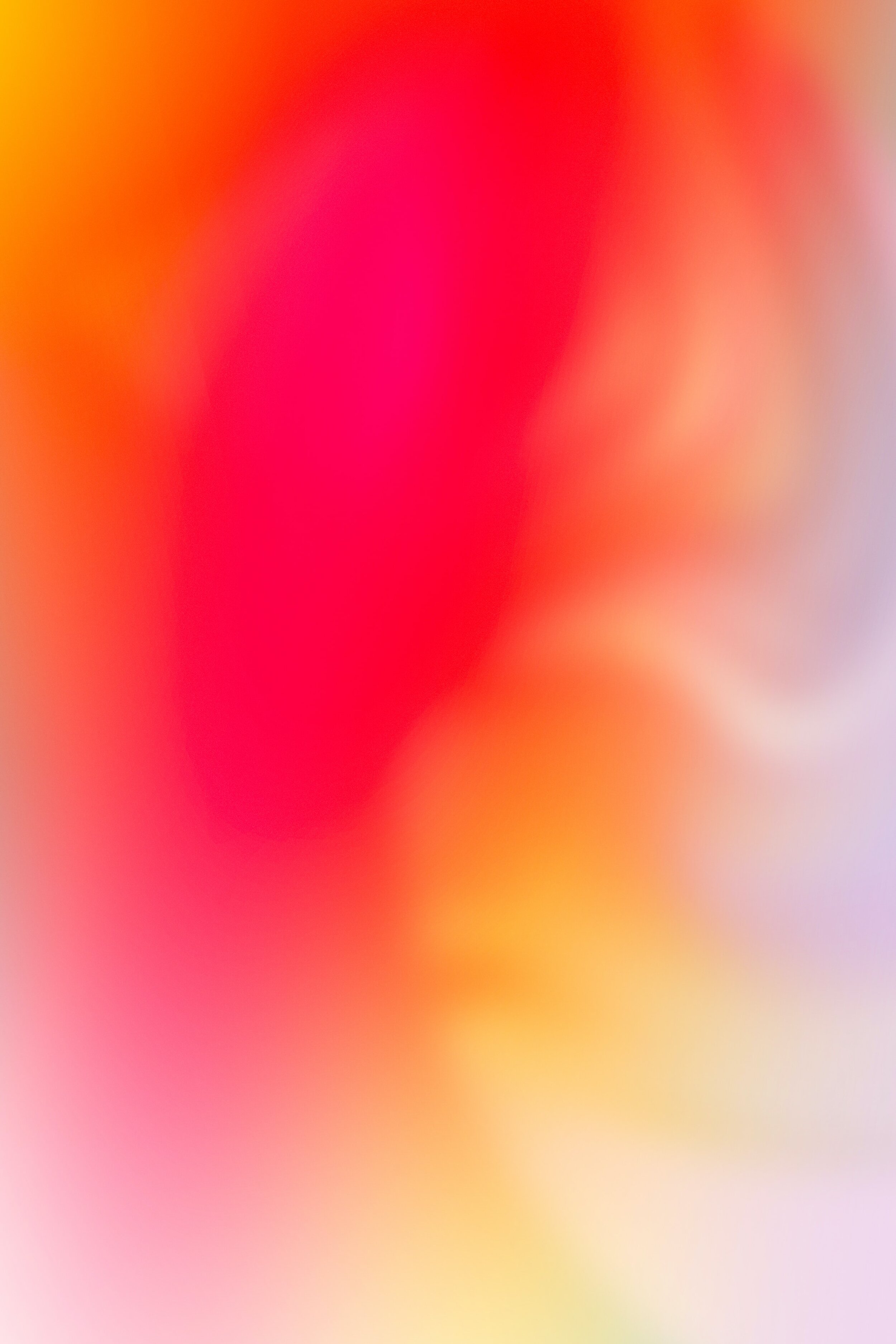
May 24 2022 • Episode 010
Rohan Katyal - Yelp: A Story Of Building An Experimentation Program
“Experimentation is not only about the technology, it’s about the people. Focus on the people and the cultural change. People are the key ingredient that make or break your experimentation program.”
Rohan is a Product Manager at Facebook (Meta) working in the New Product Experimentation team with a focus on helping creators monetise.
Before working in Facebook’s NPE team, he led growth for Messaging Monetisation on Messenger and Instagram. He also led growth of Click to WhatsApp ads to over 1M monthly advertisers.
Previously, Rohan was a PM at Yelp where he grew "Request-A-Quote" by 25% leading to more than $500M in services revenue. He was also responsible for building and scaling the Yelp experimentation program.
Rohan’s approach to democratising experimentation is the subject of a Harvard Business School case study.
Prior to Yelp, Rohan was a part of the new products team at Yahoo!. He also co-founded and spearheaded a 16 people non-profit called FindAWay focused on equitable access to education.
Get the transcript
Episode 010 - Rohan Katyal - Yelp: A Story of Building an Experimentation Program
Gavin Bryant 00:03
Hello and welcome to the Experimentation Masters Podcast. Today I would like to welcome Rohan Katyal to the show. Rohan is currently a Product Manager at Facebook Meta, working in the New Product Experimentation team with a focus on helping creators monetize. Prior to working at Meta, Rohan was a Product Manager at Yelp, where he grew request a quote by 25%, leading to more than 500 million in Services Revenue.
He was also responsible for building and scaling the Yelp Experimentation Program. For a hand on approach to democratizing, Experimentation is a subject of a Harvard Business School case study. In this episode, we're going to discuss how Rohan successfully built the Experimentation program at Yelp from the ground up. Welcome to the show Rohan
Rohan Katyal 00:59
Awesome. Thank you for the introduction, Gavin.
Gavin 01:02
Ok, let's start with your background and your experience. Can you provide us with a brief overview of your background?
Rohan 01:11
Absolutely. I grew up in Delhi, India, went to school there. I majored in CS. At the time that--- During the time I co-founded a non-profit focused, nonprofit could find a way to help develop a income stream to fund education, for underprivileged kids, did that for about three years and then moved to the US for Grad school, went to Georgia Tech Yellow Jackets. Major in CSN design. Again, full time career, always been a PM. Started out at Yahoo in the new products team, where our job is to bring Yahoo back to life and did not succeed.
Then I moved on to Yelp where first I was the PM for Experimentation, built out their Experimentation Platform Program, scaled the practice, and then shifted gears a little to work on to --- use that Experimentation knowledge onto a specific product, where I helped grow request support, which is Yelp's performance local services marketplace, the other primary revenue generation mechanism. And then did that for a couple of years. When I was back in India at the beginning of COVID, saw people use WhatsApp in an extremely creative ways to sustain their business and generate more leads.
Got super excited about that. Came back to the US, met a bunch of super exciting and smart folks on WhatsApp. Ended up joining WhatsApp to work on click to WhatsApp growth which is, WhatsApp business messaging offering, did that for about a year, then help set up a similar growth effort for Instagram and Messenger.
What business messaging, and as a very--- did that for about again for three, four months to help set up the team, the charter, helped kick off the execution on the team. And as a very recently, I have joined NPE which is New Product Experimentation, which is Facebook's experimental products team. We're building a new product called Super, which is a tool to help creators generate a direct income stream.
Gavin 03:10
Awesome. So, thinking back to Yelp, when you were leading and building the Experimentation Program there, how did that opportunity come about?
Rohan 03:24
How did that opportunity come about? Honestly, I wasn't political, I had done Experimentation and being a part of the process. But I never thought about Experimentation as deeply. I've taken Stats classes.
During my Engineering Major and I've been through this process of what is part of P-values? Why is it important? Who experiments? But before I started and doing some Internships, in my head Experimentation was only for like, Medical purposes right, for making Pharmaceutical drugs and how do you do different tests?
And Yelp was a product I'd been using for a while to find plumbers, electricians, and restaurants. I was one of the few people who use it more to find plumbers instead of restaurants. So, I knew about the product, got excited about Experimentation when someone connected me to a data team there, who was setting up the Experimentation practice and that's how I got introduced to it.
Gavin 04:42
Ok, so it was quite fortuitous, and you really just followed your nose and an opportunity has arisen.
Rohan 04:54
Yes, absolutely. I would say that. I wish, I had a more grand story that I was always looking for that, and I was always seeking that. But yes, I just went out, talk to a bunch of folks who pointed me towards other smart people, and it--- there was a match.
Gavin 05:13
Fantastic. Thinking about that achievement of establishing and scaling the Experimentation Program at Yelp, where does that rank in your career achievements today?
Rohan 05:28
I'm super proud of that. It's hard to like, place it. I think it's been pretty. It's been a key to, how I've done things after the fact? I think it's given me that experience, helped me take a deep dive. Not into just the statistical side of Experimentation, but also the people's side of Experimentation which is often overlooked.
There's a huge people element to, how you experiment? I could give you the best infrastructure that exists. I can give you the best Tech that exists, but people mess up. Right. People make the wrong decisions interpret data the wrong way. That part I got to do a deep dive into that as well. I think that was personally for me.
One of the most exciting part of that experience like, learning more about the people side of Experimentation. And then I've used that and applied it to everything, that's happened afterwards. All the Growth work that I've done, all the Experimentation work that I've done. Yes, I would rank it pretty highly. It definitely makes my top three.
Gavin 06:23
Do you think from that perspective as a PM that, it's the learning that you took from establishing the program? It's maybe broadened your perspective to PM a bit more, to uplift \ more on the people side of change.
Rohan 06:47
Absolutely. First, I guess its broadened perspective. It's also like, it helps you think about growth much better. Growth is--- I think Growth is a game of inches. Right. Growth is like, you use, you build, you learn, you iterate and getting to understand that, people side of things helps you build that muscle, build measure learn muscle, and apply that to your day-to-day on,
How you think about structuring a Growth Program and also, how do you structure Execution? I think those are like, those things were my Key tactical takeaways from our experience, and how to apply that into my--- all my roles going forward.
Gavin 07:32
Good point. Thinking about your time at Meta now and thinking about your time back at Yelp, what are some of the key differences with Experimentation between the two organizations?
Rohan 07:50
Yes, great question. Very different Experimentation practices. Fundamentally, both follow the build, measure learn cycle. Right. That's what Experimentation is all about. However, when I started out at Yelp, it was a very different Experimentation landscape. There was extent. It's not that people weren't experimenting people, there was Extensive Experimentation at Yelp.
Several types of tests were being run but all of these tests were being run in silos. There was limited cross team intelligence. There were not as many Guardrails in place. So, we were very much dependent on people for making decisions, and data science to do a lot of Ad Hoc Analysis.
Now, switching gears to Facebook. Facebook's been doing this for much longer than your past Facebook's Experimentation. Practice is exponentially more mature than Yelp's, it was at that point, and arguably still is. It's a much bigger investment for Facebook, given its scale. And, because of that, there's data governance, there's centralization. I'm sure at some point, Facebook didn't have all of that either but I haven't experienced that at Facebook.
At Yelp, the Experimentation, practice required hand holding processes, more culturally execution. Facebook everything already existed and it's very robust. They're like, office hours, people helping you out. There's on-point engineers, the infrastructure's always up. It's a large scale of the Glasgow Infrastructure. There are all Guardrails in place, you get centralized metrics, your product specific metrics. So, first and foremost, the maturity at both these places are very different.
Now, fundamentally on how both companies develop products is very different. Yelp likes to go more deep on product and I think people--- and that's why my experiences a little biased, because the group I've been focused on, mostly on growth at both organizations. And obviously, especially at Facebook, micro cultures exists.
There are differences throughout, Yelp Experimentation approach was culturally integrate by educating more people and helping people understand what everything means on. Let's say, a scorecard. What is P-value? How do you interpret it? Facebook's approach was, we want to have bias for moving fast. So, instead of educating, having programs and like, all of that jazz, we're going to make the tool where the tool helps you interpret the data as well.
So, the annotation is the same. Everyone same speaks, the same language at Facebook, when you look at the tool. Red is bad or, green is good or, if it's in this much, it'll tell you that straight. So, this is not statistic. You can look at the P-values and you can get as much detail as you want. But you can also like run without getting into that much detail.
So, all Facebook's infrastructure almost abstracts away the complexity to help you to move faster. Whereas, Yelp was us giving the people more control, more details and leaving it to people for interpretation and focusing on educating people. Which I bet is also functional, where in the maturity phase of Experimentation infrastructure argue.
Gavin 11:17
That's a good overview. Facebook probably has a 10 to 15-year head start on Yelp, and its processes, its systems, its culture, its platforms, indicative of that level of maturity. This next question I like to ask all my guests I like to understand that, their thinking approach, their principles and their mental models, based on your time and Experimentation Growth, have you formed any key principles or mental models that you now work to?
Rohan 11:49
Yes, absolutely. For a specifically for Experimentation, I think some of the things that come to mind. And also, I think generally, they apply to scaling team practices as well, but more specifically to Experimentation.
One, you might have already heard me say this multiple times, it's not about the Tech, it's about the people. Focus on people. People are the key part that make or break the infrastructure, whether it comes to Growth or, Experimentation.
Second, is Standardization. Standardize, how people test? Standardize, how experiments are created? How experiments are communicated? How experiments are interpreted? If different people don't speak the same language, you will not be able to scale an experiment. If people are always trying to align on what is this metric? What is this dashboard? What does greed mean?
Third, is focused on probabilistic learning. Experimentation is not to ship, it's to learn. And there's a very nuanced distinction, but it's a very big one. If you're focused on just experimenting, as a precursor to shipping, you're not actually thinking about learning. If Experimentation tells you that something's good, it also tells you that something's not good, and also not shipping is, something new that you've learned. You've tested it, didn't work, you learn.
So, focus on probabilistic learning. And fourth is always being testing. Just keep on shipping, keep on moving, always be testing.
Four things:
- Focus on people
- Not the tech
- Standardize probabilistic learning
- And always be testing.
Gavin 13:20
So, just thinking about probabilistic learning there, do you think that the mistake, that some teams and organizations can make is, that Experimentation becomes a validation tool, rather than a learning tool? Have you experienced that?
Rohan 13:35
Yes, absolutely. Yes, firstly, you've hit the thing. It's a bang on. Yes, Experimentation and a lot of, when this practice is not done in a proper way. It is just a precursor. It's just a part of the process that you have to do. Right, and there's a lot of reasons why this happens. It's almost in some places, it's just a firstly people are like, Ok, egos come in, I'm smarter than this. I don't need to experiment. I've been doing this for a really long time. Everywhere is the same. This would work. Let's do it.
So, one is that--- but then you're forced to do it. So you do it, but you're not really doing it, to actually learn and take away or, check your hypotheses or, remove your biases. And yes, I guess that's the biggest one. Yes, just focus. Yes, I think getting guest work into decision making and the ego aspect of this entire product development process comes into the way, and then that scenario Experimentation just becomes like a cool thing to do.
Gavin 14:50
Yes, interesting perspective. Let's, jump forward a little bit and talk more specifically about the company and culture at Yelp. So, you touched on a couple of these points earlier, what was the environment and landscape you're facing into, at the start of the Experimentation journey?
Rohan 15:11
Yes, when I started out, as I mentioned, people were still experimenting. I or, my team or, none of us introduce people to Experimentation. Everyone knew about it. People were doing it. There were several types of tests. There was hacked together infrastructure, which lacked centralization. Because of that, everyone was experimenting in silos.
There was limited cross team, experimentation intelligence. If one team was experimenting, they didn't know the impact of that on other parts of the product and vice versa. Analysis was really manual. It took anywhere between 7 to 14 days and required a Data Scientist or, an Analyst to be involved in the process. So, there was a lot of wasted effort. And all of this was slowing down product velocity.
Gavin 16:00
Ok. Thinking about the trigger then, what was the key trigger that moved the organization to rally around Experimentation more specifically to, as you put it, to increase product velocity?
Rohan 16:15
Absolutely, the biggest trigger was decision making velocity. We wanted to be able to make decisions faster. We wanted to be able to ship faster. We wanted to be able to build a better product for a user and deliver more value for investors, and shareholders of the company.
Experimentation was becoming a key blocker, in how we were building product and iterating on different things that we were building? Experimentation essentially enabled us to accelerate our velocity and add more value to our shareholders, and our users.
Gavin 16:48
Thinking about the role that leadership played in that transformation journey, can you describe the role, that leadership played in being an experimentation enabler?
Rohan 17:00
Yes, the leadership was extremely supportive. But they're already been in setups with different levels of data and product maturity. So, their experience this--- put another way, they had been there done that, and like a bunch of different setups, where they had come, there worked at the big tech of the world, and they had worked with all the fancy startups of the world.
So, their experience, having no data practices. Somewhere in between, we're trying to develop infrastructure to also be at a place where Experimentation is very accepted. It's centralized, and it's--- there's a very mature infrastructure that exists, so, given the breadth of experience that Yelp's leadership had.
There was acceptance, firstly on with Experimentation. It's a valuable tool to have, it's almost like, there's no other way to make decision. It is the fastest way to make decision. Right. Without data, everything's just an opinion. So, one was that. They were extremely supportive of it, they understood the importance, and if they supported us because they understood, and they realize that having a solid Experimentation infrastructure would actually help us deliver value faster.
They supported us with investments. They've push for adoption. Whenever we need, we were receiving pushback from different organizations to adopt, move that product development to a new infrastructure, because it required some little work on the beginning, right, to move to New Experimentation interface. They helped us with top-down mandates, becoming evangelists for the program, and also helping us with, whenever we needed resources.
Gavin 18:31
It effectively was the perfect storm for change. You had really strong leadership support. There was a desire to change to transition from an Experimentation capability that was, somewhat fragmented, and disaggregated to a high performing organization to make decisions faster, and to then stump up the resource, and investment to make it all happen.
Rohan 18:59
Absolutely
Gavin 18:59
Ok, let's think about some of those Applied Elements of the Experimentation Transformation Program How did the organization structure the Experimentation team or, Growth team, what did that look like?
Rohan 19:22
Yes, the Experimentation team, we had four components to the entire very broadly speaking. There were four components to the Experimentation infrastructure. One was called the Beaker. Beaker was the experiment control infrastructure, where people would go and create experiments. Then there was Bunsen logging. Sorry, the name of the tool is Bunsen.
Bunsen, as the Bunsen burner used in labs. So, Beaker was used in labs. So, Beaker was experiment creation infrastructure. Then there was Bunsen logging, which was the logging infrastructure, which required, which was needed to compute record all the analytics. What we use it? What users doing? And these logs are processed, compiled and indicated, and processed to compute the metrics. And
Then there was a Scorecard. Scorecard was where all the experiment results were displayed. And the Scorecard interacted, displayed outputs from a Statistical package that we call BEAD Bunsen Experimentation Analysis, I'm forgetting the full form [phonetic] it's going to come back to me. But the BEAD package, which was the statistical package.
So, there were essentially four components to this. Now, all of these components had very close interaction between data. It was a close partnership between Data Science and Engineering. The product team for Experimentation was a part of the Data Arc, the data or, included Data Science and Data Product.
We essentially divide--- and the Beaker, Bunsen logging, and the Scorecard, these three things were owned by engineering. The BEAD package which is a statistical package was owned by Data Science. And my job was essentially to work with both parties, and to make sure we build what people need.
I was a part of the Data Product org, reporting into the head of Data Science. And the engineering effort that was supporting this was a part of the Data Infrastructure Arc. The Data Infrastructure or, they were engineers who were best equipped with the knowledge, and they know how to build this. And they rolled in to the director of Data Engineering and Data Infra at Yelp, and these.
Gavin 21:51
Let's touch on a couple of those points you mentioned there, the Scorecard is one area that really interested me. And I'd seen a graph and some data that you'd produced, that showed where the Scorecard was implemented, the number of experiments in the organization increased exponentially. Can you talk a little bit about the role the Scorecard played in increasing experimentation velocity?
Rohan 22:13
Firstly, before a Scorecard, people were--- people had queries in the team, different teams have different Data Scientists who were running analysis in a different way. And people at different teams were rewriting metrics or, rewriting the same queries again. Doing the same analysis again, one is that, it was leading to wasted effort, because there was no centralization.
Second, a bigger problem was when two Data Scientists try to write the same thing, same query, it often ends up missing. There's often a mismatch, which is actually not visible like, one person might have defined active user, as something else for the other person might--- and the difference is always like very nuanced. Especially at a company of your size because there're 50 events that a user can do, which would make you active, one of them only ended up counting, let's say 47. The other one ended up counting like all 50 of them.
So, the definition of the metric changes. Second, there wasn't a common language for people to speak about Experimentation. That was the big one. Every time if you would write up, everyone was writing. These results were shared in Google Docs or, Email, and people would phrase them, write them differently. Every time someone would look at it, they would have to interpret it waste of time, having to look at which part should I be looking at? Every time you would present to an exec, you have to create another deck.
So, two things were the primary problems, not having the same language to speak about Experimentation. And on the Analytical side, people were often using queries that didn't match or, results that actually weren't the same. Even though they were calling it the same thing, let's say for this, in this example, Active users. So when we launched Scorecard, we essentially gave everyone a way to speak the same language.
We essentially told instead of having, to write Emails again and talks again, and going, and explaining your what this meant? What this metric means or, what this new metric means, and having like other teams, care about your metrics? They would only go to the site. Let's say, I run an experiment and if there's another team who cares about what I'm shipping, because it might impact their part of the product, they can quickly go to the Scorecard without this new team coming into the conversation. And just have a look at all the Guardrails and all the checks in place and see if it's needs a conversation.
So, essentially, Scorecard gave by giving everyone the same language to speak about Experimentation. It reduced the overhead needed to develop product. And this became a good enough forcing function for people to drop what they were doing before, and move on to Bunsen and experiment, because this just came out of the box.
Gavin 25:23
So, to think about some of those key elements, you talked about common language, visibility, transparency. Do you think it increased trust with Experimentation in the organization significantly? Was that the ultimate outcome?
Rohan 25:48
Yes. So, I think, trust was for the function of like, a couple of other things. This gave people the same language to speak, not necessarily trust. Reason being, we knew that when people would talk about, let's say, Experimentation and going back to the example, I just gave about active users, people were using different definitions. And we were auditing the experiments that people were doing, what results were they making? And how many of those decisions were actually correct?
We just--- instead of having to no writing, no additional analysis needed. It automatic, it's out of the box. So, this became a big value prop. And also when we launched the Scorecard, we saw a sudden uptake in experiments happening on Bunsen. Because everyone started dropping whatever they were doing and they started moving on to Bunsen to do their experiment.
When I say correct, we did a Meta-analysis, where data science would like pick random samples of experiments, and see if they agreed with the outcome of the decision that was made. Why or, why not? As a part of this process, we find out about different problems, what was wrong? And what was--- why were people not being able to make these decisions, about people making correct decisions? In a lot of cases, people didn't realize that what the problems were? People didn't.
This is Data Science, because the audit knew that two people made a decision by having a conversation about active users. But actually both of them measure Active users in a very different way. Because we got both of their scripts, we audited them to figure out where the problems lie.
So, Scorecard gave--- made it the value add from the team product, teams’ perspective, was easier but less work, less overhead. I'm thinking with other teams, but not necessarily trust. Because they didn't know at that point that we were actually giving them the same language to speak, and it was they were problems in that entire process beforehand.
Gavin 27:21
Good distinction. Just a quick question around engineering capability, did you find some uplift was required in the engineering teams? Formulating and designing experiments is a different to day-to-day product design. How did that work?
Rohan 27:40
Yes, with the--- we focus a lot on that as, on helping different. We focus a lot on the process of where different, We thought a lot about, how different teams would onboard onto months? What would that mean, and what would that take? Essentially, you required a couple of lines. We built an entire API around this entire experiment infrastructure. There were two pieces to this code API
One was used to law. One was used to send cohort users, which would enable logging for different Cohorts and different users. And the second was the BEAD the statistical package, which Bunsen logging was used to figure out, which Analysis to do? Which metrics to pull? And all of that jazz. So, having--- by building two core API's and abstracting away of the all the complexity, we actually made it significantly easier for people to run experiments.
Prior to this, people would actually have to use their own, there was another three months, there was a different Cohorting infrastructure that existed, but that required a lot more work. People had, engineers had to write code. Engineers had to do this every single time, and it wasn't. And there were also different flavors of the Cohorting logic that existed. People would--- for engineers would fork it for their needs, and develop a different flavor.
There wasn't any centralization. There were about four or five different variants of the Cohorting service, for example, that existed pretty Bunsen. So, we took all of that. We took the guesswork out. We built an API for you to just quickly run experiments. Add a couple of lines of code here, you get Cohorting, you can quickly start logging things, and the status of package would automatically figure out, pick your logs and spit out results on the Scorecard. So, we actually made it really easy for people to run experiments.
Gavin 29:31
Yes, the thing that you've mentioned this a couple of times now, both at Meta and at Yelp is abstracting away all the complexity to make experiments as easy and accessible as possible.
Rohan 29:44
Yes, I'm a big fan of figuring out patterns in specifically in DevOps and just putting them behind an API, if there's something that an Engineer has to do more than five times. That's an opportunity for me.
Gavin 29:57
Excellent. Now, let's do a little bit of a deep dive on metrics. This is one of the areas that I'm really interested to understand. Talking about the formulation of those Guardrail metrics, can you talk us through the process and the journey to arrive at that outcome, please?
Rohan 30:17
Yes, before to give you a little bit of quick context before Bunsen, as I mentioned, multiple definition of the same metric, which caused a considerable amount of confusion. But with Bunsen, we wanted when decided building centralization with logging and stats. And now we had the opportunity because we had Centralized Analysis, we had Centralized Logging, and we had made it easy for you to talk about Experimentation. Then finally this gave us the opportunity and take this one step further, and build a Centralized Metrics Hub. That's not what we called it, but that's just an easy way to explain it.
We had three categories of metrics at a • Decision Metric • Tracking Metric and • Guardrail Metrics. Decision metric was why you experiment? What you care about? This is your goal. You--- if this is what you will primarily use to make a shipped or, no shipped decision or, learn about the experiment. Second, was tracking metrics. These are secondary metrics around the core top line that you care about, you don't want to see where they go. You're not negatively impacting them. You could potentially be, they could be moving.
But you don't make decisions based on tracking metrics. You make decision based on the decision metric and, because this is also factored into the Power Analysis. We're not, if we--- the more metrics you look at, the more you need to get more data, and it's going to take more time. So, the Statistical Engine at the back made use of this piece of information. This is a decision metric. This is a tracking metric, and how many of these exist? And how many Data Samples would we need to get statistic results? And
Third, were the Guardrail Metrics. I think Guardrail Metrics was super interesting. How the motivation for this was, as I mentioned before that, we did a Meta-Analysis, where we would look at experiments that had shipped, and whether Data Science agreed with the outcome of that decision or not? Should that have been shipped? Should that have not been shipped? And when we read further deep dives, in some cases, we saw that an experiment, that had actually shipped, had a negative impact in on another team's product. And that team had never found out about it, because these metrics had never been computed. And some cases were intuitive. Some cases were not intuitive.
Now with this, we started looking at how other people or, other companies have solved this problem of providing more transparency and visibility? And after having done a bunch of research on looking at, what other people, other teams were doing? When I see other teams, I mean, non-Yelp teams, other companies. We came up with the idea of Guardrail Metrics for in the context of Yelp.
The idea behind this was that, we're going to have top line company level Guardrail Metrics that the entire company, to the Chief Product Officer level cares about. And these are metrics that no one should harm beyond a certain limit. Now, essentially helping people define their trade-offs. Now, in order to like, actually evangelize this, we could build the logging and write the queries and like, operationalize the metric. But there was a huge cultural aspect of this. I can't just come up and say, everyone stop, what you're doing? There’s 20 metrics. This is what we all cared about, for like, a 6000 people company.
How do we evangelize it? First step was to get buy-in from the heads of all of these different product teams the VPs at Facebook. How do you get buy-in from them? We took the audit that we did to different VPs, to get buy-in to show them that, how their orgs had been negatively impacted without any visibility to them that got attention from them that got us in room with them, and this became a conversation with that point.
After, that we devised the program, where these VPs would tell us the product owners of these orgs would tell us about the metric they cared about the most. They would become the owner of thus metric and the small exact sponsor of this metric. We would build it. We would operationalize it. Now we've built infrastructure. We've got buy-in from VPs and VPs have put a top down mandate that, we--- every experiment as part of the process, the analysis process, we will look at Carter's metrics to make decisions.
However, now the metrics are being computed, but there's so many experiments running all the time. How does one team even know this? Out of these 1000 experiments, this one could potentially impact reviews or, potentially impact act like, Active users? Then we build the final piece of the puzzle, which was an alerting system. If a Guardrail was trending negative, warning Email would be sent to all the key stakeholders.
If the Guardrail had hit that, beyond the accepted range, then everyone would again get a Email, and if it had, there was some--- there was another limit define if I had finally crossed the fully acceptable limit, the experiment will be paused till we get sign-offs from different parties to actually conduct an experiment again. Now this was a step back from what we had initially set out to do. Initially, the whole idea of experimentation was to increase velocity. But adding this Guardrails and this Experimentation,
This cross-team intelligence added more friction, which slowed people down, which was counterintuitive to what we were doing. But given the increase that we saw in the quality of decision making, there's a trade-off intentionally made. And when we started rolling out Guardrails, initially, we saw an increase in Experimentation. But then we started seeing a decrease in velocity gradually till it plateau. But we saw a significant increase in the quality of decisions being made. And it was a trade-off we were willing to make.
Gavin 36:14
Yes, interesting trade-off between quality, velocity and do no harm, right? So, thinking about that metric submission process, how many candidates did you have submitted, when you starting to consult with all of the departments for their key metrics?
Rohan 36:38
We let them define it. We got buy-in from them on the revenue laws or, whatever they cared about this is law that potentially happened. And then we let them tell us what metric mattered to them.
Gavin 36:54
Ok, and that arrived at a set of 20 or, less that we used going forward.
Rohan 37:01
When I last, when I was there, but they were about 19. Now that number might have increased or decreased. I'm not sure. But yes, there were 19.
Gavin 37:08
How long did that journey take, how many months?
Rohan 37:12
To build 19 metrics
Gavin 37:14
To agree and align on the core set of metrics
Rohan 37:19
Two and a half to three quarters, long process. It was a long process. And also like, because after you agree with an expert, let's say, you agree that you need a metric, we get sign-off, then you start having conversations around. Ok, this is what you said, we need. This is what we have right now. This is the logging that exists. Now we need more logging. Can we do that? Ok, we can do it, go ahead.
Let's make it whatever a couple of months prioritize do it. If you come back, and this logging just can't happen, then you go back, and then you figure out what's the closest proxy to this. Then you go back again, figuring out the logging. So, there's a lot of back and forth, figuring that stuff out.
Gavin 38:00
Ok, let's think about the Cultural Transformation and Cultural Integration, I have previously heard you say, prior to this Podcast that was the most challenging element of the transformation project with Experimentation. And today we've suggested that 50% of the job is performing experiments and 50% is Organizational Cultural Transformation. Can you talk us through some of the key elements of the Cultural change?
Rohan 38:31
Yes. Absolutely. Well, I've been saying absolutely a lot. Need to stop doing that. But three steps, I think there were three key elements of this entire aspect. One was, as I mentioned, we went down the education route, we said we given the size of Yelp. And given our engineering effort we had, we could educate people faster than we could iterate or, build and do different things. So, we started both streams in parallel and focus a bunch on education. That actually I'll get into details, that helped us a lot, the statically what did we do.
Second was Standardization. A big part of Cultural Integration is first you educate people so that they know, what they need to look for. Second is to Standardize them. So, you give them a same way. Everyone's been educated. Now everyone needs to be able to speak the same language. And third, was now you monitor, if you're actually up leveling and being able to do what you set out to do.
So, educate to up level. We got buy-in from teams, to get, to be focused on Experimentation education. The Data Science team developed an Experimentation guide that, we saw, we had like, a mandate from VPs that everyone will have to go up, go through. And at the end of this after you've done with this guide, there was a short like, I think, 8, 10 question quiz that the Data Science has developed.
The Data center had developed that all PMs had to pass when, who already were at the company. This was for all the existing people. Now new PMs were joining, Product Managers were joining the company all the time. So, this became a part of the on boarding experience at, for people to go through this Experimentation training. It was actually pretty short and pretty quick, but just helped you make, get at par with like, Experimentation practices at Yelp, and also help up level people. Now, when this helped up level in general help up level, the entire company with respect to Experimentation and basic understanding of Stats.
However, we also wanted to create Bunsen evangelists and different parts of the company. To do that, we developed a four-week training program, to train people, to become Bunsen deputies. These people were essentially for any Bunsen problem or, Bunsen questions, they were the first touch point in their own teams. They became Bunsen, this helped us. This helped us embed Bunsen evangelists into key product teams, which helped facilitate and encourage widespread adoption of Bunsen. So, this is how we statically did the education piece.
Now coming to the second one which is Standardization, Standardization was before, during and after the experiment, after the after the test was created. Before the test was planned, before when we did the experiment design was being talked about or, created, we created something called a PEP a Product Enhancement Proposal. It was essentially a template to, how do you document experiments? How do you think about hypotheses? And how do you think about power analysis? And how metrics and everything
So, It was a template that we created, which was actually published as part of the Harvard Business School case study, and also has been used by several other companies for their practices as well. So, people started documenting and taking about experiment design, using that template. It was a checklist to make sure you've thought through all the things. During we Standardize by adding Bunsen logging, taking all the logging burden, the metric burden, and also by centralizing the analysis piece, BEAD package.
And third was, after the fact we already talked about it, the Scorecard. Now, you were talking about Experimentation in the same way. Now we had educated people, we've given them the tools to actually speak about it the same way, uplift in theory, statistical upliftment that happened. But now how do we measure it?
To measure it, we started conducting a regular Meta-Analysis of past experiments, to determine the proportion of experiments that were correctly designed and executed. This is the audit that I was talking about. And we initially did this as a one-time exercise to actually figure out, what were the areas where we could add value by building this new program. And
Eventually, this became the North Star of our Experimentation product development process. And we started doing this every quarter, and reporting it to execs. And I think, over the course of one and a half, the first one and a half years, we were able to improve this particular metric proportion of correct decision, the decision accuracy metric that we used to call over two times. Over we had 118, 120% something like that, some over 100% improvement in correct decisions being made at Yelp.
I think cultural difference was a function of three things • Education, • Standardization, and then actually • Monitoring and measuring the decision accuracy to see if things were--- if we were actually correctly experimenting our Experimentation platform within the company.
Gavin 43:51
If you were to conduct a retro for the Cultural Transformation, was there something that just didn't work?
Rohan 44:01
Something that didn't work
Gavin 44:04
Or, something that you would advise other organizations. It didn't work so well.
Rohan 44:14
Think when I'm talking about this, it's seeming like, this was a linear process that we always knew it was a people more problem, focused on people and not in Tech, like Standardization seemed obvious. These were essentially our learning. Then none of this was a linear process. There was a back end. This was a very haphazard, circular motion. No, this was not even needed. We invested like, a month into this. This is like, complete garbage. Let's throw it out. We need to build something else. So, we went through this back and forth, a bunch of times and we built a quite a few things, that were actually no one really like, cared about it actually needed.
I think one of the things was we built like Automation for people to dimensionalize experiments like, split this metric by country split, something by, some other country or, some other attribute. But those were so nuanced. That one people were not using it. Everyone always had more attributes. Than Bunsen already had all of them, didn't more logging. So, we didn't really think through this. And then we again went back. And this was again, this comes back to the same thing. We can keep on building endlessly building tech, but like people's needs are very different. It's a people issue. People need new things; people are creating new products. So this dimensionalizing thing is not going to work. And this is sounding like a very linear process. But
It's not like Standardization education. These things were very after the fact. Now I can say, yes, it was probably a people problem more than it was a tech problem. However, if like someone was starting to build out their Experimentation platform, I think, one thing I would say is, audit your current needs by looking at four things. •What is the volume, actual sizing? • What is the volume of tests that you're running? • And what volume of tests you want to take the company to? That defines a large part of it. How much can you potentially, if I was to give you the best infrastructure, can you really do 1000 experiments? Do you have enough engineers? You have enough designers? And all, do you have the organizational capability to do it? Be real with yourself.
You don't always have to build the best thing. Sometimes good enough is good enough. Second is, what is your required accuracy levels for, when you're trying to make decisions on medical COVID vaccine? For example, you need very high decision, very high accuracy. But in some other cases, where there are, some parts of the product which you reasonable, you want to be reasonably comfortable when making a decision. You're fine not having as much accuracy. And also, in some newer products you need to be honest with yourself like, Experimentation wouldn't help. Like,
If you have, let's say 10 people, you're building a zero to one product, and you have 10 people on it, you can't do any Experimentation. It's part science, its part art. Go talk to people, you need a certain amount of scale for it, to actually be worthwhile. You could be featured, you could be rolling it out, instead of doing A/B test. And third, is understand the company's existing Data Aptitude. This is also where that Cultural and Ego aspect comes in. You want to be real with yourselves. Either people understand Experimentation or, data or, people don't. Right, and at Facebook for example, Facebook, a general product managers, and even other functions have very high Data Aptitude. So
Decision making and these product decisions can be seen in the how the Experimentation Infrastructure is built. If we don't--- at Yelp oftentimes, we might initially, we had to do additional hand holding as a part of the process. At Facebook, you don't need to do that, the Data Aptitude. That's just the nature of people who work at Facebook. The Data Aptitude is high. And after you've done this audit, I think it's useful to buy before you start building your own Experimentation platform. Buying is way easier. There's a cost of buying as well. Buying is not free.
It's not just money, you need Active Engineers, Active Data Scientists to be hand holding and helping people. But building is exponentially more expensive. It's starting a new business line, even if it's an internal product. So, a lot of investment in engineering figuring out what to build? And doing these audits so, buying initially at least, when you're starting out, buying is much better than building in my opinion.
Gavin 48:26
Good point. One of the key things that I took away from the summary there is that, when establishing an Experimentation Program, you need to be prepared to experiment on your own Experimentation Program that, it's a very messy, ambiguous process. It's non-linear. And there's no right way.
There's only a way that works for your company. And you need to figure that out. So let's finish off with our fast four questions. Now, can you think of a series of experiments that you performed, that shifted Organizational Thinking or, Organizational Perspective?
Rohan 49:05
From products that we shared or, people started thinking about experiments in a new way?
Gavin 49:10
Yes, thinking product side, maybe some opinions or assumptions that were busted?
Rohan 49:15
That's a tough one. I think, at Yelp, it was definitely this idea of learning experiments. Before we did that or, we evangelize this idea. People want shipping to learn. People were always shipping to ship sorry, experimenting to ship there. This idea, that you could do an experiment, to just learn something about the user did not exist. But when I joined a new team, we were building a new zero to one product. And we launched an experiment. And our entire goal outcome for that was, we had three hypotheses, and how users would react? It wasn't a new change, but it was educating a bigger change that we wanted to make.
That was a big, I think, fundamental change around, how people were thinking about experimentation? This seems a good idea. We can use this for learning. These are cheaper, you can do painted door tests around this. And this helped. Often, this helped people experiment in a quicker and in a way, where they could make lowered investments.
Gavin 50:16
Good point. Number two. What are you obsessing about right now?
Rohan 50:22
What I'm obsessing about. One is helping Creators Monetize their audience that's become my day job. I'm super excited about it. See how we can do that? Second is, I don't know if you follow cricket, but the cricket T20 World Cup is this year. So, I've been eagerly waiting for that, and see if I can go make it to Australia, to watch it. And third is the price of Doge coin. I'm stressed out about that. Things need to go up. Cannot buy any more dips.
Gavin 50:52
Three pretty diverse areas to focus on.
Rohan 50:56
You got to keep it interesting.
Gavin 51:00
What are you learning about, that we all need to know about, outside those three points of interest you've just discussed?
Rohan 51:10
One is zero to one Product Development. This is my first role where it's truly 100% zero to one Product Development. This is the first time where I've been in a position where Experimentation doesn't help. We just don't have users. So, it's a very new experience for me, which I'm super excited about. And
I think everyone should experiment with this at some point. What happens when you just cannot experiment? Your best bet is going out and talking to people which you should always do. But when that becomes your only option, it's super interesting. So, that's one thing. Second, outside work also just recently discovered my passion for CrossFit, which has been super fun.
Gavin 51:55
Good challenge for you. So, last one, thinking about some resources, some books, Podcasts, blogs, that have helped you on your journey. What would some of those key resources be?
Rohan 52:11
What would my key resources be? There's a lot. I talk a bit about general things that helped me, I think that get better at thinking about products and also the industry in general. And how these things facilitate better? I guess, almost better hypothesis for the test that you want to run.
One is Stratechary. It's my favorite newsletter by Ben Thompson. It's just an absolute must, if you work in Tech. It's amazing. I make sure this is one thing I read every single day.
Second is, something that aside reading more recently is Lenny Rachetsky's newsletter, on again, product. He goes about varied topics around marketplaces, product growth, subscriptions. Super interesting read. It's pretty good to get insight, into how different companies operate and think about problems?
Third, is I think, a very good--- it's most, it's an article, more than a blog, is building a culture of Experimentation by Stephan Thomke, if I'm not butchering his name. He's a Professor at Harvard Business School. He talks a lot about Experimentation on Social Media and does a lot of work in the field of Experimentation. It's a super interesting person to follow, if you want to learn more about Experimentation.
Another one is good experiment, bad experiment by Fareed Mosawat. It's, I hope I'm not butchering his last name. He is on the Reforge blog. It's a very good note on what constitutes a good experiment and bad experiment? He also runs Experimentation Program or, used to run. I think for Reforge, whichever really good things about, and final one plug-in, for you to check out the Yelp Experimentation [phonetic] case, where we documented everything that we did in more details.
Gavin 54:03
Awesome. Thanks so much for those. Let's leave it there. We're out of time. And thank you so much for your time today Rohan. Great chatting to you, and thank you for the insight and all the detail. Amazing.
Rohan 54:16
Thank you, Gavin. Thank you for having me. It's a super fun.
“Experimentation is not about shipping product, it’s about learning. If you’re focussed on experimenting, only as a precursor to shipping, then you’re not learning. Experimentation can tell you if an idea is good, or an idea is not good. Not shipping a feature is learning. You’ve tested, it didn’t work, you learn.”
Highlights
The people and cultural side of Experimentation is the most commonly overlooked. People are a huge part of how a company experiments. It’s no point having all the right technology and systems if people can’t interpret the data and make bad decisions. Rohan suggests “cultural transformation was the the most challenging part of building experimentation at Yelp”
Growth is a game of inches - you experiment, you learn, you build, you iterate
Experimentation culture at Yelp - is people focussed - has a culture of educating the team to help people understand how experimentation works. A focus on education and training programs to build capability and understanding. People have more control to interpret data and make decisions.
Experimentation culture at Facebook (Meta) - is biased towards moving fast - abstracts away all of the complexity of experimentation using technology to help people run faster. Less emphasis on data analysis and interpretation and more emphasis on faster, quality decisions. Experimentation maturity is higher, with a shared, common language around experimentation
Standardisation of experimentation is critical - how tests are designed, how tests are executed, how tests are communicated, how tests are interpreted. If the entire organisation does not speak the same language, you will not be able to scale experimentation
Experimentation is not a validation tool, it’s a system of continuous learning. If you’re focussed on experimenting only as a precursor to shipping, you’re not focussed on learning. Not shipping a feature is also organisational learning
In the early days at Yelp experimentation slowed down product velocity - experimentation in silos, no cross-functional experimentation intelligence, no awareness on upstream/downstream impacts of experiments, hacked together systems and tools, lots of manual data analysis and duplicated, wasted effort
The trigger for the Yelp experimentation program - to be able to make decisions faster, to be able to ship product faster and create and deliver a better product for users. Experimentation had become a key blocker and was decreasing product velocity
Strong support from the Leadership Team is required - there was an acceptance from the Yelp Leadership Team that experimentation was a valuable asset and the best way to make quality decisions. It’s the fastest way to make quality decisions, otherwise everything else is just an opinion. The Yelp Leadership Team provided resource, funding and drove adoption
Experimentation Scorecard - was a strong forcing function for Yelp to increase experimentation velocity exponentially. Created a standardised communication tool and language within the business
Yelp designed three categories of Metrics (1). Decision Metrics - strategic goal metrics (2). Tracking Metrics - secondary short-term driver metrics and (3). Guardrail Metrics - monitor negative impacts of experiments. While the Guardrail Metrics, at times, slowed down product velocity, they ultimately increased the quality of decisions and transparency on trade-offs
Key Pillars of Yelp Cultural Transformation (1). Experimentation Guide - education on statistics and data interpretation (2). Product Enhancement Proposal - standardised experimentation template (3). Scorecard - experimentation communication tool (4). Bunsen Deputies - experimentation evangelists in cross-functional teams (5). Monitoring - meta-analysis of past experiments to monitor decision quality
Building a culture of experimentation in a large enterprise is a messy, non-linear process. You need to experiment on your experimentation program to figure out what will work. Every company is different. What works in one organisation, may not work in yours
In this episode we discuss:
Leaving a legacy at Yelp
The differences between experimentation at Yelp and Facebook (Meta)
Rohan’s top four experimentation guiding principles
Experimentation at Yelp in the early days
The role of Yelp leadership in accelerating experimentation uptake
How experimentation was structured at Yelp
Challenges with experimentation in the organisation
How Yelp aligned on a core set of experimentation metrics
The key pillars to experimentation culture transformation
What Rohan’s learning about right now
Success starts now.
Beat The Odds newsletter is jam packed with 100% practical lessons, strategies, and tips from world-leading experts in Experimentation, Innovation and Product Design.
So, join the hundreds of people who Beat The Odds every month with our help.