what different effects is, you basically take something with a parallel trend assumption with similar trends assumption, and you actually discount that to find out the true. Simple enough, right? Simple enough.
When you go and look at this in papers, it's pretty confusing, because there are a lot of online papers and the way they try to explain what different is. The key insight is, as I said, simple enough, without any variant in Washington without changing prices, it would have actually changed California, it would have seen the change that California sees. So just remove that difference from this difference. And then the remaining effect is basically what you would see in because of the change that you made. So that's one technique that is still a common, this is still a technique that is used in marketplaces, especially when you don't have a sophisticated level of permutation. It's better than nothing, right? So you have something to fall back on. All right. What are the good things about this? You can use this technique especially when you cannot run randomized control experiments. So again, Gavin, the point that you made was that companies are mini economies, companies are small economies, but the good thing is you control, people who run the company actually control the entire economy, have control over the entire economy, right. But then there is the real world where nobody has, it is truly random, right? The real world is random. And you don't really have control over all the variables that can change in the real world. And in that instance, when you cannot run an experiment, this technique is very heavily used. It's very heavily used to, for example, evaluate the impact of a statewide policy, when one state does something versus the other state. It's not like they're not going to select audiences to implement policy on one segment of the population versus the other, they do it statewide.
And using our Washington, California example, assume that instead of that dollar, the book dollars, it was some minimum wage, let's assume that, for example, right? Then you will actually have to fall back on something like this, to be able to say, what was the effect of changing minimum wages due to a policy change? Because you cannot really run a randomized control, where you can say, half of the population gets a different minimum wage versus the other, you can't do that. It's not a closed economy. It's in the real world. The problem here is in marketplaces, in controlled economies, similar trends are rare. You shouldn't assume that everything... Even in our example, we saw that something external could have happened, which would have thrown California sales off, it's not very common, similar trends are rare. So usually, you should use this in conjunction with other testing techniques. It's an analysis technique. It's not a test technique. It's an analysis technique. So you use geo, you use time-based or some other techniques that we will see coming forward. And then you use this as sort of like parsing out some of the unwanted effects or some of the external biases that would have happened without you doing anything to the market in the first place.
In fact, the thing that I mentioned, here are two couple of case studies, they are actually hyperlinks for the audience members will share the deck, and you're welcome to go and click on that, as you said, the deck will be shared, you can go and click the links. But one of them was exactly that, which was what was the impact of minimum wage on change on employment rate? In a couple of states in the US, there is a famous study that is that study is actually cited, as I said, that's from Wikipedia. It's actually a study from 1990s, started as a famous study for highlighting how difference in difference was actually used. In fact, the study comes from the 1800s that the first bullet, there was the impact of, there was a change in water providers to Indian companies, and that actually led to cholera outbreak. So this is what that study really shows in London that was in London, but that study shows is, without the change, how much would have cholera spread anyway, which is the same idea, with that change, then what was the impact of bringing that way? And bringing that new company with that change, what was the incremental effect? Or what was the alleviation that company did, either added or took away from cholera, there were three companies actually in our study. So that was basically that was a technique of using different which was unchanged. This was how much cholera progressed anyway, with these companies. This is how it actually impacted the rate of communism. It was corresponded in the 1800s. And as I said, we are learning things which are over a century old at this point, and multiplicity are starting to use these things.
Gavin Bryant 58:19
Read that study, it's very interesting that it completely flipped the thinking around cholera outbreak in London from being a virus that was airborne to waterborne. So a great piece of scientific analysis.
<< END OF FIRST EPISODE >>
Highlights
In the modern business, experimentation is the primary technique for growing a business, increasing customer engagement and deepening how users experience the product. Learning how to perform experiments is critical for modern technologists
Product Managers need to think more like a Hunter, than Farmer. Product Managers need to spend more time thinking about solving customer problems than project management. The data-driven Hunter is more valuable than the output driven Farmer
Good Product Managers are constantly performing experiments to update their product intuition and mental models, avoiding a Perception-Reality gap between what they think customers want, and what customers actually need
The primary goal of experimentation is to avoid correlation. We need to ensure strong causation between the cause and affect, with very high confidence, to ensure a low likelihood of making a business error
Marketplace experimentation is challenging due to the highly interconnected nature of multi-sided marketplaces. When performing experiments in a marketplace you are inherently altering marketplace equilibrium - changing test demand effects supply to the control group
Think of your online marketplace more like its own micro-economy
For example, if your experiment promotes a book to users in the treatment group, if users buy up copies of the book, it will mean less supply for users in the control group. Therefore, sales to the control group are impacted too
There is strong evidence of bias due to test-control interference in online marketplace experiments. Experiment interference can overstate or understate test treatment effects
TESTING TECHNIQUE #1 - Time Based Pre-Post Testing - (PRO’s) simple, easy to understand, common (CON’s) susceptible to interference, proving causation is difficult, not scientific, more suitable to top-line decision-making
TESTING TECHNIQUE #2 - Geography Based Testing - (PRO’s) easy to understand and analyse (CON’s) non-scalable, testing required in all markets
ANALYSIS TECHNIQUE - Difference in Differences - (In Practice) used in conjunction with other testing techniques (PRO’s) can estimate causation when controlled experiments aren’t possible (CON’s) trends and patterns can be rare in marketplaces
In this episode we discuss:
The goals and challenges of experimentation
Why Product Managers need to be more like a Hunter, not Farmer
Updating product intuition to avoid blind spots
The pitfalls with causal inference
Why you shouldn’t hear music if the piano’s not playing
The art and science of experimentation
Case study analysis - Increasing marketplace book sales
Testing Techniques: Time Based Pre-Post Testing
Testing Techniques: Geography Based Testing
Analysis Techniques: Difference-In-Differences
Episode Resources
Slides - Marketplace Experimentation: Zero to One
Book - John Doerr - Measure What Matters
Book - Causal Inference: The Mixtape
Lyft - Experimentation in a Ridesharing Marketplace
Difference-in-Difference in The Time of Cholera
Difference in Difference - Card & Krueger - New Jersey Minimum Wage
Success starts now.
Beat The Odds newsletter is jam packed with 100% practical lessons, strategies, and tips from world-leading experts in Experimentation, Innovation and Product Design.
So, join the hundreds of people who Beat The Odds every month with our help.