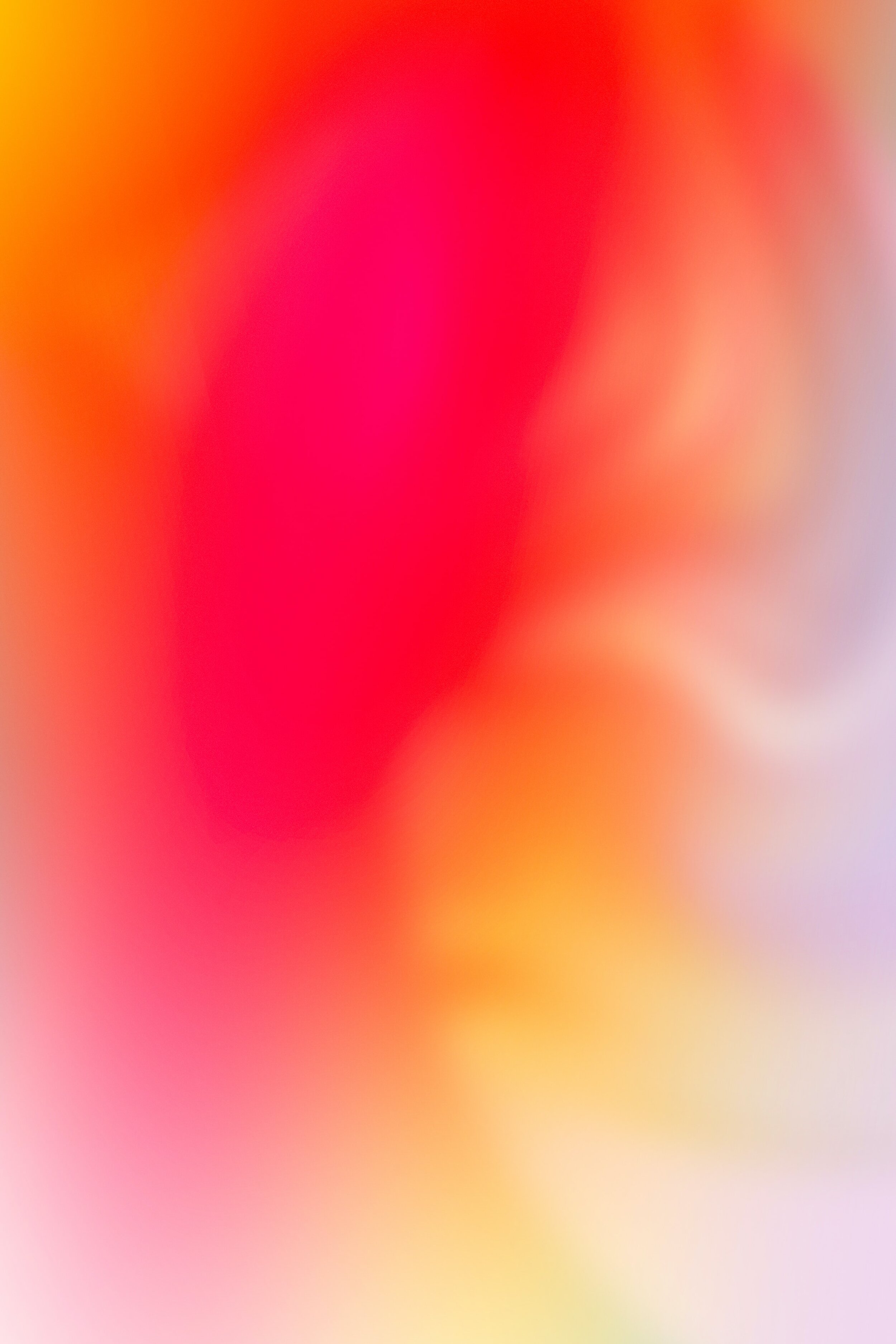
February 24 2022 • Episode 006
Michael Luca - The Power Of Experiments: Decision Making in a Data-Driven World
“Over the past 50 years we’ve seen a growing body of evidence that indicates that we don’t know in advance what will work. Our intuition is flawed. Experiments are one way to check our intuition, making sure that we’re removing biases from our decisions where possible.”
Michael Luca is an Associate Professor of Business Administration at Harvard Business School. His research, teaching, and advisory work focuses on the design of online platforms, and on the ways in which data can inform managerial and policy decisions.
His research has been published in academic journals including the Journal of Economic Perspectives, Management Science and the American Economic Journal. He has also written about behavioural economics and online platforms for media outlets including The Wall Street Journal, The Atlantic, Wired, and Slate.
Professor Luca is a co-author of The Power of Experiments: Decision-Making in a Data Driven World, which received favourable reviews by publications including the New Yorker and the Wall Street Journal, and has been used in the MBA classroom for courses on business analytics and on behavioural economics.
At Harvard, Professor Luca developed and teaches an MBA course on using experiments to guide managerial decisions, called From Data to Decisions: Leveraging Experiments for Effective Strategy, Marketing, and Entrepreneurship. He has also taught and developed materials for executive education and MBA courses on platform design, behavioural economics, and business analytics.
Get the transcript
Episode 006 - Michael Luca - The Power of Experiments: Decision-Making in a Data-Driven World
Gavin Bryant 00:03
Hello and welcome to the Experimentation Masters Podcast.
Today I would like to welcome Michael Luca to the show. Michael is an Associate Professor of Business Administration at Harvard Business School. His research, teaching and advisory work focuses on the design of online platforms on the ways in which data can inform managerial and policy decisions. His research has been published in leading academic journals. He's also written about behavioural economics and online platforms for media outlets, including the Wall Street Journal, The Atlantic and Wired. At Harvard professor Luca developed and teaches an MBA course on using experiments to guide managerial decisions, called from data to decisions, leveraging experiments for effective strategy, marketing, and entrepreneurship.
In this episode, we're going to dive into the book that Michael co-authored with Max H. Bazerman, The Power of Experiments: Decision Making in a Data Driven World.
Welcome to the show, Michael.
Michael Luca 01:15
Thanks Gavin, for having me.
Gavin Bryant 01:17
So let's just discuss your start with experimentation. Where did you find your way into experimentation and develop your passion for experimentation?
Michael Luca 01:31
So in my research, I've always been interested in how insights and tools from the Social Sciences can be useful for organizations. So over the years, I've done a lot of work with companies and with governments around this. And during that journey, and I've done a lot of work, where I run my own experiments and help to provide guidance to organizations. But the thing that I realized and had a series of conversations with Max about is that it's no longer just the insights from social sciences. So it's not just the findings of experiments that are becoming interested for organizations, but it's the experimentation process itself.
Now, we noticed that it's no longer just sort of this esoteric set of academics that are running experiments and coming up with insights and then telling those insights people, but it's actually companies and governments that are saying, How can I better use data? How can I better use experiments to help guide my decisions in a day to day process. And what we've been interested in is the complementarity between some of the tools and findings from social sciences, and how we could put this into practice in organizations so that they could take the academic research a starting points, but then further develop their own processes and their own findings.
Gavin Bryant 02:48
Excellent. So thinking about your own personal philosophy, or your experimentation thesis, what are some your guiding principles that you work to with experimentation?
Michael Luca 03:00
For Me, experiments often start with really asking the right question. So if you're a leader; your organization, your decision maker, it's important to realize that having data and having experiments is not quite enough. You need to have the right frameworks to map from those experiments back to decisions that are at hand. And it's an area that I've been passionate about, because I think that there's often a gap between the experiments that are being run and the decisions that are being put into place.
Gavin Bryant 03:35
And we'll come back later in the podcast to a really good example that Michael provides around Alibaba, which highlights that it's really important to ask the right question upfront, which informs that of the direction of the hypotheses and experiments. So we'll come back to that one.
So let's start off with a somewhat philosophical question, maybe? Do you think that it's really fair and reasonable to compare digital online experiments to social science and medical experiments?
Michael Luca 04:13
So I think there are parts that are analogous. I think that when we think about where experimentation has become more common, so you can think about an academic research. You can think about a medical trials, and now you can think about online platforms and the tech sector. So what makes the tech sector good fit for experimentation? Like, how do they discover that?
Well, we could think about some features of the tech sector. They have data, they have outcomes that they're actually measuring. They have large number of people often that are engaging with the platform, and then they're able to randomize people in a different treatment. So if you're deciding whether platform should have a blue background or a yellow background, you don't have to just guess, you don't have to just have discussion on one team about it, you could just test the two and try to figure out which one people are more likely to engage with.
So I think the dropping costs of data, the increase technical, ease of running experiments have sort of come to the forefront of why we see more experiments in tech. But there's also something else that's going on. And even though parts of the book are focused on the tech sector, we point to another or set of organizations where experimentation has become common, which has some similar themes, but also some others, now which is government. So he talked about the Behavioural Insights Team, we focus on the UK and one of the chapters there, but Australia has one as well. And countries are increasingly growing these teams that are focused on decision making.
So why experimentation there?
I think over the last 50 years or so we've seen a growing body of evidence that it's hard to understand exactly what would work. Our intuition could often be flawed. And experiments and other types of data are one way to help check our intuition and help to make sure that we're removing biases from our decisions where possible.
Gavin Bryant 06:12
Yeah, excellent point. So thinking about the leadership toolkit, in your book, you highlight that experimentation is a critical component of any leadership toolkit in the new age of management. Why should experimentation be a critical component of any leaders’ toolkit?
Michael Luca 06:34
So it's a real blind spot. If you're not thinking about experiments, as a leader. Now, you might ask yourself. Do I think my organization should be trying new things? Most leaders would say, "Yes."
Should I have a sense of humility? Most leaders who should say, "Yes." Should I get more information about what will work when it's feasible? Most leaders would say, "Yes", so then you should be experimenting. And you should be seeking out not only your own experiments, but look at the literature, looking to your other organizations and their experiments to try to learn more about what works and what doesn't work.
Gavin Bryant 07:10
And what do you think holds leaders back from trying experimentation in the first instance?
Michael Luca 07:19
It's a great question. So I think part of this is just that experimentation hasn't really been in the common vernacular for all that long, right. So I think we sort of have historically thought of this as a social science tool, maybe a little bit of medical trials. Now, more recently being decades, thought about it in the context of tech platforms, and maybe evaluating ads. But you know, let me give a concrete example of how hard it is to change the culture of the organization, we can think about the tech platform, eBay. So we have a chapter on this in the book. Now, they've been running lots of experiments. So it's an experiment heavy organization, they have data, they know how to use data.
Well, the thing that we talked about in the book isn't necessarily an early success, but it's actually a cultural change that got us intrigued about eBay and experimentation. So they had been experimenting on the design of their platform. And they had been advertising on Google ads, and Bing Ads and other advertising platforms to kind of bring people in to even encourage people to use their platform.
Now, they have been running this thinking our platform seems to work. And they had some evidence. And in fact, they hired a consulting company to come in and do an analysis of the impact of their ads. And they produced a number of compelling facts. And they showed them that the people that were seeing the ads were likely to buy stuff on eBay afterwards, maybe that seems like a success. Then they looked at how their ad spending varied over time and across place and saw, okay, well, when you are advertising more in California than New York, then you see a spike in purchases in California relative to you know, so you look at this data, and it seems like it's a win. But a group of economists who are trained in the tools of causal inference had actually come in and said, "Not so fast." You haven't run an experiment here. There are some problems with that analysis. In particular, ads are very targeted. So if you're just looking at where as they're getting spent, they're going to be sent to people who are most likely to be purchasing on eBay anywhere.
So this team of economist Tom Blake, Steve Salas, Chris Mascow, ran this large scale experiment where they actually started with some natural experiments and ran a new experiment where they turned on and off eBay ads in different markets. And what they found is that most of the money, which is about $50 million a year that eBay was spending on ads seemed to be a waste. And the problem was that the people are coming in through ads. seemed like they would have come to eBay anyway. And it's the experiment that taught them this lesson.
So what did eBay do afterwards?
So there are a couple of things they did. So they kind of change their strategy a little bit. But not only did they tweak their ads, which I think is of interest, probably only a subset of people would be listening here. But what I think is a general lesson is that they thought about other areas where they might then start experiment, should they just have a more experimental mindset for all of the ads that they're running? So I think once you start to think like that, you say, look, even if you're already running some experiments, you as a leader should be thinking about what are the different pieces of my organization, and where am I going bring in a little bit more data to help guide my decisions. Not only is that going to help me discover new things, but that's going to help me to test existing products and services, and see if we've been on the right track for things we're doing.
Gavin Bryant 10:53
Yeah, it's a fantastic example there. Just to dive in a little bit deeper on one of the points you touched on that earlier was culture. So if leaders are seeking to create an organization that's dedicated to experimentation, and evidence based decision making, the culture is really important. Are you able to highlight some of those cultural transformations that are necessary to start on that journey with experimentation?
Michael Luca 11:23
So I think some of this is just leaders being willing to engage in discussions of data and evidence. Now, what are some of the things that you need to get, right? So you need to think what are my objectives as an organization, and when I'm running experiments, are the outcomes that I'm measuring aligned with outcomes that I care about, like I want my KPIs to sort of be as closely connected back to the things that I'm measuring, which are going to be different as possible.
1. So one is sort of thinking about our objective functions.
2. The second is thinking about what are you as a leader trying to learn from an experiment? Like, are you trying to evaluate one specific product, one specific service? Or are you trying to develop some sort of internal framework, so I think kind of leader who understands that different purposes that experiments could play is going to have an organization that then gets more out of experimentation.
3. The third thing I would point to, is that you can't just reward victories, in a sense, like, you have to redefine what does it mean to be successful as an organization. So, trying and failing, trying and failing early, and then moving on to the right thing, should be rewarded rather than punished.
So sort of having the humility to know you might be wrong, and then having the agility to then say, okay, we tried these different options, these didn't work well. But now it set us on this different path that set us up for long term success is an important part of creating an organization, it's going to thrive by leveraging experiments.
Gavin Bryant 12:58
A really good point that you make there is about what a success looks like for the business and really, in an experimentation led organization that becomes a focus more about learning, and the value of learning? Have you seen instances where that can become problematic? Because if rewards and incentives aren't anchored around learning, then you drive a different behavior?
Michael Luca 13:29
Yeah, so I would say that in lots of situations that if you're just trying to come up with a thing, to pitch a solution without having evidence that it works, it can be problematic, but I guess, I would almost rephrase that a little bit to think about, like, what is an example where by tweaking the rewards and sort of like trying to think a little bit about how can we get more experimentation and clear evidence so we can benefit?
Now one example where I was involved is we had done this academic research, looking at Airbnb, and Airbnb had already been running, like 1000s of experiments, right? So they kind of were experimenting at a pretty large scale. And we had come in and started looking at not just the productivity of the platform, but the inclusivity of the platform. And what my co-authors Ben Adam and Dan Sversky and I had looked at is we ran an experiment where we tested for racial discrimination on the platform, we found widespread discrimination against African Americans on Airbnb.
So we surfaced our findings. We presented it publicly and spoke with people at Airbnb about it as well, and based on our findings, I think that disconnect there was that the company had been optimizing to narrow a goal. So they have been thinking about things like short run conversions on the platforms, but not thinking about the risk of unintended consequences, like the fact that you may be achieving some short run metrics that kind of is in your corporate goals, but not thinking about this long run inclusivity or lack thereof that they ended up facilitating with it.
So as a result of our paper, they created a task force and ultimately created a new team that was centered around thinking about how do you reduce discrimination on the platform?
So I think there they rephrase it to learn not just kind of how do we encourage short run growth? But how do we make reducing discrimination, more of a corporate priority as well, and then have experiments that are aligned with the goal of learning how we should go about doing that? I think once I had that mindset, then they trialed a whole bunch of different things to try to see how they could keep growing the platform, while not having a platform that facilitated discrimination.
Gavin Bryant 15:48
Mm hmm. Yeah, that's a really good example in the book that you discuss. So I recommend to the audience to review that. And I think that's a really good example of thinking about.... You suggested the unintended consequences there. So extrapolating thinking now, and looking at more of those second order effects, and those third order effects, which can sit outside and immediate and I guess, razor sharp focus on a metric. So it's about thinking more broadly about the impacts of those experiments to...
Michael Luca 16:24
And when you say razor, some of it is trying to think about things outside of the experiment. But other things are thinking about how do you bring more things inside of the experiment. So once you start thinking about the fact that, you know, I want a platform that's going to be widely used, I also want a platform that's not going to create, facilitate discrimination, you could bring that more directly into your suite of metrics, and take a more holistic view, even though it's still a very data-driven view and how to proceed,
Gavin Bryant 16:56
Yeah, that's a good point. So thinking about managerial decision making, one of the areas that really interests me, and I observe in my work, is many leaders, still making decisions that are based on past experiences, intuition and feelings? Why do you think that so many leaders still prefer to make decisions in this manner with sometimes not such a focus on an evidence based decision making approach?
Michael Luca 17:32
So let me return I guess, to the eBay example for a second, right, because I think this is also a nice illustration of that. You come in, you're the marketing director, you wrote this marketing campaign, you've designed the whole strategy. And now you're trying to think about should I run an experiment to show me that the strategy was not effective? It's a tricky territory there, you've put this leader of this part of the organization and, and I think, sort of there, we get unbundled out into the different pieces, like first, once I've designed a thing, I may be more inclined to think that it's going to work right. So I may be over confident about something I just put together. And I think by tweaking the incentives a little bit and tweaking the norms a little bit to get that information, seeking step, you get help to combat that in a way that's going to help people think not just how do I design something that's going to work? But how do I design a good test of whether that's going to work them, then sort building on that a little bit, you can think about how you have different parts of the organization all engaging. So sticking with the eBay experiment a little bit further. Now, there's the marketing team. But no, they also had the Econ team that came in to talk about this, and they also had a finance team that was involved.
So by having different stakeholders who had different lenses, they were able to sort of break through some of these barriers, and get a more holistic view that then push them to do more experimentation. To now we can sort of zoom out and say you're sitting at a company, and you feel like experiments would be helpful, but the other leaders don't all agree. Why don't people agree and what can I do about it? I think understanding the source of the barriers, experiments might be costly, the person may not want to find information, they may not have the right incentives, they may want the information but may not know how to change even if you bring them new evidence. Those are important barriers.
So I would urge leaders to think, what are the organizational dynamics? And to overcome those kind of think, what is the barrier to evidence seeking and what's the thing I need to do to overcome it?
So is it to kind of give more evidence that this is an issue that companies should be thinking you have to begin with? Is it that I need to help lay a path forward for like if we find x, then we'll do this. If we find y then we'll do that, and to help get by in about what the different paths that you might take are. But I think once you start thinking about those broader organizational issues, it can help to point out, what experiments should you run? And where should you be running them? And how should they be designed?
Gavin Bryant 20:13
Hmm, that's a really good point. One of the ways I think about strategy is really just a hypothesis about what may happen in the future. And we really don't know, the path to achieve that strategy. So where the experiments become really powerful, is that they help you to discover the optimal path to be able to achieve your goals and objectives. So I think flipping the thinking around that strategy is a set and forget that a strategy is its living, its breathing, and it is effectively a hypothesis or theory around what we think may happen, but we're not sure, and we need to validate that to unearth the best way to get there.
Michael Luca 21:04
It's a great point, like I was chatting with the CEO of a large pizza chain, when they were thinking about their strategy. And they were trying to think, where should they be going in their organization? Should they have more takeout they have more sit down to they have healthier items on their menu? Should they make more types of pizza, so you could think about all these choices, and essentially, they're all sort of embedded in that where a series of hypotheses about what their existing customers want, and what potential customers want. But then, by designing experiments, that were either targeted toward existing customers, or potential new customers, they could sort of lay out these hypotheses, and then start to experimentally test them to understand not just their existing products, but to give them a broader framework for understanding what should their path forward look like. And I think this is kind of why it's important for organizations, long lines of what you were just saying, to start thinking about how can this fit into their decision making.
Gavin Bryant 22:05
So let's talk about the Alibaba example, I think this is a great example, one of the things we talked about at the start of the podcast was about asking the right questions up front and setting the scene correctly to begin with. And if we think about those three levels, that we initially start out with a question, a line of investigation, which feeds into our hypotheses, which then connects through into our experiments. Are you able to give listeners a little bit of an overview of the Alibaba example, where potentially asking a different question upfront may have yielded a different outcome?
Michael Luca 22:47
Yeah, so it's a good example of an organization that I think broadly was experimenting, but basically, they had been running these discount programs. So you put something in your shopping cart, Alibaba sees whether the thing is still in your shopping cart or not. And if you leave it sitting there, they could offer you a discount, and see whether you're more likely to buy the item afterwards. So they had run an experiment, where they tested whether discounts effective behavior, and they found that in the short run, you're more likely to buy the thing that was in your shopping cart if offered a discount, but you're not really spending more overall. And the conclusion that they reached was essentially, it's not really worth scaling the program up anymore, and potentially worth kind of ramping it back down. But now, if you're a leader at Alibaba, like you did a nice.... Like, they did a nice job of thinking, kind of use an experiment to understand whether this specific program worked, or what the implication of it was. But there's this whole second purpose of experiments to say, like what might a good program look like? Or how should you design a program? And there you might start thinking about other hypotheses, like, is it the extent of the discount? Is it the salience of the information? Is it the specific people you're targeting? And by more systematically laying out a program of experimentation, you could start to revamp and maybe reach the same conclusion and say, "Look, there's no version of this, that's going to be effective." But actually, maybe you learn something that says it's not the fact that discounts aren't effective. It's the fact that we didn't have them design in the way that was going to keep people engaged on the platform. So I think by sort of shifting from doing this specific thing, work to a broader framework building mindset, that having a program of experiments can be a valuable approach for organizations to take.
Gavin Bryant 24:39
That's a good point rather than thinking of it in binary terms, and to ask more broadly, what could those possibilities be it opens up a number of different opportunities, which may have not been considered or thought about previously. So it's a really good example. So just to shift focus a little bit now your advisory and consulting work is taking you across larger mature organizations, but also into the startup space as well. From your experience how does experimentation differ from the large mature enterprise and between the startup?
Michael Luca 25:20
Yeah, so it's a great question, right? Because it's like the feasibility can be different. The goals of experiments can be different. The outcomes you're able to measure may be different. It's hard to kind of give a one size fits all answer. But I would say in general, like early stage experimentation, you might be also testing earlier in the process, you may not be able to do as many large tests on all of your existing people that you're engaging with, but maybe who go online and do more little pilots to see different directions to go. So I would say you might, as an early stage organization, kind of bring it back to earlier forms of experimentation as well. So kind of like a simple online AB test to sort of test different basic ideas or hypotheses, and then kind of think about how that should affect your design.
Another thing is like, I've seen some startups test their product as a whole. And this has been useful in helping them into engage with other stakeholders. So you sort of design an app and you think the app is going to help people save more money?
So one thing that you could do is you could tell people, here are three reasons why I think the app is going to help people save more money.
Another thing you could do is pay or team up with an organization that's interested in helping to nudge savings, and then roll out the app to some people, but not everybody tracks their savings on the back end, and demonstrate what the impact of your app is. And this gives you a powerful new data point, and a credible way to communicate what the impact of your app was and if the app did help people save money, you would have compelling evidence of that. And if not, then that would give you information that you should revamp your app and figure out how can you go back to the drawing board and find something that will help them achieve the goal that you've set out to do?
In a more mature organization some of the struggles are, how do I now scale experimentation, and it's not always that the larger you get, and the more data you have, the more experiments you want to run.
So kind of give one concrete example that we talked about a little bit in the book is you can think about Uber. So Uber has clearly run lots of experiments. But one lesson that they've learned is as they've grown as, as they've scaled, they've got some really big decisions that they're struggling with, like, do they need new products? Are there new markets, they should think about?
Now, it wasn't just about running more and more A/B tests. But actually, at some point, they pivoted a little bit and said, "Okay, maybe it's not just more experiments, but it's more thoughtful experiments". They actually thought maybe we should run fewer experiments. But for those, we're going to track them longer. We're going to think more carefully about like, what exactly we're trying to learn. And we lay out a nice experiment that they run in the book that had multiple stages, where they were thinking about Uber Express pool, till they had Uber express their Uber pool, and they were trying to think like we have products that allow people to walk together in the car or allow people to wait a little bit more. And they started off with just some calibrations on historical data, then they ran a pilot to see like, does the product that we're trying to build even work? Then they ran like a synthetic control kind of experiment over a few cities. And they were able to get a better sense of what was happening. And then they went even after that back to the drawing board to say, now can we further tweak it? So they sort of built this whole process around one large strategic decision, and then they did this for other decisions too, but then had many parts of each process to make sure they were getting as much as possible out of the series of experiments that they were running for it.
Gavin Bryant 29:03
Yeah, I like that example that you provided in book where Uber elevated it up to market level experimentation, rather than focus on micro AB testing.
I think one of the other important things that you discuss there as well was for the startups to be focusing on experimentation as a tool for directionality rather than answering those question of what should we build? But it's more helping to answer that question. Should we build it and providing maybe trends and indicators of what direction you should head. So yeah, different application, different objectives to a large institutionalize program of experimentation in a large corporate? So, a question I'm really interested to ask you about based on your experience across consulting, advisory and research, what are the key pieces of advice that you would give to organizations who are looking to get started with experimentation
Michael Luca 30:08
Anything you hear about organizations that have a run experiments yet.
Gavin Bryant 30:13
Yes, so an organization that is interested but hasn't experimented yet?
Michael Luca 30:21
Yeah. So here, I would, again kind of returned to thinking about what are the components of experimentation and how my data help. And I would think both about the benefits and the costs of experiments in a particular setting. In particular, you could think about how important is a given decision within the organization. And the more important is, the more valuable more information on it could be? And also think about the fidelity of the experiment to like, how clean can the evidence that you're getting going to be? And the cleaner the evidence, the more useful that's going to be? So you could sort of imagine almost a curve where you're trying to figure out what are the things that give more weight to things that are more important and more weight to things that are more, have cleaner evidence and sort of use that to guide decisions about where to land, and then sort of weigh the cost of the experiment, it gets the benefit?
Now kind of getting a little bit more granular, you get imagine thinking about what do you need for an experiment to take off, you need to have some outcome? It's you need like something you're going to track at the tail end to see are you able to move the needle on that objective? Yeah, you need to be able to randomize people under different conditions. And you need to have a large enough sample right to be able to meaningfully estimate what the impact of the changes.
So I think, there are some kind of core nuts or bolts that leaders need to think about, and sort of guide like, where they should be experimenting. And then once you've decided that you want to experiment, you want to start to think about what the right questions to ask are. Are you thinking about a hypothesis that you have? Is there a specific product that you're trying to test? So those are some of the general things that we've talked about, but it's hard to have, like a one size fits all sort of lesson for leaders who are trying to start experimenting? I wouldn't give a couple examples of one in particular comes to mind, I think one thing I've been impressed by over the years as organizations who didn't have a culture of experimenting, who then started.
So one cool example, I thought is the UK tax department, which we talked about in the book, too. And now one of the things they have been doing is they've been sending out letters to people who owe back taxes, like your please pay your back taxes. And at some point, somebody from the Behavioral Insights Team kind of come in and said, well, like two things...
So,
1) You could use some insights from behavioural economics to increase the likelihood that someone's going to pay their back taxes, but
2) You don't just need to believe that it's going to work, you could actually experimentally test to see what the impact of deliver messages were.
So they started by setting a small number of letters to people, and found that you could greatly increase tax payment rates by changing the language of the letters going out. And once they tried it a few letters, then they started creating a team to sort of say, can we scale up these efforts? And try even more letters, it tried to build some internal frameworks for like, how do you approach tax collection in general? Are there other problems, we might want to think about where we could apply this as well? So I think once you're a leader in an organisation who kind of gets the nuts and bolts of experimentation, it's easy to see, like, 'Oh, here's an area where an experiment is feasible, where it's a pretty important question.' In that case, it was one where the cost of experimenting wasn't too high. And it seems like an easy win to start experimenting.
Gavin Bryant 33:54
Yeah, I like that summary there that experimentation is more utilitarian than purely a digital platform. And there's many, many applications and problems to be solved more broadly throughout a business. And if we think about that example, marketers have been experimenting with direct mail and catalogs since the 1950s, and 60s, so yeah, there's many opportunities that exist outside the realms of our website and app.
So let's finish off now with 3 quick questions to close with.
First question, an experiment that you've performed or observed that reframed organization or perspective, and this might be something that wasn't explored in the book.
Michael Luca 34:49
So it's a good question. There are a few that come to mind. So the one from the book that I'll just return to briefly is this Airbnb experiment, and I wanted to just sort of highlight that as an example where they had already been experimenting, but by pointing out, oh, here's an extra outcome that you really should be thinking about. And here are some tools to help them fight discrimination, got them to reorient their process for experimentation, to build a more robust process that would allow them to continue their growth, while also kind of being more mindful of challenges on the platform. So I'd say that's one example.
Now, during the pandemic, we have experiments of different types to start to try to figure out how can you better help businesses make decisions? So early on, we are interested in actually as a policy question like, how much would giving funding or small grants to small businesses help to increase their likelihood of being able to weather the shutdowns that were happening at the time? And there we ran a series of experiments where we would kind of change the framing of choices and ask questions to businesses about what the likelihood of survival would be given different program availability. And then that gave us useful early information that we could share with policymakers on how to design a program, and what good design might look like, depending on what their policy objectives are.
So I would say, if you step into a policy question, you step into a business decision, there are going to be important questions that experiments and data more generally, could help.
Third example, I'm going to step away from my own research for a second and just say, in general, I think that there's a couple of reasons that leaders really should understand experiments at causal inference.
So
1) Organisations should be thinking about how to run and benefit from their own experiments.
But
2) I'd say, even if you're a leader who thinks that you shouldn't be running your own experiment, there's still great value in trying to understand what you can learn from experiments and here, I would sort of point to the fact that it's very rare for a one size fits all policy change or product change to be right for all businesses in all situations. And once that's not the case, then it can be helpful for a leader to be able to step in and say, well, what's the source of the data? What was the variation? Was it experimental? Was it not? If it's not experimental how much should I trust it, then you could start to dig in a little bit deeper down that part of the path that maybe you trust a lot, and maybe you don't, but it's helpful to be able to think through if it's an experiment, it can be helpful to think about what was the outcome being measured? What was the population being studied? How much does that pour it [phonetic 37:48] over to the setting that I care about? And I'll give one concrete example of this, although I could think of any.
Now you can think about remote work. Every company now is thinking about remote work. So basically lots of companies a bit forced into remote work. And now a lot of companies are interested in like, is remote work helping, is it hurting, should I continue? If I don't continue? What should my return to work policy look like? And there's a lot of guesswork in that. And there's a lot of heterogeneity and what the effect of remote work is for different companies. But one interesting experiment that happened well before the pandemic was run by a company sea [phonetic 38:30] trip, so a travel agency, where they actually said, "Let's experimentally test remote work."
So they ran a lottery that allowed some people to work remotely. And then afterwards, like you, either everyone who opted into a lottery, like either got the lottery and were able to work remotely or not. And they were able to track productivity, job satisfaction, and it gave them some early read on what the impact of remote work was going to be to now when they're forced to make a decision around it, they had some information to go on.
Now, what should leaders make of this;
So
1) Sea trip is probably feeling pretty good that they have at least some evidence internally from this, that gave him a starting point. So you're that's kind of victory that they had that experiment handy to help them make decisions.
But
2) If you're a leader at another company, maybe you should run your own experiment on this. But certainly, you should be able to look at the existing experiments that others have run and sort of think through, like, what does that generalize to my setting, what exactly where they're testing. Are their things I might learn from this. And if you don't have kind of a basic fluency in experiments and data, it's pretty hard to think about how that generalizes to me because you're just going to depend on what somebody else is telling you about it rather than your own sort of understanding of how to interpret that data. So I think kind of getting that skill set could be super valuable for organizations.
Gavin Bryant 39:58
I think that's a really good example that throughout the pandemic, it's thrown up many conditions of uncertainty and ambiguity where it's not possible to know all the answers. And a way that you can potentially find some of the answers to these very difficult questions or trying to answer is to run experiments. And those experiments may be customer facing, but to your final example, that they may also be internally facing, which impact ways of working employee engagement and other parameters inside the business.
So quick question number-2. So thinking about three resources that you've found helpful in helping you to become more effective experimentation outside of the power of experiments book, what would those three resources be?
Michael Luca 40:54
Let me start with just like three types of resource. I think there's lots of resources that are super useful.
I would say one type of resource that is useful is research judgment or decision making. So there's this behavioral economics literature on what are the mistakes we make when we're looking at data or thinking about data? I think that could be helpful for people who just want to get calibrated on, where am I potentially making mistakes in my own decisions and how my more systematic looks at data help?
There, you might look at knowledge, you might look at some of the Danny Kahneman work, you might have worked, look at some of the work by the Behavioral Insights Team in the UK. So that's sort of one bucket of things.
The second bucket, I would say, is just like kind of how do I run an experiment? So what are the nuts and bolts? Like what are the tools? And there I think there's a series of good resources on how do you design and experiment? And what should the experiment look like you're so Gerber Green, if you're looking for a textbook style treatment, I think mostly harmless econometrics has some useful principles. So there are a number of good books, textbooks and general books that sort of give treatments for some of the technical aspects of it.
And the third, I would say, is almost the managerial kind of what are the best practices around experimentation, and then I also think there's a number of good resources that are starting to come on. Now, Ronnie Kohavi has nice book. You know, I'm going to misquote the name of it, but kind of something like a manager's Guide to Online experimentation. So if you looked up Ronnie Kohavi, you'll see the book. And I think it has a very practical book as somebody who's in an organization who wants to implement an online experiment that just helps the lady walk through some of the decisions involved, trying to think if there's other ones along that line. Stephen Tomke, has a new book on experiments. But when I think about it, rather than any one resource, it would really be thinking about, what are these buckets of things that will help to shift my paradigm when thinking about experiments?
Gavin Bryant 43:08
Good point. Experimentation is multi-dimensional. So you think about the buckets rather than any one, two or three individual resources.
Final question; Where can listeners reach out to you if they'd like to get in contact?
Michael Luca 43:24
So I keep all my research on my website. So that's one area for people to just want to read more I'm on LinkedIn, and I'm on Twitter. So if people wanted to find me at either of those.
Gavin Bryant 43:39
Excellent. Thank you so much for your time today, Michael. We really appreciate it.
Michael Luca 43:44
Great, thanks. It was great to chat.
Highlights
Businesses are not only increasingly becoming more interested in using the findings of experiments to be more effective, but the experimentation process as a better way to solve complex business problems
Experiments start with asking the right question. The research question you ask upfront >> connects to your hypotheses >> which connects to experiment design
If you’re a leader, or decision maker, it’s important to realise that having data and experiments is not enough. You need to be able to link experiments back to managerial decision-making
Over the past 50 years we’ve seen a growing body of evidence that indicates that we don’t know in advance what will work. Our intuition is flawed. Experiments are one way to check our intuition, making sure that we’re removing biases from our decisions where possible
Experimentation has become so prevalent in the tech sector because there’s no longer a need to guess - tech companies have large numbers of users, they’re constantly measuring outcomes, it’s easy to randomise users, the cost of data is constantly dropping and the technical ease of performing experiments is decreasing. Why would you guess, when you can test?
As a leader, if you’re not thinking about experiments, you’re introducing blind spots into your decision-making process
Experimentation hasn’t been in business lexicon for very long. We can often forget how difficult it can be to elicit large scale cultural transformation to enable experimentation
eBay has saved more than $50M per annum by stopping paid advertising. A team of eBay economists ran large-scale experiments in different markets, turning paid ads on and off. The team discovered that paid advertising was a waste as users still came to eBay through organic search
As a leader you need to be willing to engage in discussions of data and evidence. You need to be constantly thinking about organisational objectives, and when you’re performing experiments, are the outcomes you’re measuring the outcomes that you care about
You can’t just reward victories. In your organisation you have to define what it is to be successful. Trying and failing, trying and failing early, and then moving on to the right thing, should be valued and rewarded, not punished
Be careful that you’re not optimising on a goal that is too narrow. Focussing on short run conversions can often lead to unintended consequences. You also need to be aware of the Second Order and Third Order effects. Airbnb was so focussed on short run corporate growth that it neglected the platform contributing to racial discrimination
Experiment velocity is not the ultimate end-game. Don’t get hung up trying to perform more and more experiments, when you should be zooming out and thinking about how you can perform more thoughtful experiments. Often, performing fewer experiments, with a clearer focus on learning outcomes, is a better strategy
In this episode we discuss:
How Michael developed his passion for experimentation
Michael’s principles for experimentation
Why experimentation is a critical part of the leadership toolkit
How eBay saved $50M per annum by stopping paid advertising
Why leaders need to be willing to have evidence based discussions
How Airbnb overlooked second and third effects of experimentation
Why leaders avoid experimentation
How Uber changed their strategy for experimentation
Advice for businesses getting started with experimentation
Success starts now.
Beat The Odds newsletter is jam packed with 100% practical lessons, strategies, and tips from world-leading experts in Experimentation, Innovation and Product Design.
So, join the hundreds of people who Beat The Odds every month with our help.